Spatial-Temporal Adaptive Graph Convolutional Network for Skeleton-Based Action Recognition.
ACCV (4)(2022)
摘要
Skeleton-based action recognition approaches usually construct the skeleton sequence as spatial-temporal graphs and perform graph convolution on these graphs to extract discriminative features. However, due to the fixed topology shared among different poses and the lack of direct long-range temporal dependencies, it is not trivial to learn the robust spatial-temporal feature. Therefore, we present a spatial-temporal adaptive graph convolutional network (STA-GCN) to learn adaptive spatial and temporal topologies and effectively aggregate features for skeleton-based action recognition. The proposed network is composed of spatial adaptive graph convolution (SA-GC) and temporal adaptive graph convolution (TA-GC) with an adaptive topology encoder. The SA-GC can extract the spatial feature for each pose with the spatial adaptive topology, while the TA-GC can learn the temporal feature by modeling the direct long-range temporal dependencies adaptively. On three large-scale skeleton action recognition datasets: NTU RGB+D 60, NTU RGB+D 120, and Kinetics Skeleton, the STA-GCN outperforms the existing state-of-the-art methods. The code is available at
https://github.com/hang-rui/STA-GCN
.
更多查看译文
关键词
action,graph,recognition,spatial-temporal,skeleton-based
AI 理解论文
溯源树
样例
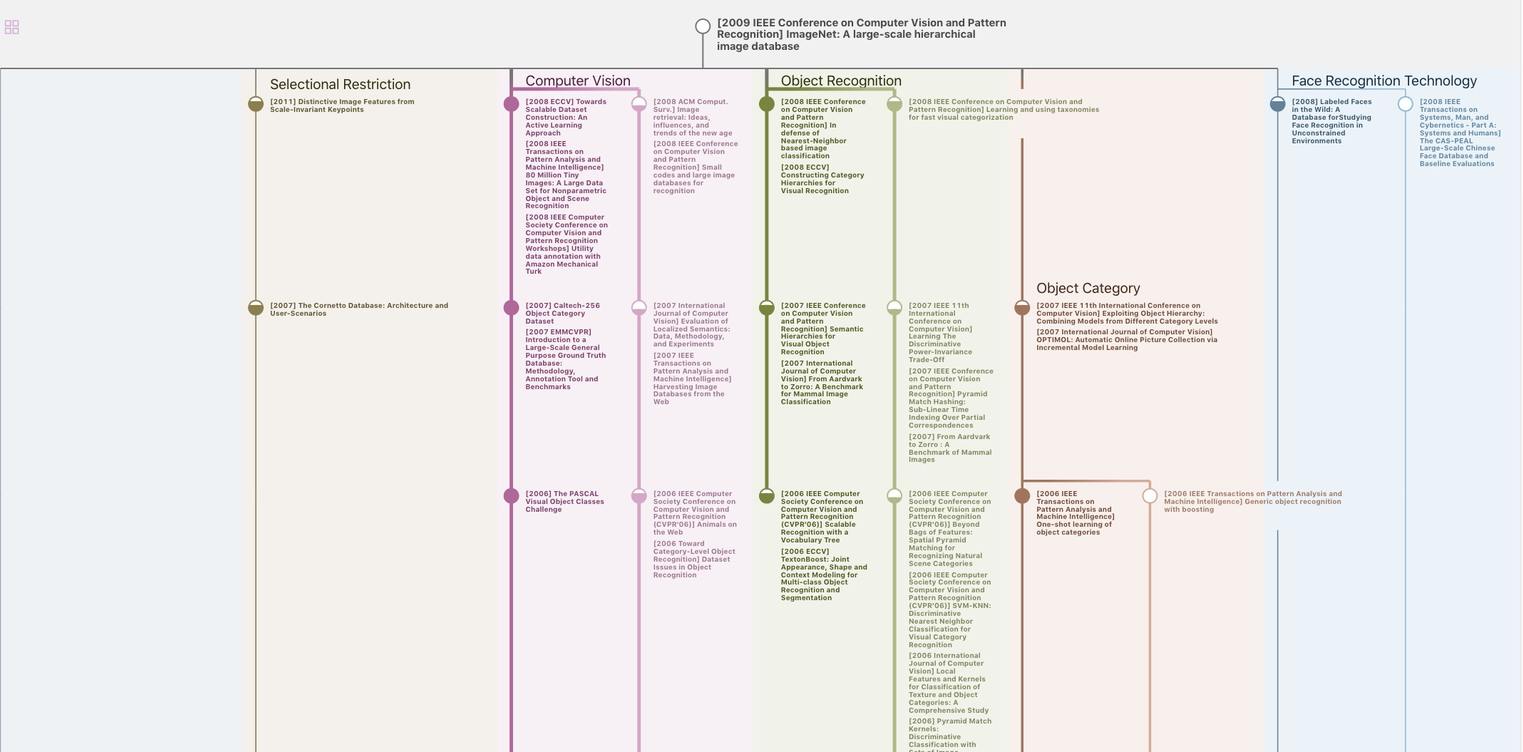
生成溯源树,研究论文发展脉络
Chat Paper
正在生成论文摘要