DLVR-NWP: A Novel Data-Driven Bearing Degradation Model for RUL Estimation.
IEEE Trans. Instrum. Meas.(2023)
摘要
Performance degradation process modeling and remaining useful life (RUL) estimation have gained much attention for condition-based maintenance of important engineering equipment such as the bearings of gantry cranes. The existing work mainly uses the original or elaborately selected features that may not necessarily correspond to the current degradation process. In view of this, this article proposes a novel data-driven bearing degradation modeling method, called dynamic latent variable reconstruction nonlinear Wiener process (DLVR-NWP). The proposed DLVR-NWP method is composed of a feature generation, a dynamic latent variable (DLV)-based nonlinear degradation detection, a DLV reconstruction (DLVR)-based degradation-relevant dynamic feature extraction, and a failure magnitude-based nonlinear Wiener process (NWP) model for RUL estimation. The reduced-dimensional degradation-relevant dynamic feature is first extracted from multiple highly-correlated time-domain and frequency-domain features using a DLV reconstruction method that finds out the magnitude feature along the historical similar degradation processes. By incorporating the DLV-based nonlinear degradation detection mechanism, degradation-relevant dynamic features during the degradation stage instead of the overall operation duration are integrated into the NWP model for RUL estimation. Results from case studies on real bearings indicate that the proposed method using degradation-relevant dynamic feature significantly improves the RUL estimation accuracy compared to the traditional methods.
更多查看译文
关键词
degradation model,bearing,dlvr-nwp,data-driven
AI 理解论文
溯源树
样例
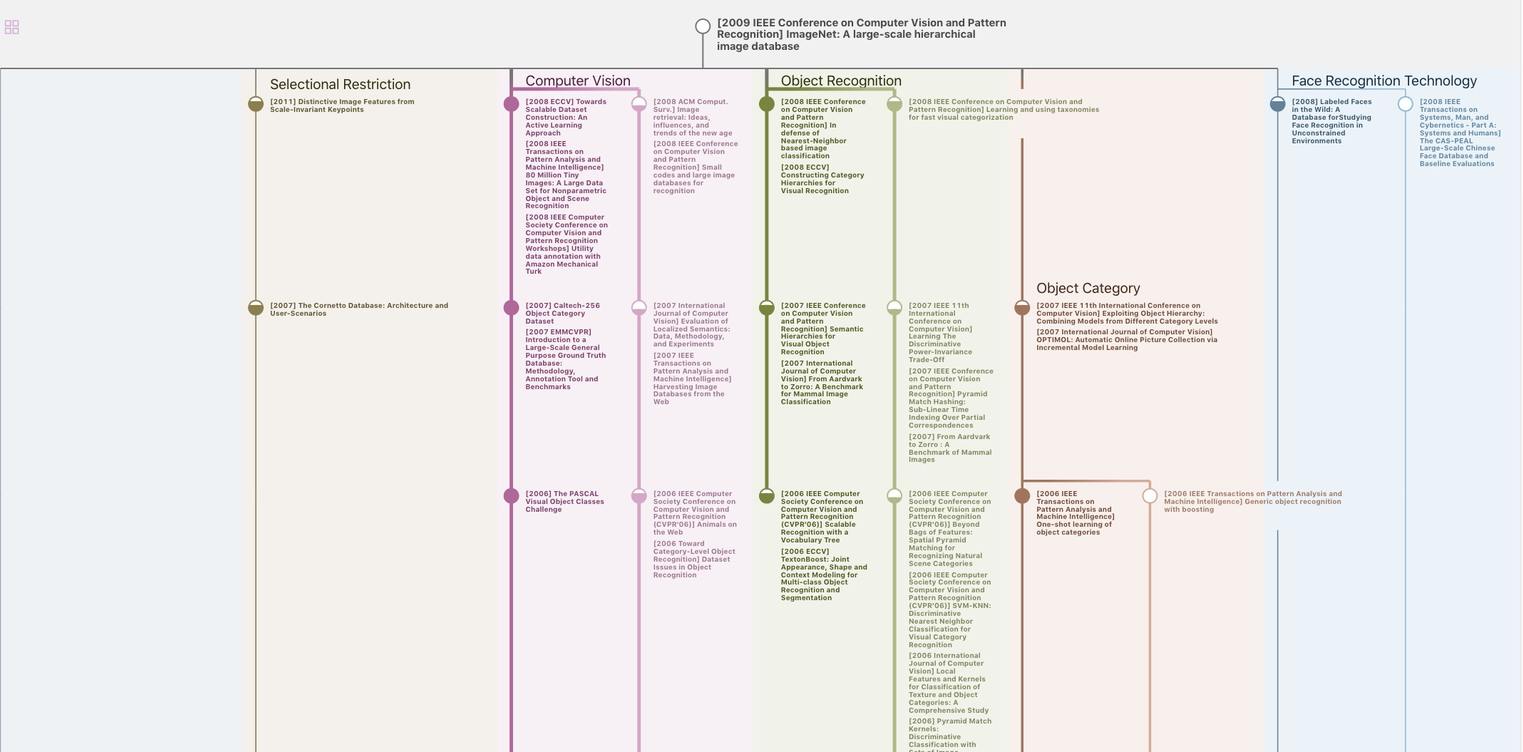
生成溯源树,研究论文发展脉络
Chat Paper
正在生成论文摘要