Evolving Interpretable Classification Models via Readability-Enhanced Genetic Programming.
SSCI(2022)
摘要
As the impact of Machine Learning (ML) on business and society grows, there is a need for making opaque ML models transparent and interpretable, especially in the light of fairness, bias, and discrimination. Nevertheless, interpreting complex opaque models is not trivial. Current interpretability approaches rely on local explanations or produce long explanations that tend to overload the user's cognitive abilities. In this paper, we address this problem by extracting interpretable, transparent models from opaque ones via a new readability-enhanced multiobjective Genetic Programming approach called REMO-GP. To achieve that, we adapt text readability metrics into model complexity proxies that support evaluating ML interpretability. We demonstrate that our approach can generate global interpretable models that mimic the decisions of complex opaque models over several datasets, while keeping model complexity low.
更多查看译文
关键词
Artificial Intelligence,Opaque Models,Genetic Programming,Interpretability,Binary Classification
AI 理解论文
溯源树
样例
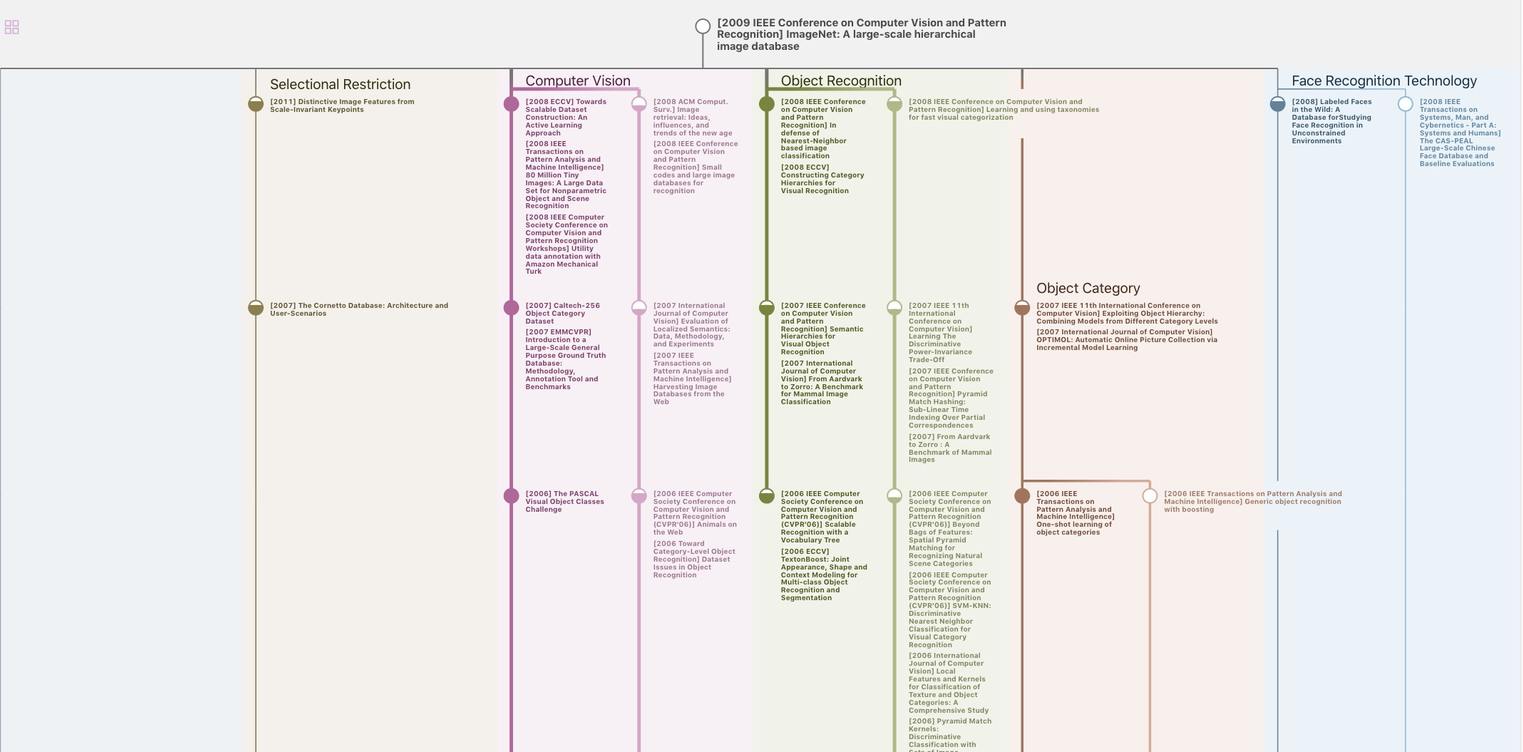
生成溯源树,研究论文发展脉络
Chat Paper
正在生成论文摘要