Towards federated learning: An overview of methods and applications.
WIREs Data. Mining. Knowl. Discov.(2023)
摘要
Federated learning (FL) is a collaborative, decentralized privacy-preserving method to attach the challenges of storing data and data privacy. Artificial intelligence, machine learning, smart devices, and deep learning have strongly marked the last years. Two challenges arose in data science as a result. First, the regulation protected the data by creating the General Data Protection Regulation, in which organizations are not allowed to keep or transfer data without the owner's authorization. Another challenge is the large volume of data generated in the era of big data, and keeping that data in one only server becomes increasingly tricky. Therefore, the data is allocated into different locations or generated by devices, creating the need to build models or perform calculations without transferring data to a single location. The new term FL emerged as a sub-area of machine learning that aims to solve the challenge of making distributed models with privacy considerations. This survey starts by describing relevant concepts, definitions, and methods, followed by an in-depth investigation of federated model evaluation. Finally, we discuss three promising applications for further research: anomaly detection, distributed data streams, and graph representation.This article is categorized under:Technologies > Machine LearningTechnologies > Artificial Intelligence
更多查看译文
关键词
artificial intelligence,federated frameworks,federated learning,machine learning,privacy
AI 理解论文
溯源树
样例
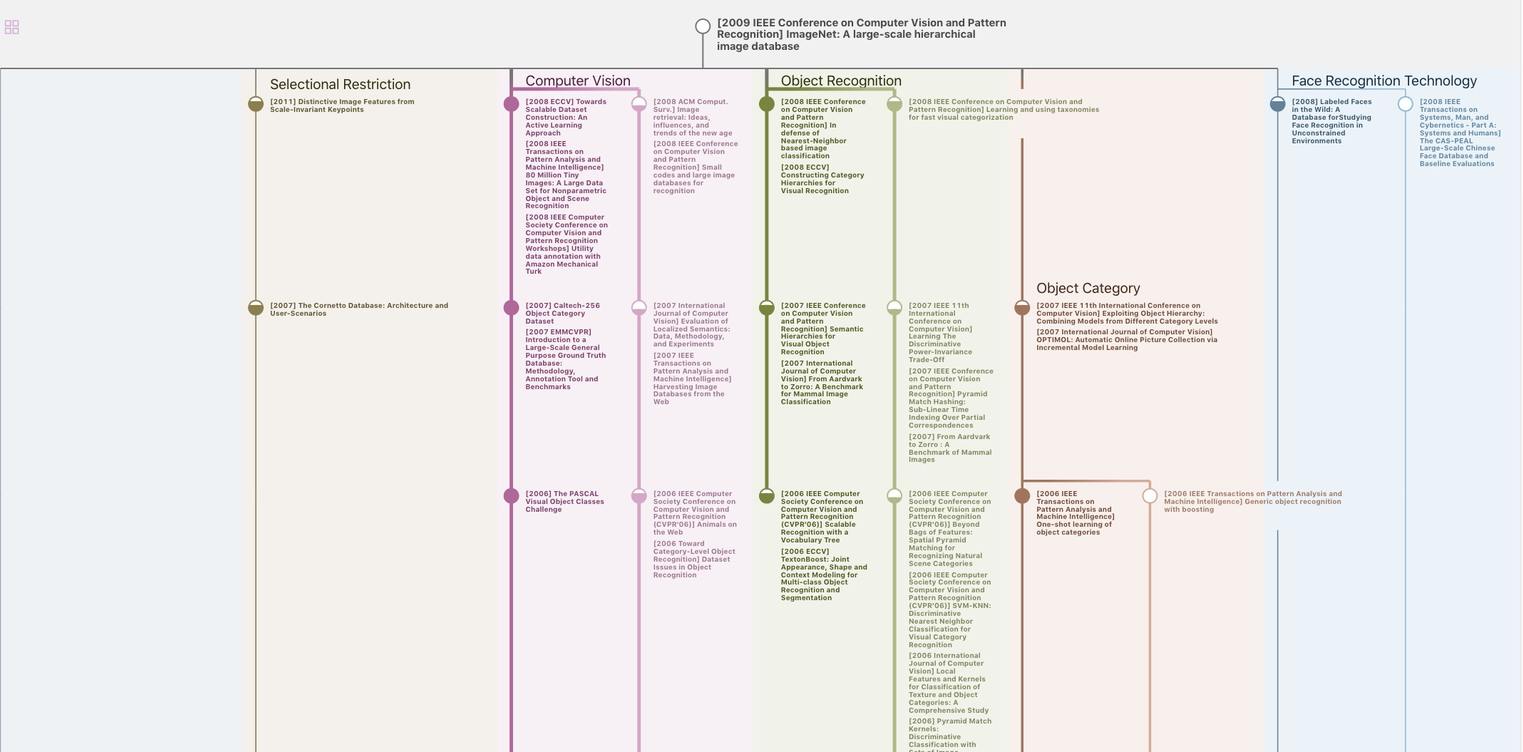
生成溯源树,研究论文发展脉络
Chat Paper
正在生成论文摘要