Low-Rank and Sparse Metamorphic Autoencoders for Unsupervised Pathology Disentanglement.
MAD@MICCAI(2022)
摘要
In order to establish population-based analysis of image data from multi-center studies, it is often helpful to disentangle images in their shape and appearance components. However, abnormal (e.g. pathological) and normal appearances of images strongly differ and should ideally be separated in the modeling process. In this work, we propose a metamorphic autoencoder for the disentanglement of shape as well as normal and abnormal appearance of medical images by integrating a low-rank and sparse decomposition into the training process. Experiments show that this method can reliably be used for unsupervised pathology disentanglement opening perspectives for unsupervised pathology segmentation, pseudo-healthy image synthesis and conditional image generation.
更多查看译文
关键词
sparse metamorphic autoencoders,pathology,low-rank
AI 理解论文
溯源树
样例
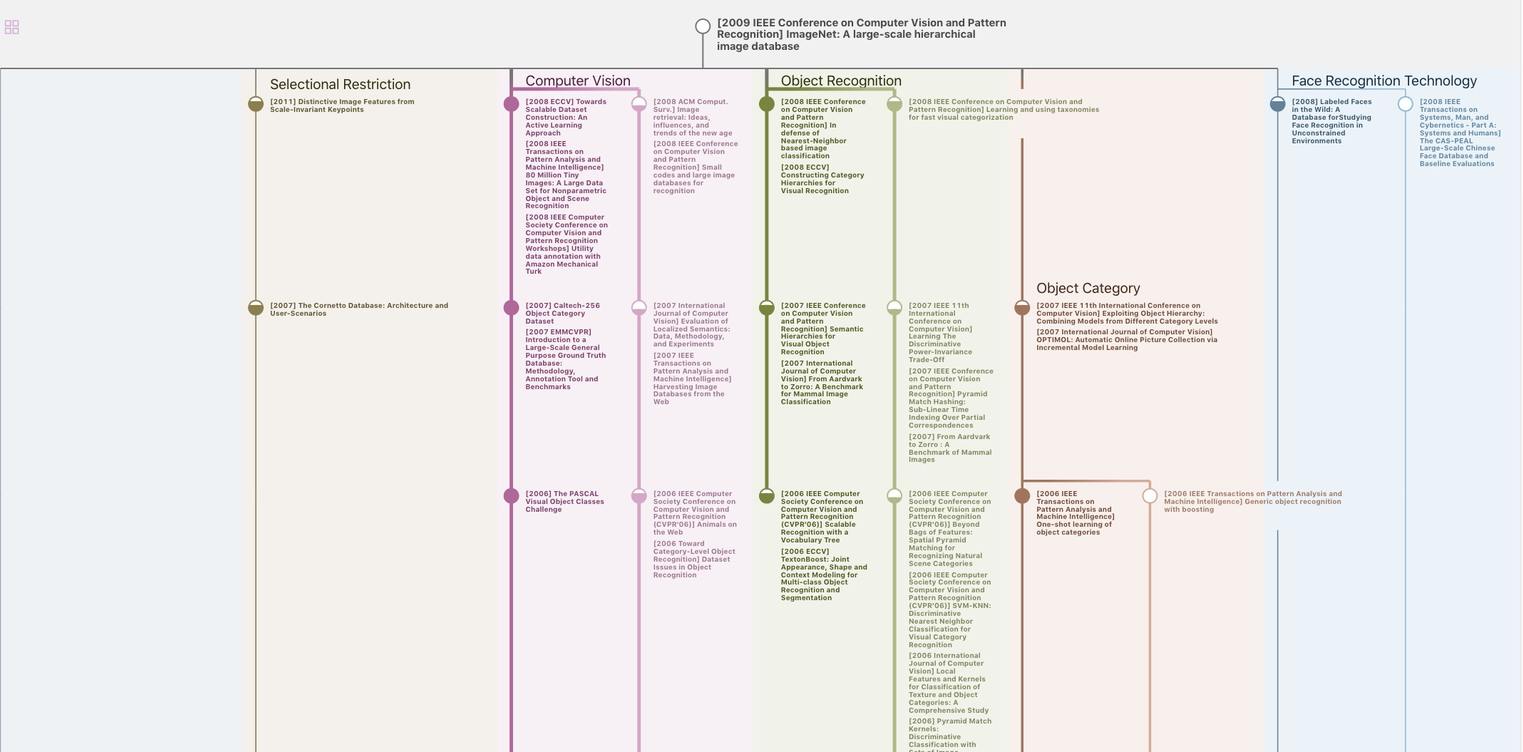
生成溯源树,研究论文发展脉络
Chat Paper
正在生成论文摘要