RPConvformer: A novel Transformer-based deep neural networks for traffic flow prediction.
Expert Syst. Appl.(2023)
摘要
Traffic prediction problem is one of the essential tasks of intelligent transportation system (ITS), alleviating traffic congestion effectively and promoting the intelligent development of urban traffic. To accommodate long-range dependencies, Transformer-based methods have been used in traffic prediction tasks due to the parallelizable processing of sequences and explanation of attention matrices compared with recurrent neural units (RNNs). However, the Transformer-based model has two limitations, on the one hand, it ignores the local correlation in the traffic state in its parallel processing of the sequence, on the other hand, the absolute positional embedding is adopted to represent the positional relationship of time nodes is destroyed when it comes to calculate attention score. To address two embarrassing shortcomings, a novel framework called RPConvformer is proposed, where the improved parts are 1D causal convolutional sequence embedding and relative position encoding. In sequence embedding, we develop a sequence embedding layer composed of convolutional units, which consist of origin 1D convolutional and 1D causal convolutional. The size of the receptive field of the convolution can focus on the local region correlation of the sequence. In relative position encoding, we introduce a bias vector to automatically learn the relative position information of time nodes when linearly mapping the feature tensor. We respect the encoding and decoding framework of the Transformer, the encoder is responsible for extracting historical traffic state information, and the decoder autoregressively predicts the future traffic state. The multi-head attention mechanism is adopted by both encoder and decoder aims to focus on rich temporal feature patterns. Moreover, key mask technique is used after computing attention matrix to mask the traffic state at missing moments improving the resilience of the model. Extensive experiments on two real-world traffic flow datasets. The results show that RPConvformer achieves the best performance compared to state-of-the-art time series models. Ablation experiments show that considering the local correlation of time series has a higher gain on prediction performance. Random mask experiments show that the model is robust when the historical data is less than 10% missing. In addition, multi-head attention matrix provides further explanation for the dependence between time nodes. RPConvformer as an improved Transformer-based model can provide new ideas for molding temporal dimension in traffic prediction tasks. Our code has been open-sourced at (https://github.com/YanJieWen/RPConvformer).
更多查看译文
关键词
Intelligent transportation system,Traffic prediction,Transformer,Positional embedding,1D causal convolutional,Multi-head attention
AI 理解论文
溯源树
样例
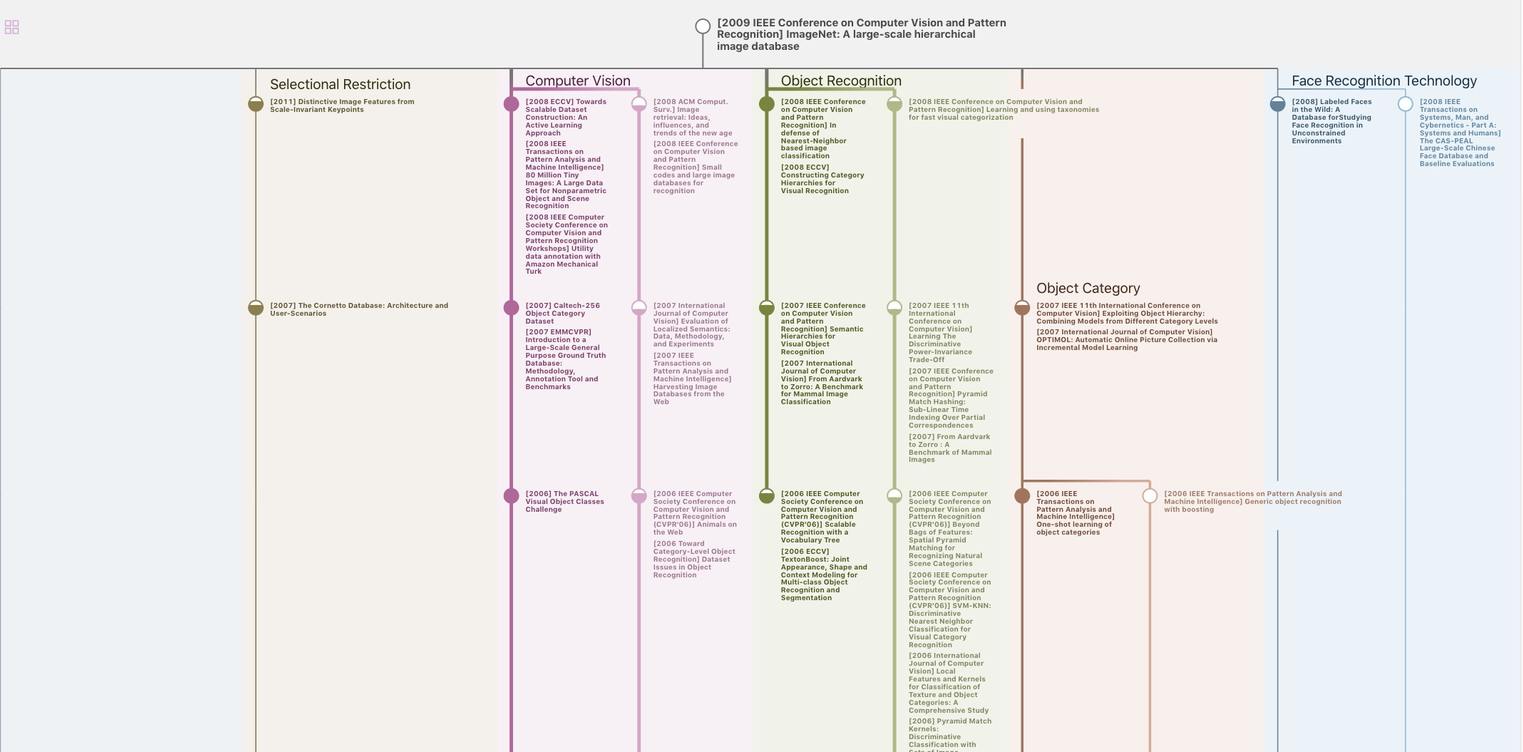
生成溯源树,研究论文发展脉络
Chat Paper
正在生成论文摘要