Riemannian Geometric Instance Filtering for Transfer Learning in Brain-Computer Interfaces
SenSys(2022)
摘要
Due to the inter-subject variability of Electroencephalogram(EEG) signals, a long calibration time is required to collect a large number of labeled trials to calibrate classifier parameters before using the Brain-computer Interface(BCI). This challenge greatly limits the practical roll-out of BCIs. To address this problem, we propose a novel instance-based transfer learning framework named Riemannian Geometric Instance Filtering (RGIF) to reduce calibration time without sacrificing accuracy. A new inter-subject similarity metric based on Riemannian geometry is proposed to measure the similarity between a few trials from the target subject and adequate trials from source subjects. The classification model for the target subject is then trained with the help of abundant trials from similar source subjects with high similarity to the target subject. We evaluate our method on two open-source EEG datasets. The results show that our approach improves significantly compared with other baselines. Furthermore, compared with using all source subjects data, our method reduces the training time by at least half and achieves slightly better accuracy.
更多查看译文
关键词
Brain-computer interface, motor imagery, transfer learning, Riemannian geometry, inter-subject similarity
AI 理解论文
溯源树
样例
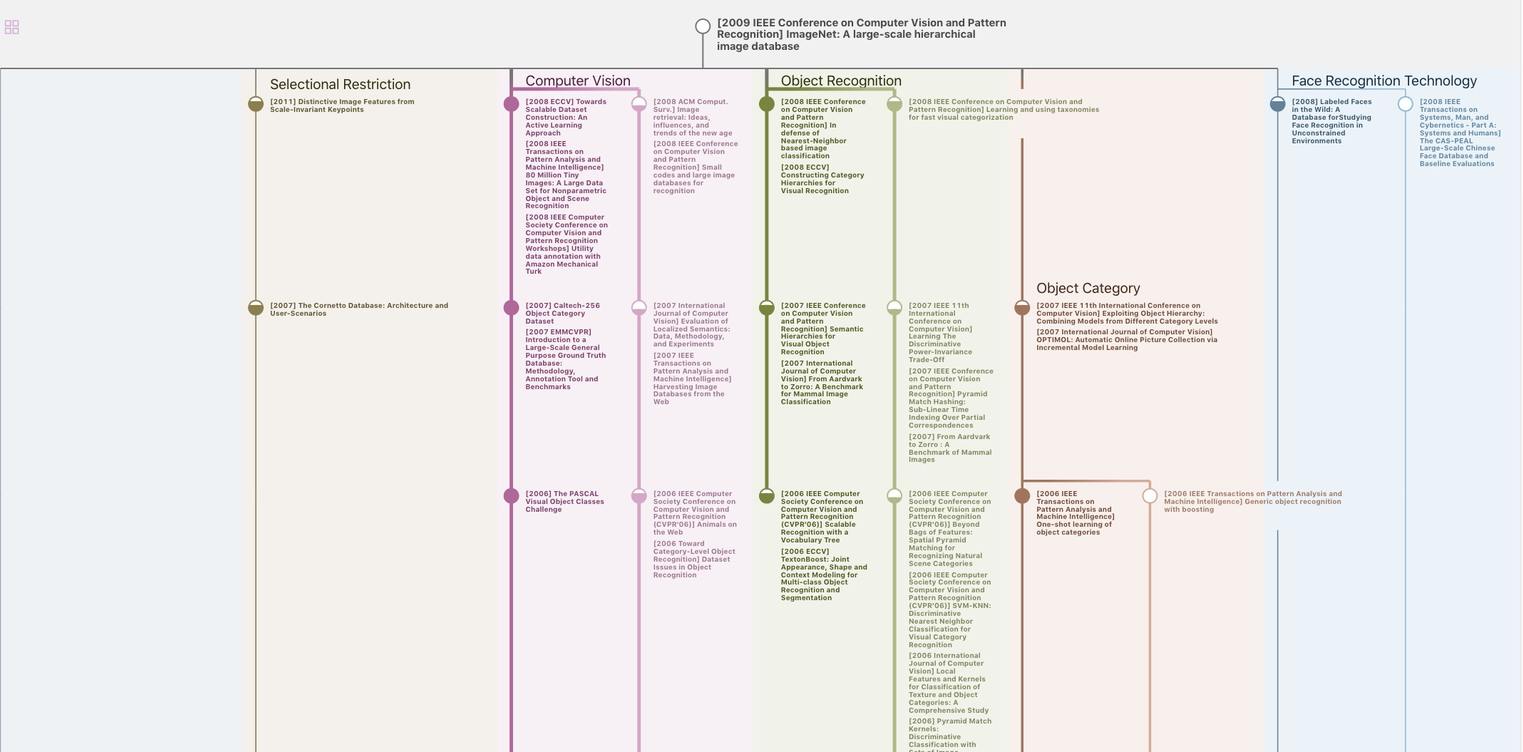
生成溯源树,研究论文发展脉络
Chat Paper
正在生成论文摘要