Multi-scale integrated deep self-attention network for predicting remaining useful life of aero-engine
Eng. Appl. Artif. Intell.(2023)
摘要
Remaining useful life (RUL) prediction is the core research task of aero-engine prognostics health management (PHM), which is crucial to promoting the safety, reliability and economy. Therefore, in this paper, a multi-scale integrated deep self-attention network (MSIDSN) is proposed to process aero-engine multisensory data containing degradation information at different scales and then accurately predict the corresponding RUL of the aero-engine. Firstly, multi-scale blocks with self-attention strategy are constructed to selectively extract multisensory features on different scales. Secondly, an enhanced recurrent neural network module is designed to comprehensively extract the degraded features on multiple temporal scales. Finally, the feature fusion layer fuses the features and outputs the predicted RUL. Furthermore, an efficient loss function is developed to correct for the delay prediction, and avoid accidents. The experimental comparison results with other models verify the superiority of MSIDSN.
更多查看译文
关键词
Remaining useful life prediction,Aero-engine multisensory data,Multi-scale block,Enhanced recurrent neural network module
AI 理解论文
溯源树
样例
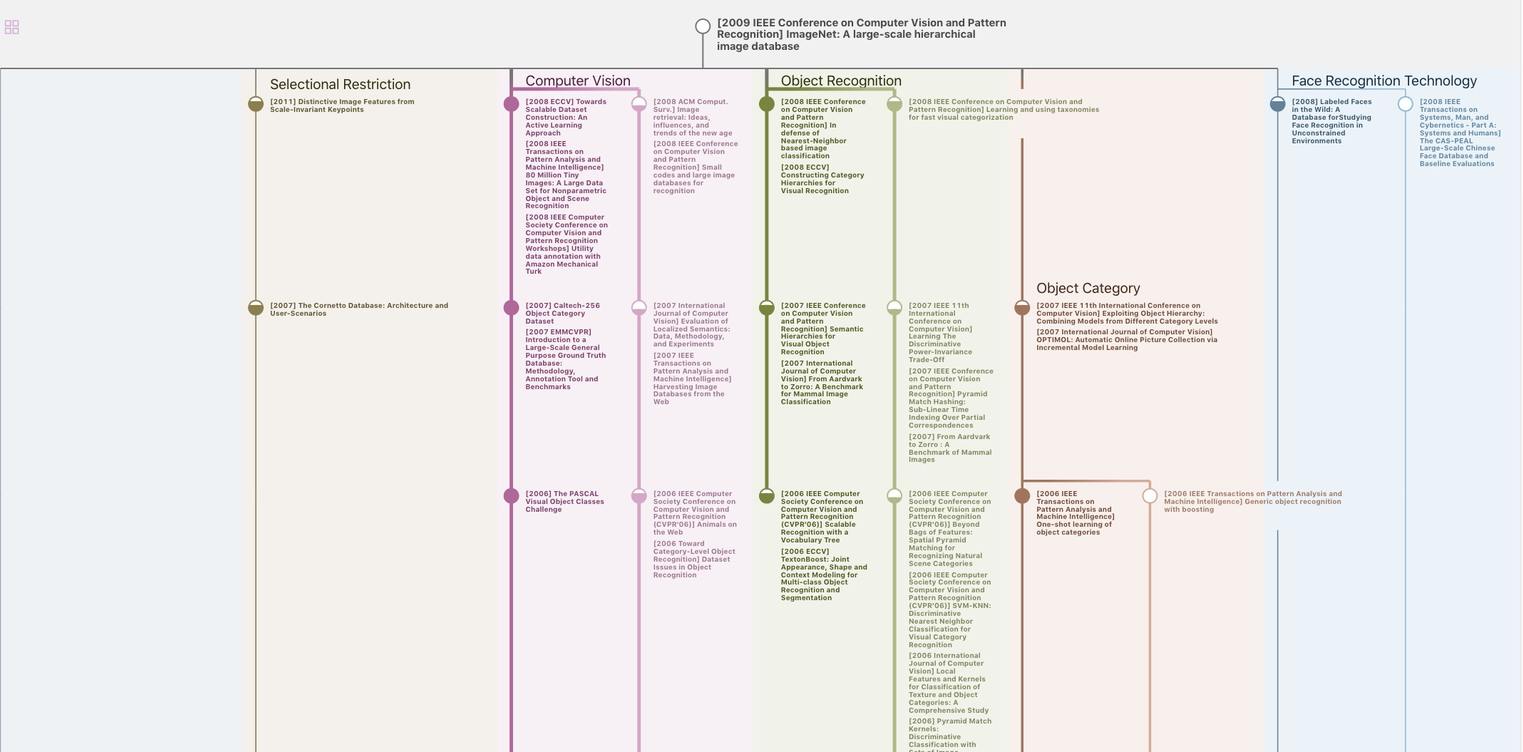
生成溯源树,研究论文发展脉络
Chat Paper
正在生成论文摘要