Charge Own Job: Saliency Map and Visual Word Encoder for Image-Level Semantic Segmentation.
ECML/PKDD (3)(2022)
摘要
Significant advances in weakly-supervised semantic segmentation (WSSS) methods with image-level labels have been made, but they have several key limitations: incomplete object regions, object boundary mismatch, and co-occurring pixels from non-target objects. To address these issues, we propose a novel joint learning framework, namely Saliency Map and Visual Word Encoder (SMVWE), which employs two weak supervisions to generate the high-quality pseudo labels. Specifically, we develop a visual word encoder to encode the localization map into semantic words with a learnable codebook, making the network generate localization maps containing more semantic regions with the encoded fine-grained semantic words. Moreover, to obtain accurate object boundaries and eliminate co-occurring pixels, we design a saliency map selection mechanism with the pseudo-pixel feedback to separate the foreground from the background. During joint learning, we fully utilize the cooperation relationship between semantic word labels and saliency maps to generate high-quality pseudo-labels, thus remarkably improving the segmentation accuracy. Extensive experiments demonstrate that our proposed method better tackles above key challenges of WSSS and obtains the state-of-the-art performance on the PASCAL VOC 2012 segmentation benchmark.
更多查看译文
关键词
saliency map,visual word encoder,image-level
AI 理解论文
溯源树
样例
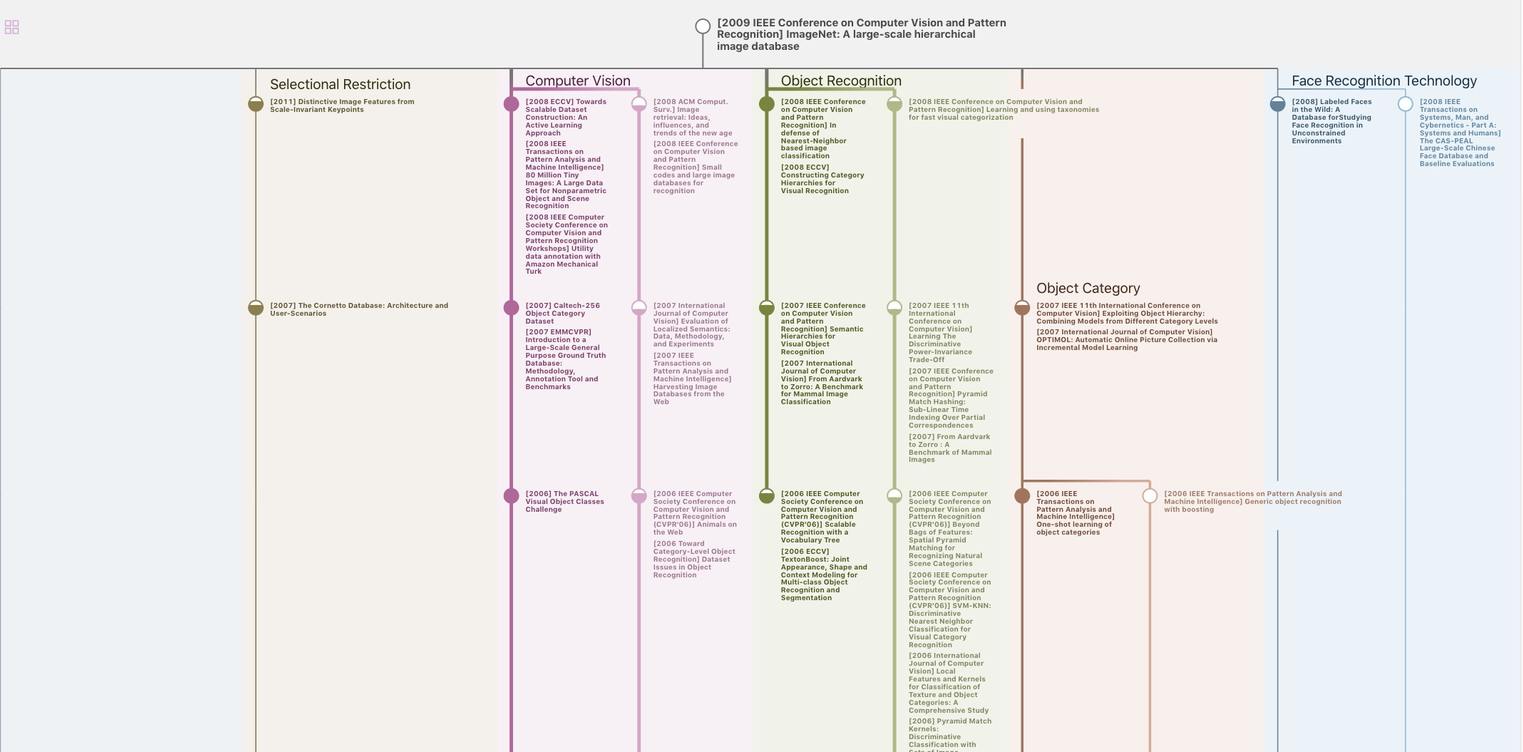
生成溯源树,研究论文发展脉络
Chat Paper
正在生成论文摘要