Robust simulation optimization with stratification
WSC(2022)
摘要
Stratification has been widely used as a variance reduction technique when estimating a simulation output, whereby the input variates are generated following a stratified sampling rule from previously determined strata. This study shows that an adaptive sampling class of simulation optimization solvers called ASTRO-DF could become more robust with stratification, S-ASTRO-DF. For a simulation optimization algorithm, we discuss how to monitor the robustness in terms of bias and variance of the outcome and introduce several metrics to compute and compare the robustness of solvers. We find that while stratified sampling improves the algorithm's performance, its robustness is sensitive to the stratification structure. In particular, as the number of strata increases, the stratified sampling-based algorithms may become less effective.
更多查看译文
关键词
adaptive sampling class,ASTRO-DF,robust simulation optimization algorithm,S-ASTRO-DF,stratification structure,stratified sampling rule,stratified sampling-based algorithms,variance reduction technique
AI 理解论文
溯源树
样例
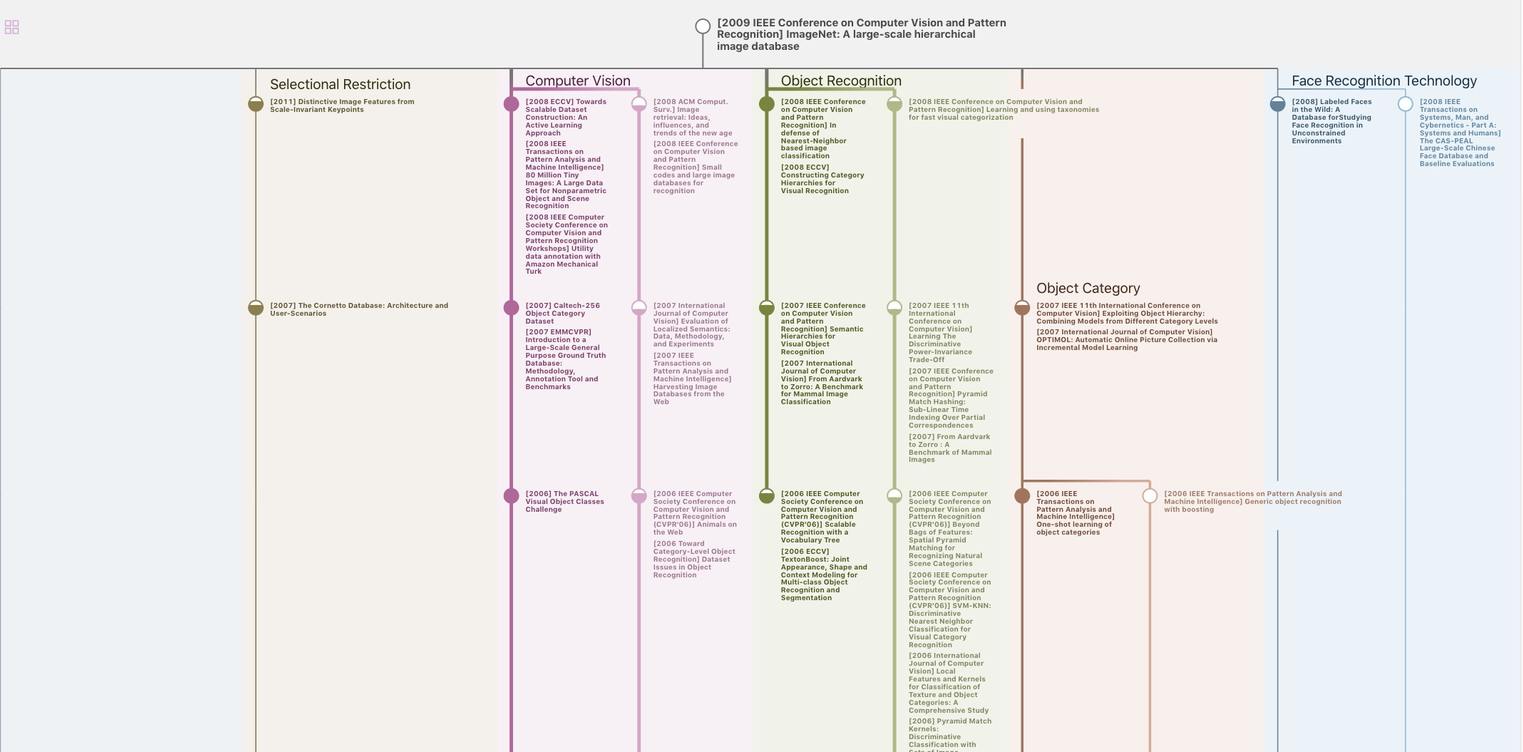
生成溯源树,研究论文发展脉络
Chat Paper
正在生成论文摘要