SCGraph: Accelerating Sample-based GNN Training by Staged Caching of Features on GPUs.
ISPA/BDCloud/SocialCom/SustainCom(2022)
摘要
Graph neural networks (GNNs) have been becoming important tools for processing structured graph data and successfully applied to multiple graph-based application scenarios. The existing GNN systems adopt sample-based training on large-scale graphs over multiple GPUs. Although they support large-scale graph training, large data loading overhead of transferring vertex features between CPUs and GPUs is still a bottleneck. In this work, we propose SCGraph, a method that supports GPU high-speed feature caching. SCGraph classifies the graph vertices sorted by out-degrees. For high out-degree vertices, SCGraph sets grading caches via different GPUs to increase the overall cache content through NVLink high-speed data transmission between them. For low out-degree vertices, SCGraph expands training vertices' neighborhood in advance to regenerate cache. We evaluate SCGraph against two state-of-the-art industrial GNN frameworks, i.e., DGL and PaGraph on various benchmarks. Experimental results show that SCGraph improves the cache hit rate over GPUs up to 23.6%, and achieves up to 1.71x performance speedup over the state-of-the-art baselines while the convergence almost constant.
更多查看译文
关键词
graph neural networks,feature caching,sampling
AI 理解论文
溯源树
样例
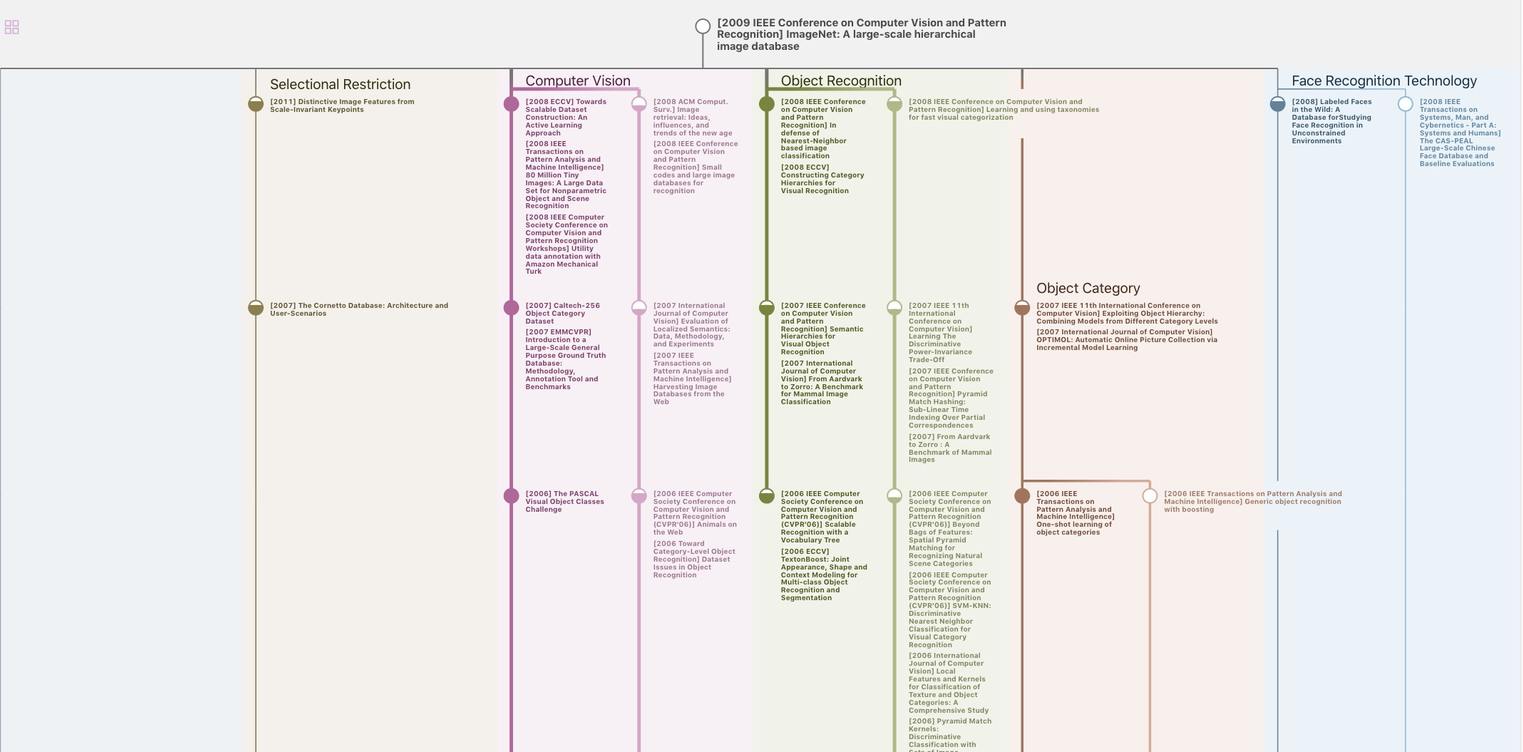
生成溯源树,研究论文发展脉络
Chat Paper
正在生成论文摘要