An accurate automated speaker counting architecture based on James Webb Pattern.
Eng. Appl. Artif. Intell.(2023)
摘要
Speaker counting is an important research area in sound forensics. There are limited speaker counting papers in the literature, as it is challenging to collect datasets. This work aims to collect a new overlapping speech signal dataset for speaker counting and propose a novel feature engineering model. In this work, textural feature extraction is based on the iconic James Webb space telescope; hence, this pattern is named James Webb Pattern (JWPat). A new speaker counting speech dataset comprising 3,121 speeches divided into 32 classes (the class number corresponded to the number of speakers) was collected. A new framework that mimics the deep learning model has been proposed to classify the collected speech classes. The proposed feature engineering model is self-organized and uses various mother wavelet functions to generate features at both low and high levels. We have obtained the best classification accuracy of 86.74% using the symlet4 mother wavelet function. Using our proposed framework, eight classification results have been calculated with accuracy ranging from 75.94% to 86.74%. This range is over 10% accuracy, and it demonstrates the effect of the mother wavelet function on the classification performance. Moreover, the feature extraction capability of the mirror of the James Webb telescope has been demonstrated. Our proposed method yielded 86.74% accuracy on a large dataset and indicated the success of our proposed model.
更多查看译文
关键词
James Webb pattern,Unbalanced tree discrete wavelet transform,Speaker counting,Iterative neighborhood component analysis,Sound forensics
AI 理解论文
溯源树
样例
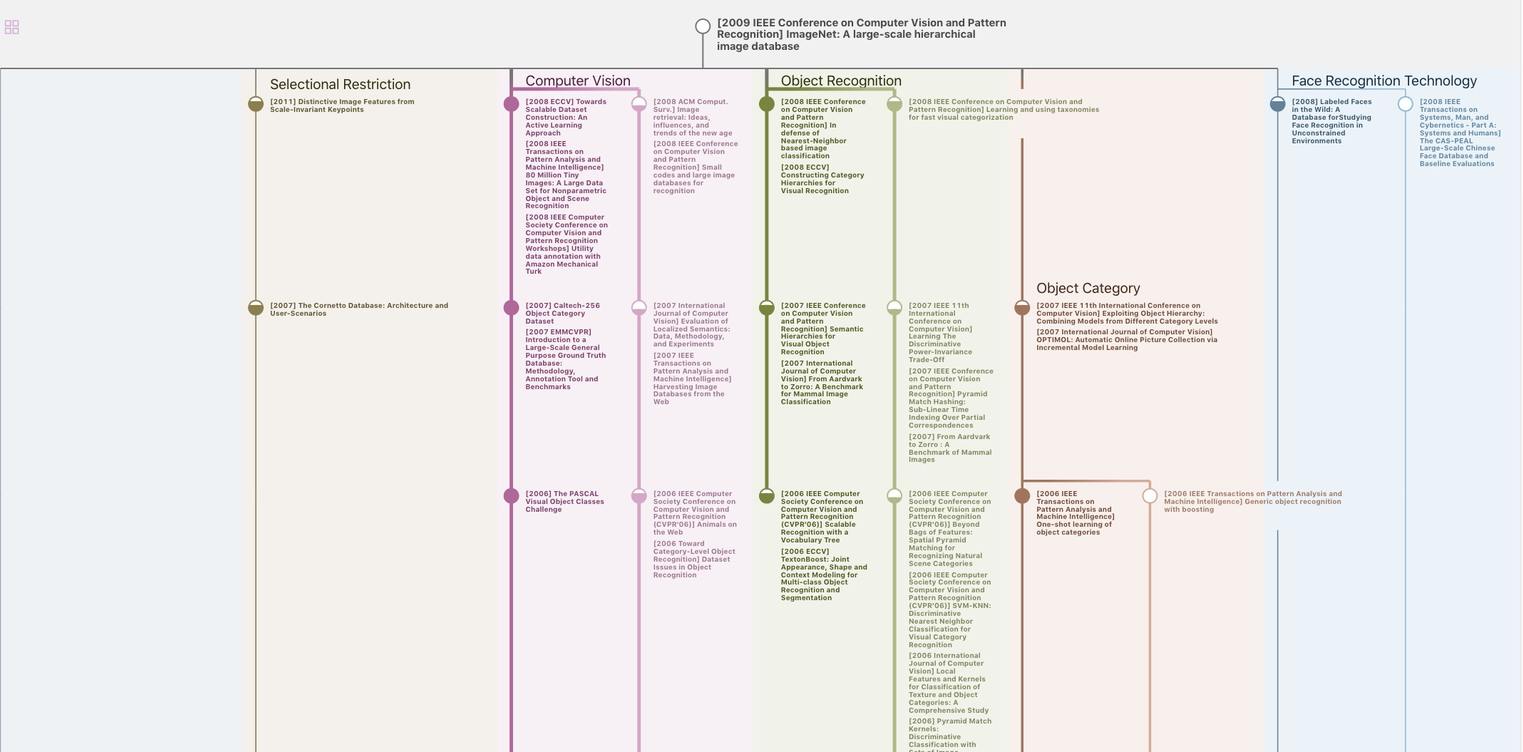
生成溯源树,研究论文发展脉络
Chat Paper
正在生成论文摘要