Distributed Data Minimization for Decentralized Collaborative Filtering Systems
PROCEEDINGS OF THE 24TH INTERNATIONAL CONFERENCE ON DISTRIBUTED COMPUTING AND NETWORKING, ICDCN 2023(2023)
摘要
Data minimization is a legal principle that mandates the limitation of personal data to a necessary minimum in order to protect the privacy of individuals. In this light, we address ourselves to decentralized collaborative filtering systems in which individual users interact with each other instead of institutional recommendation providers to obtain recommendations. Decentralized collaborative filtering systems, in which data is distributed over all users, bear a privacy benefit over traditional centralized systems in which data is kept at a single authoritative node. We propose the first formal definition of data minimization for use in distributed systems in order to combine the privacy benefits of data minimization and data distribution. On the basis of this definition, we propose a distributed data minimization scheme based on Distributed Gradient Descent (DGD) that solves the data minimization problem for decentralized collaborative filtering systems. Here, data minimization represents a collection minimization problem in which users aim to collect necessary minimum amounts of rating data from other users in the system. We find by means of simulation that the overall amount of data collected by all users can be reduced significantly (> 14.6%) without jeopardizing recommendation performance, when users coordinate data collection. We thus prove that users collect unnecessarily much data, when they do not coordinate data collection.
更多查看译文
关键词
data minimization,recommender systems,decentralized collaborative filtering,distributed systems,distributed gradient descent,redundancy minimization
AI 理解论文
溯源树
样例
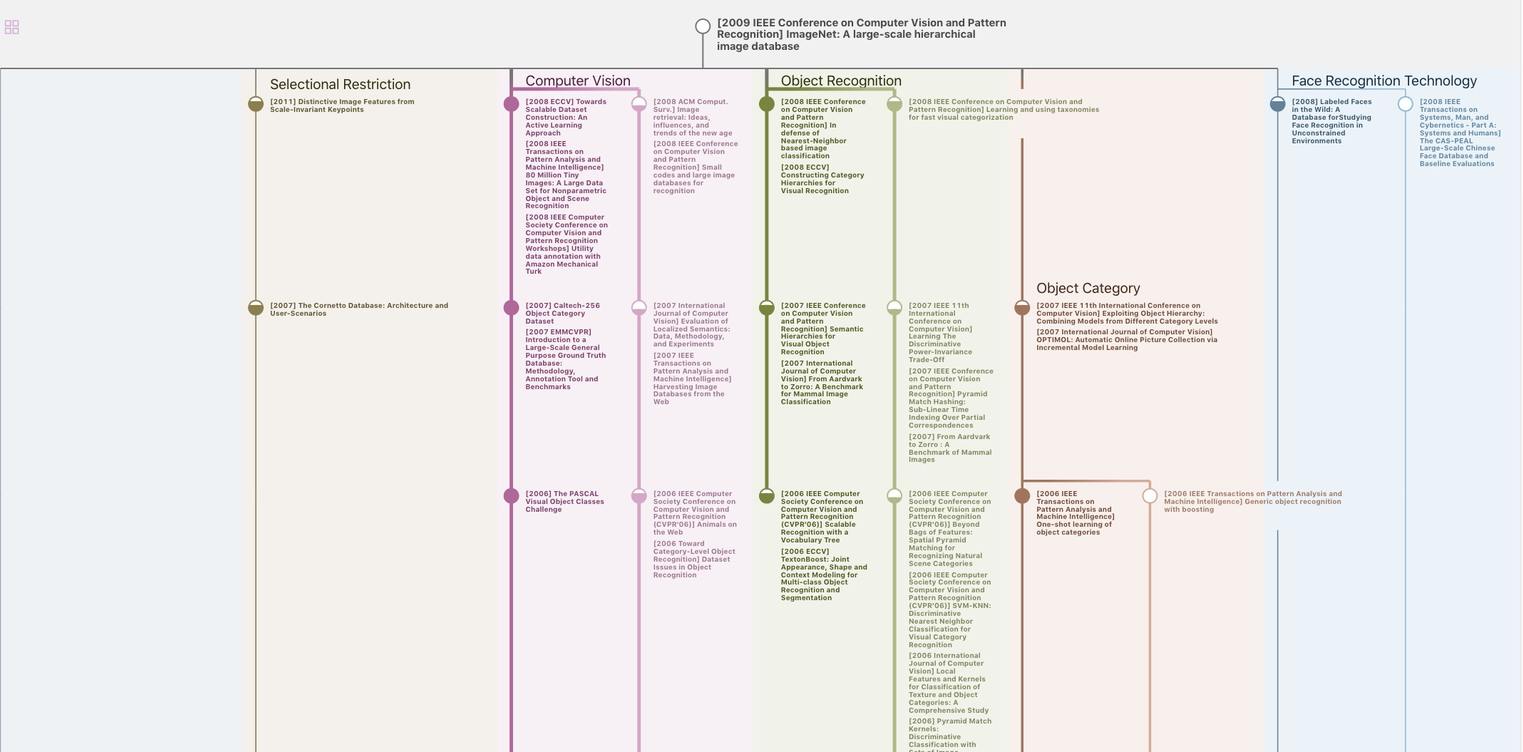
生成溯源树,研究论文发展脉络
Chat Paper
正在生成论文摘要