Selecting slacks-based data envelopment analysis models.
Eur. J. Oper. Res.(2023)
摘要
Data envelopment analysis (DEA) is a well-known data-driven mathematical modeling approach that aims at evaluating the relative efficiency of a set of comparable decision making units (DMUs) with multiple inputs and multiple outputs. The number of inputs and outputs (performance factors) plays a vital role for successful applications of DEA. There is a statistical and empirical rule in DEA that if the number of performance factors is high in comparison with the number of DMUs, then a large percentage of the units will be determined as efficient, which is questionable and unacceptable in the performance evaluation context. However, in some real-world applications, the number of performance factors is relatively larger than the number of DMUs. To cope with this issue, selecting models have been developed to select a subset of performance factors that lead to acceptable results. In this paper, we extend a pair of optimistic and pessimistic approaches, involving two alternative individual and summative selecting models, based on the slacks-based model. We mathematically validate the proposed models with some theorems and lemmas and illustrate the applicability of our models using 18 active auto part companies in the largest stock exchange in Iran. (c) 2022 Elsevier B.V. All rights reserved.
更多查看译文
关键词
Data envelopment analysis,slacks -based measure (SBM),Selecting models,Optimistic and pessimistic approaches,performance factors
AI 理解论文
溯源树
样例
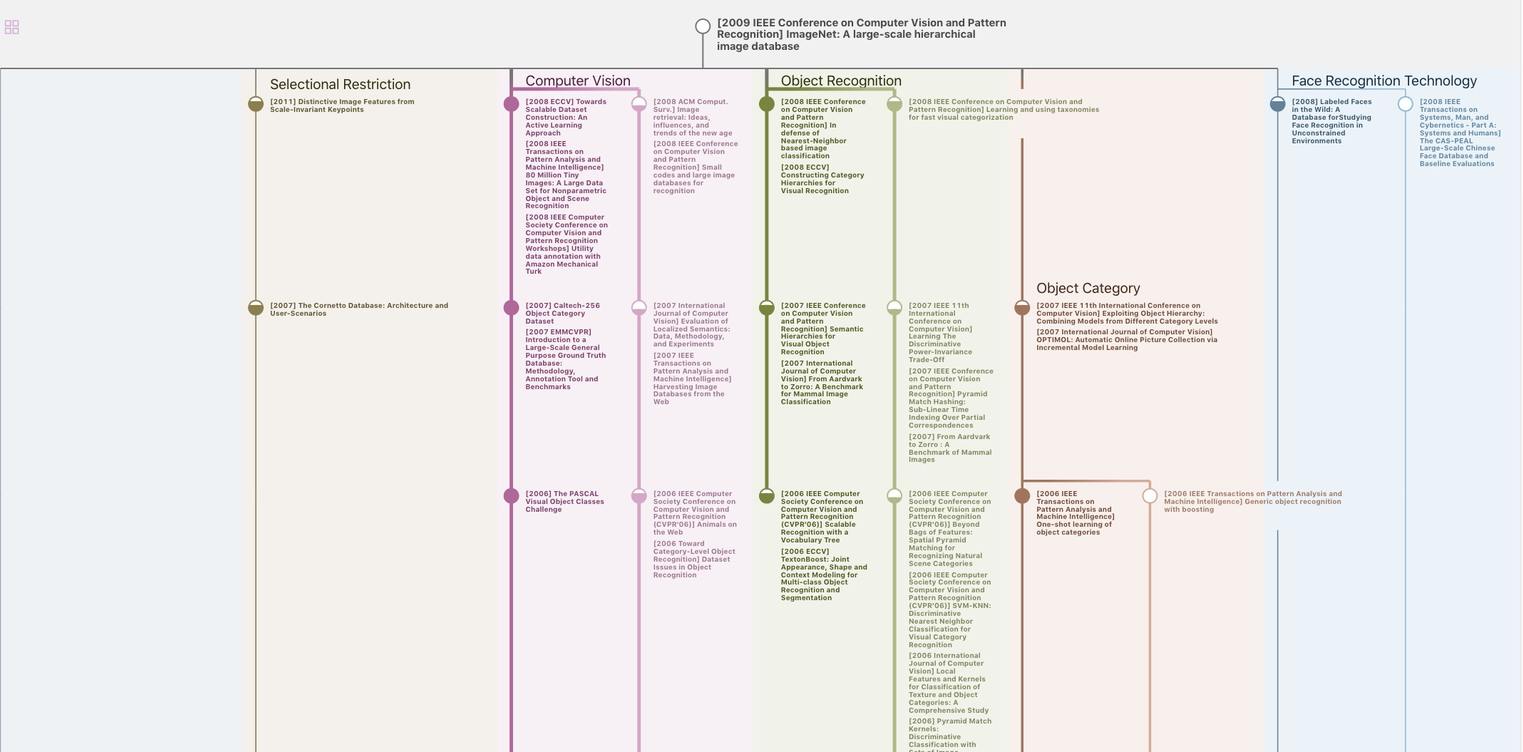
生成溯源树,研究论文发展脉络
Chat Paper
正在生成论文摘要