Metapath-fused heterogeneous graph network for molecular property prediction.
Inf. Sci.(2023)
摘要
Molecular property prediction can guide molecular design and optimization in drug discovery. As molecules are inherently graph-structured data, graph learning has significantly boosted molecular property prediction tasks. However, many existing graph-based methods are designed for low-order node interactions in homogeneous graphs, ignoring different types of atomic nodes or edges. In this paper, we propose to model a molecule as a heterogeneous graph and leverage metapaths to capture latent features for chemical functional groups. To preserve the chemical environments revealed by metapaths, we construct metapath-based connectivity and decompose the heterogeneous graph into subgraphs according to relation types. In addition, a hierarchical attention strategy is designed to aggregate heterogeneous information at the node level and relation level. Consequently, our approach is more natural and suitable for learning the complex interactions among atomic nodes and effective molecular fingerprints. We evaluate our model on public molecular datasets, and the experimental results exhibit the effectiveness of our model with competitive performance against the existing approaches.
更多查看译文
关键词
Drug discovery,Graph neural network,Heterogeneous information network,Graph mining
AI 理解论文
溯源树
样例
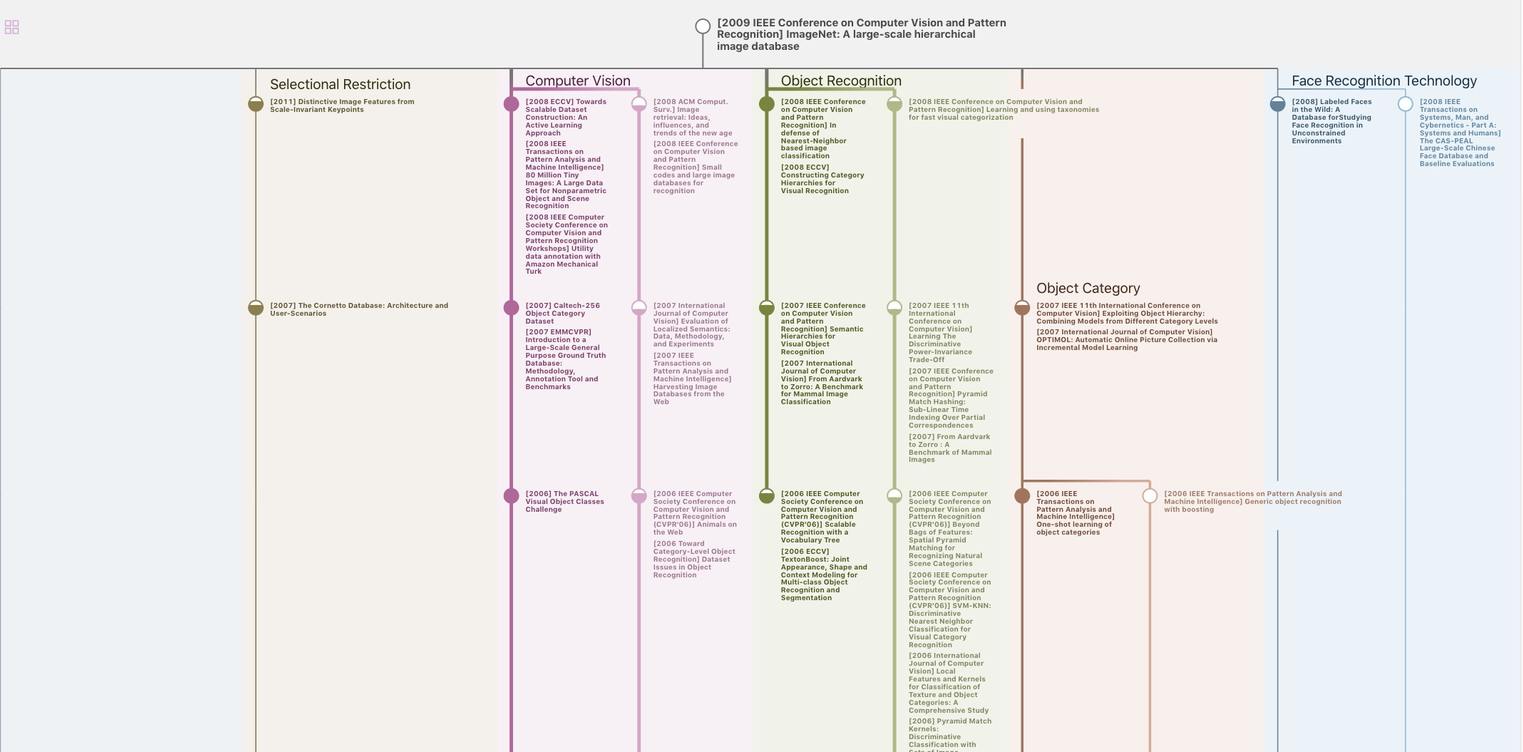
生成溯源树,研究论文发展脉络
Chat Paper
正在生成论文摘要