QoE-Guaranteed Heterogeneous Task Offloading with Deep Reinforcement Learning in Edge Computing.
CCIS(2022)
摘要
In edge-enabled Internet-of-Things (IoT), various IoT applications commonly generate heterogeneous tasks. Existing heterogeneous task offloading methods are developed to guarantee the Quality-of-Service (QoS), instead of the Quality-of-Experience (QoE) from the user’s perspective. However, QoE-guaranteed heterogeneous task offloading can significantly improve the actual user experiences of IoT applications. In this paper, the heterogeneity of offloading tasks is categorized as delay-aware, energy-aware, and privacy-aware. We define a novel QoE metric and adopt a statistical policy to relax the deterministic constraints of heterogeneous tasks to improve the offloading success rate. Furthermore, we formulate the QoE-guaranteed heterogeneous tasks offloading problem as a Mixed-integer Nonlinear Programming (MINLP) problem. Conventional numerical optimization methods are inefficient in solving such problems, therefore, we apply a Deep Reinforcement Learning (DRL) algorithm to make the optimal offloading decision that satisfies the offloading intention of the task. Experiment results show that our algorithm effectively guarantees the global QoE performance and improves the offloading success rate of heterogeneous tasks.
更多查看译文
关键词
Task offloading,heterogeneous task,edge computing,Quality of Experience (QoE)
AI 理解论文
溯源树
样例
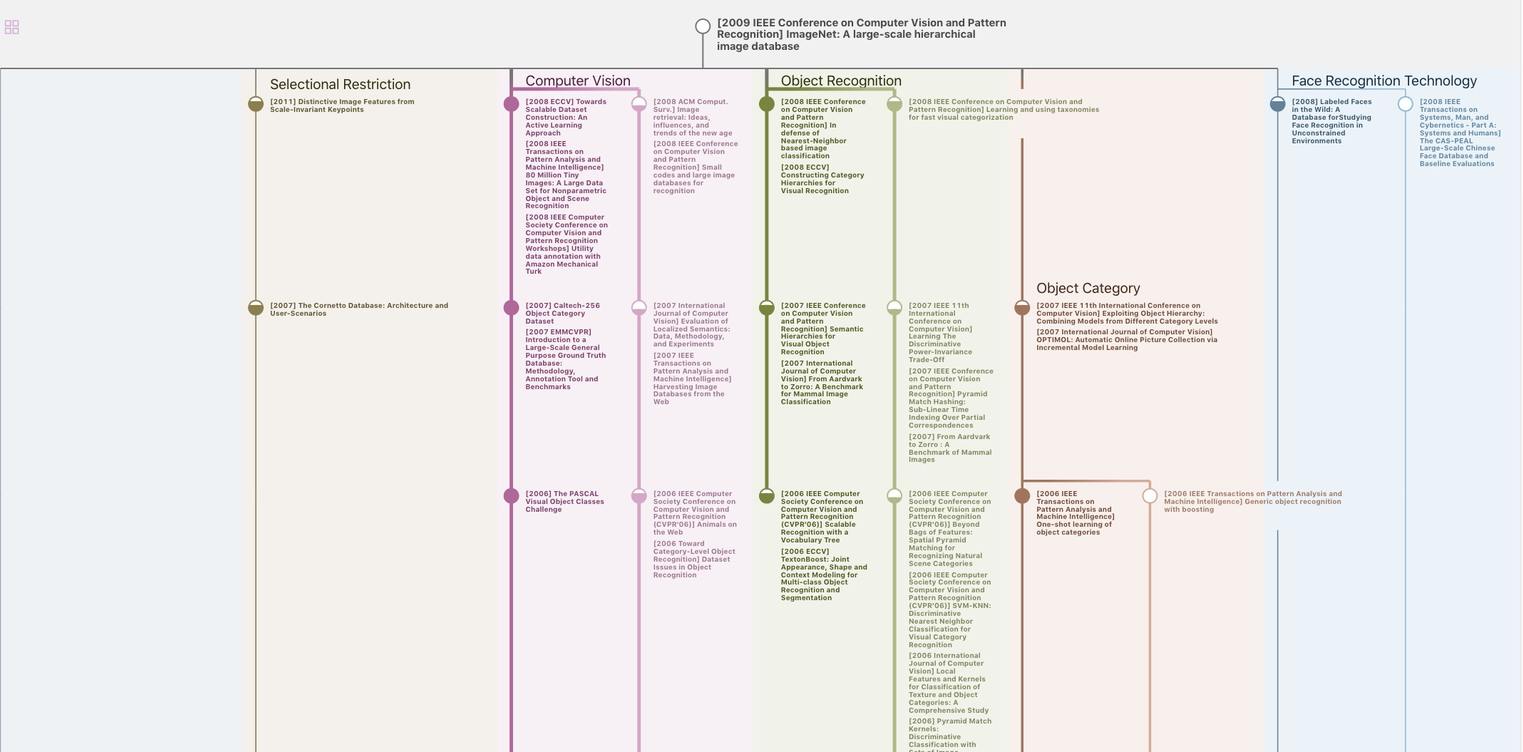
生成溯源树,研究论文发展脉络
Chat Paper
正在生成论文摘要