A Zero-Shot Approach to Identifying Children's Speech in Automatic Gender Classification.
SLT(2022)
摘要
Detecting whether a speech utterance belongs to an adult male, adult female or a child category, also known as malefemale-child (MFC) classification is particularly challenging due to two main reasons - paucity of children's speech data, and high variability in children's speech due to developmental changes. It is difficult to obtain speech datasets with children's voices due to privacy reasons. This paper explores a zero-shot learning approach to MFC classification. Different algorithms are explored to create artificial childlike voices from adult voices. Methods such as pitch shifting, Vocal Tract Length Perturbation, and Segmental Warping Perturbation are used to create synthetic childlike speech for the MFC classification task. Speaker embeddings extracted from a DNN based speaker recognition system are used as features for MFC classification. Compared to a pitch frequency based baseline MFC classifier, the proposed method improves the child classification accuracy by 47%.
更多查看译文
关键词
Children's speech, gender classification, age estimation, voice attributes, spectral warping, data augmentation, vocal tract length perturbation
AI 理解论文
溯源树
样例
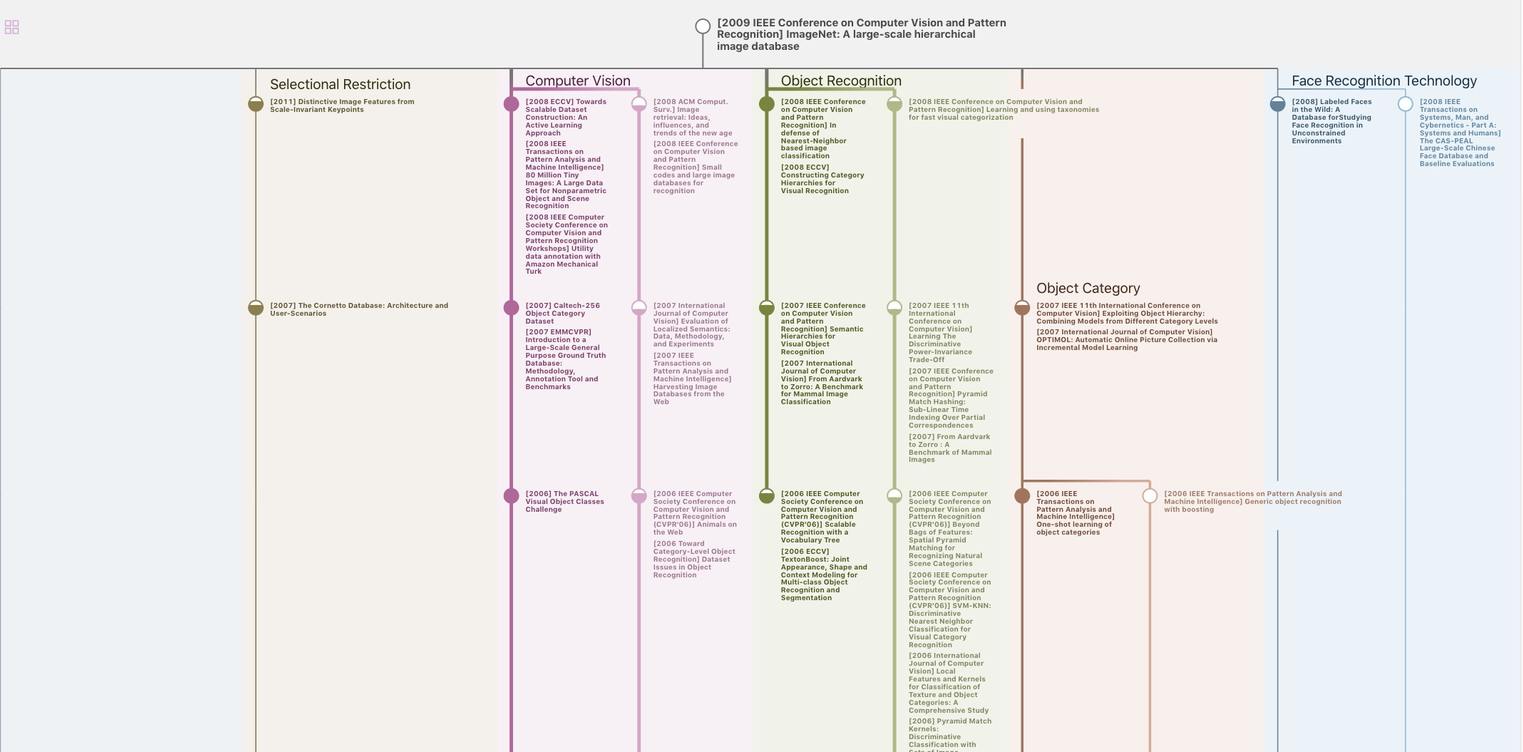
生成溯源树,研究论文发展脉络
Chat Paper
正在生成论文摘要