Feature Patch Based Attention Model for Dental Caries Classification.
CLIP@MICCAI(2022)
摘要
Dental caries is a common dental disease. According to statistics, about 90% of adults suffer from dental caries. Therefore, early detection and treatment of dental caries are crucial to dental health. According to the depth of carious lesions, dental caries can be classified into shallow, moderate, and deep caries. Among them, the accurate classification of moderate caries and deep caries is important to making the subsequent treatment plan. Clinically, doctors can make the diagnosis with the help of CBCT images. However, due to the spatial complexity of the 3D volume, the difficulty of labeling the carious lesion, and the insignificant difference between moderate and deep caries, there is still a great challenge to accurately identifying moderate and deep caries. And to the best of our knowledge, there is no study on automatic dental caries classification based on CBCT images. In this paper, we propose a feature patch based attention model to improve the classification accuracy of dental caries in CBCT images. We extract overlapping patches from the 3D feature maps and assign every patch with a corresponding weight computed by adaptive learning to achieve automatic screening of regions that are critical for classification. We collect a real-world dental dataset which includes 167 CBCT scans with moderate caries and 157 CBCT scans with deep caries. A series of experiments demonstrate that our algorithm achieves 92% accuracy on caries classification, which outperforms state-of-the-art methods by a large margin.
更多查看译文
关键词
Dental caries diagnosis, Attention mechanism, Dental cbct
AI 理解论文
溯源树
样例
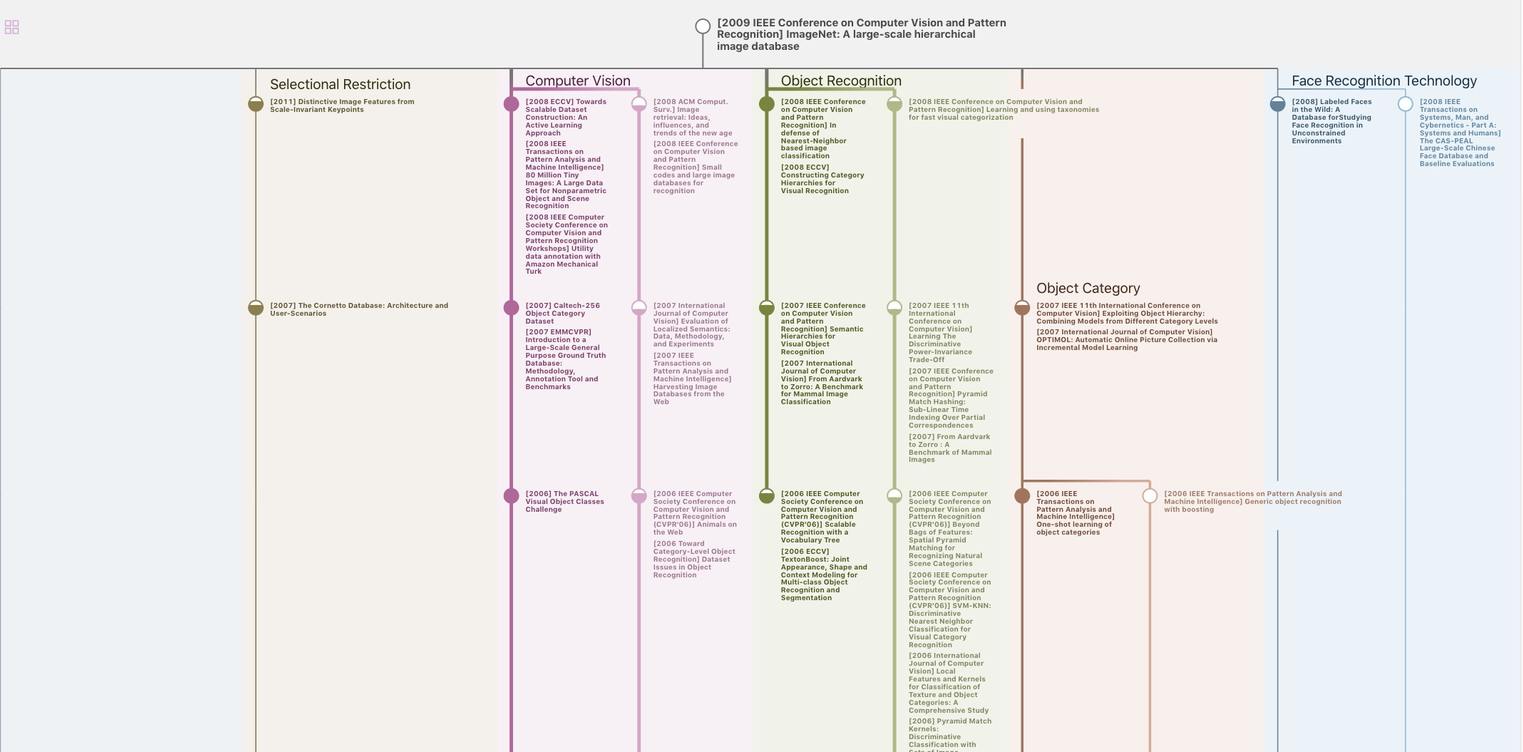
生成溯源树,研究论文发展脉络
Chat Paper
正在生成论文摘要