Analysis of Measure-Valued Derivatives in a Reinforcement Learning Actor-Critic Framework.
WSC(2022)
摘要
Policy gradient methods are successful for a wide range of reinforcement learning tasks. Traditionally, such methods utilize the score function as stochastic gradient estimator. We investigate the effect of replacing the score function with a measure-valued derivative within an on-policy actor-critic algorithm. The hypothesis is that measure-valued derivatives reduce the need for score function variance reduction techniques that are common in policy gradient algorithms. We adapt the actor-critic to measure-valued derivatives and develop a novel algorithm. This method keeps the computational complexity of the measure-valued derivative within bounds by using a parameterized state-value function approximation. We show empirically that measure-valued derivatives have comparable performance to score functions on the environments Pendulum and MountainCar. The empirical results of this study suggest that measure-valued derivatives can serve as low-variance alternative to score functions in on-policy actor-critic and indeed reduce the need for variance reduction techniques.
更多查看译文
关键词
derivatives,learning,measure-valued,actor-critic
AI 理解论文
溯源树
样例
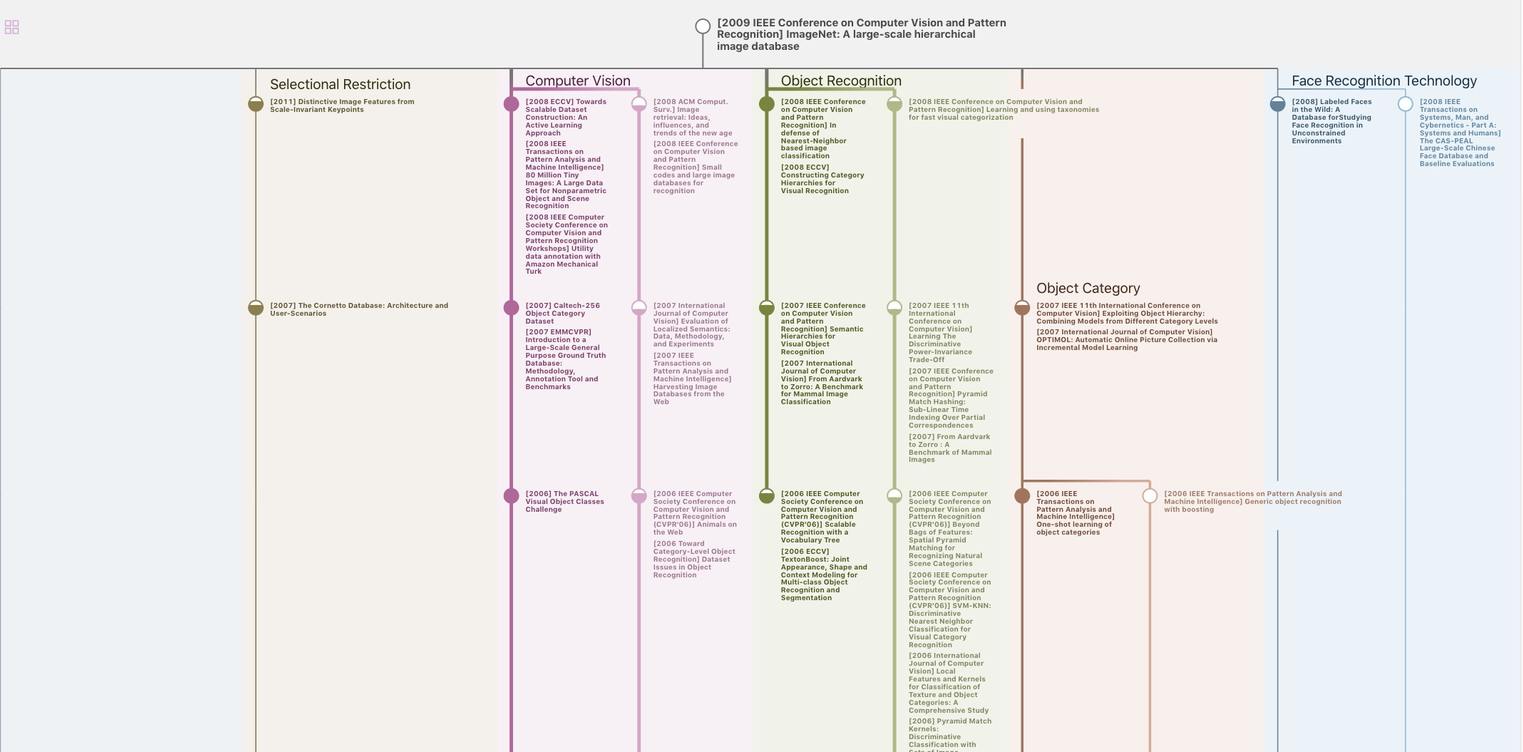
生成溯源树,研究论文发展脉络
Chat Paper
正在生成论文摘要