Distributionally Robust Optimization for Input Model Uncertainty in Simulation-Based Decision Making
WSC(2022)
摘要
We consider a new approach to solve distributionally robust optimization formulations that address nonparametric input model uncertainty in simulation-based decision making problems. Our approach for the minimax formulations applies stochastic gradient descent to the outer minimization problem and efficiently estimates the gradient of the inner maximization problem through multi-level Monte Carlo randomization. Leveraging theoretical results that shed light on why standard gradient estimators fail, we establish the optimal parameterization of the gradient estimators of our approach that balances a fundamental tradeoff between computation time and statistical variance. We apply our approach to nonconvex portfolio choice modeling under cumulative prospect theory, where numerical experiments demonstrate the significant benefits of this approach over previous related work.
更多查看译文
关键词
input model uncertainty,optimization,decision making,simulation-based
AI 理解论文
溯源树
样例
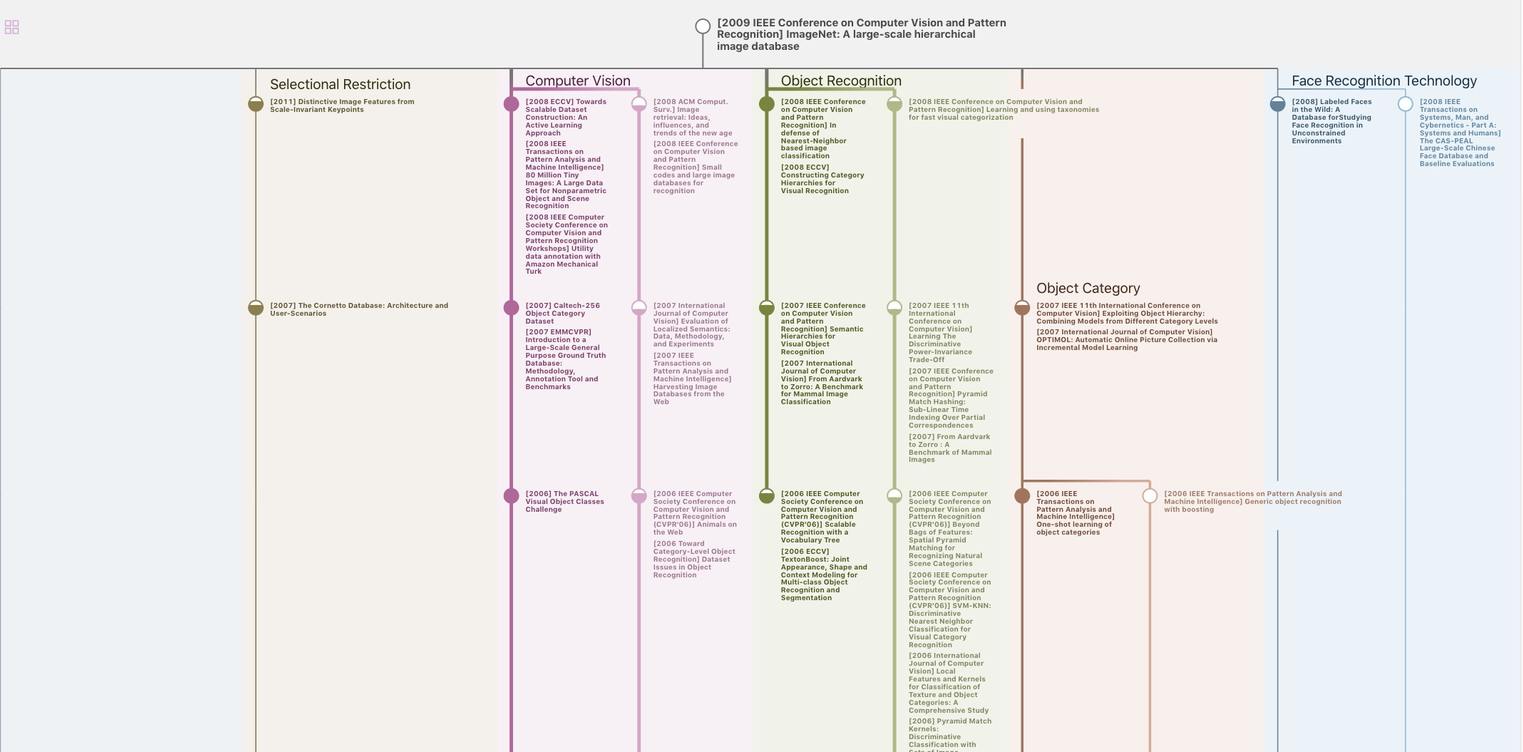
生成溯源树,研究论文发展脉络
Chat Paper
正在生成论文摘要