Code-Switched Language Modelling Using a Code Predictive Lstm in Under-Resourced South African Languages.
SLT(2022)
摘要
We present a new LSTM language model architecture for code-switched speech incorporating a neural structure that explicitly models language switches. Experimental evaluation of this code predictive model for four under-resourced South African languages shows consistent improvements in perplexity as well as perplexity specifically over code-switches compared to an LSTM baseline. Substantial reductions in absolute speech recognition word error rates (0.5%-1.2%) as well as errors specifically at code-switches (0.6%-2.3%) are also achieved during n-best rescoring. When used for both data augmentation and n-best rescoring, our code predictive model reduces word error rate by a further 0.8%-2.6% absolute and consistently outperforms a baseline LSTM. The similar and consistent trends observed across all four language pairs allows us to conclude that explicit modelling of language switches by a dedicated language model component is a suitable strategy for code-switched speech recognition.
更多查看译文
关键词
Code-switching,Bantu languages,n-best rescoring,language model data augmentation,speech recognition,under-resourced languages
AI 理解论文
溯源树
样例
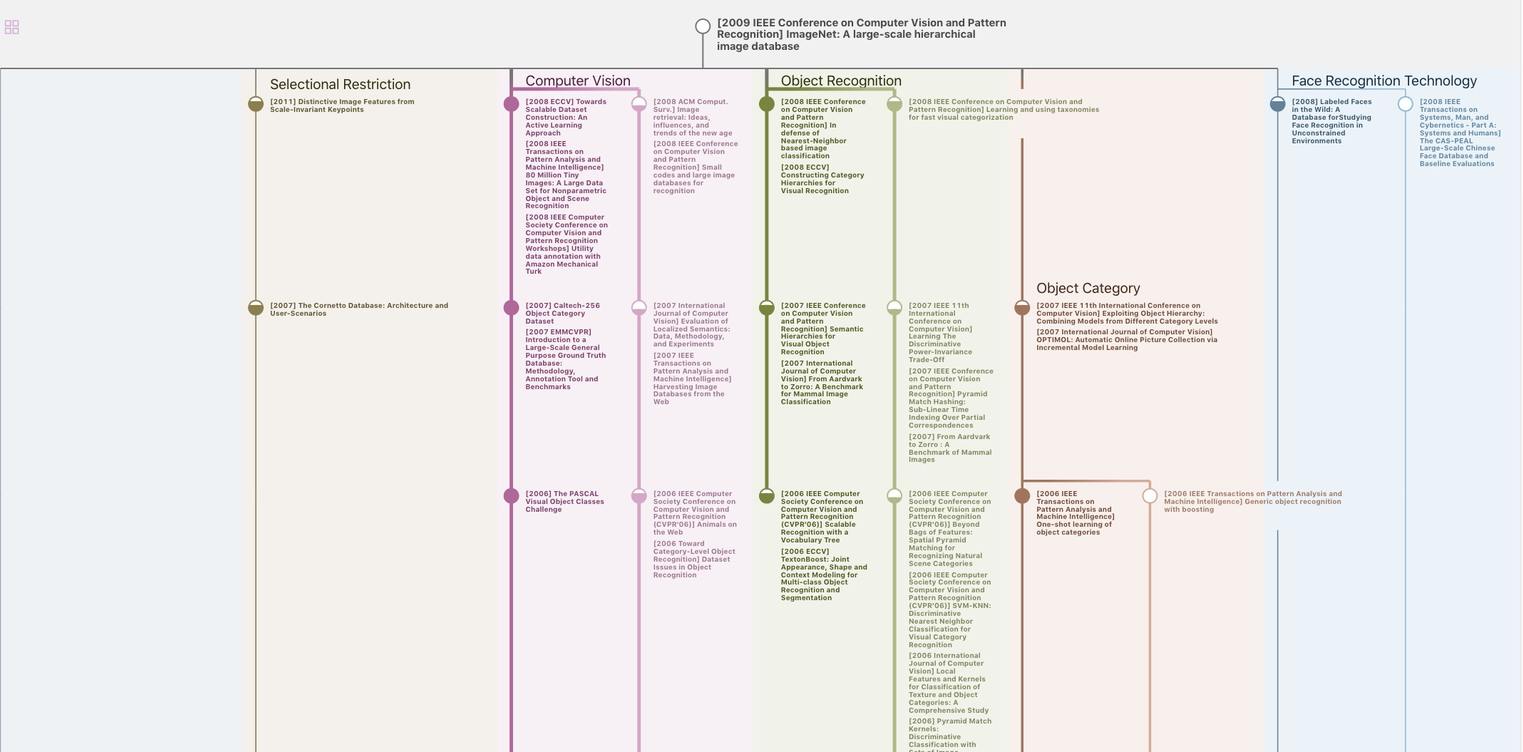
生成溯源树,研究论文发展脉络
Chat Paper
正在生成论文摘要