Channel Estimation for Reconfigurable Intelligent Surface Assisted Wireless Communications via Structured Sparse Bayesian Learning.
VTC Fall(2022)
摘要
This paper investigates the challenging channel estimation problem for reconfigurable intelligent surface assisted wireless communications. By exploiting the channel's structured-sparsity and two-timescale characteristics, a three-stage channel estimation method based on structured sparse Bayesian learning (SBL) is proposed to improve the estimation accuracy and reduce the pilot overhead. The row support set is first estimated at the large timescale to reduce the dimension of sparse channel matrix. A structured SBL algorithm is then designed to estimate the block sparse channel vector at the small timescale. The estimated support set and block sparse vector are finally used to reconstruct the channel. The computational complexity of the proposed method is analyzed. Numerical results show that the proposed method outperforms state-of-the-art benchmarks in terms of estimation accuracy, pilot overhead and robustness.
更多查看译文
关键词
Reconfigurable intelligent surface,channel estimation,structured sparsity,sparse Bayesian learning
AI 理解论文
溯源树
样例
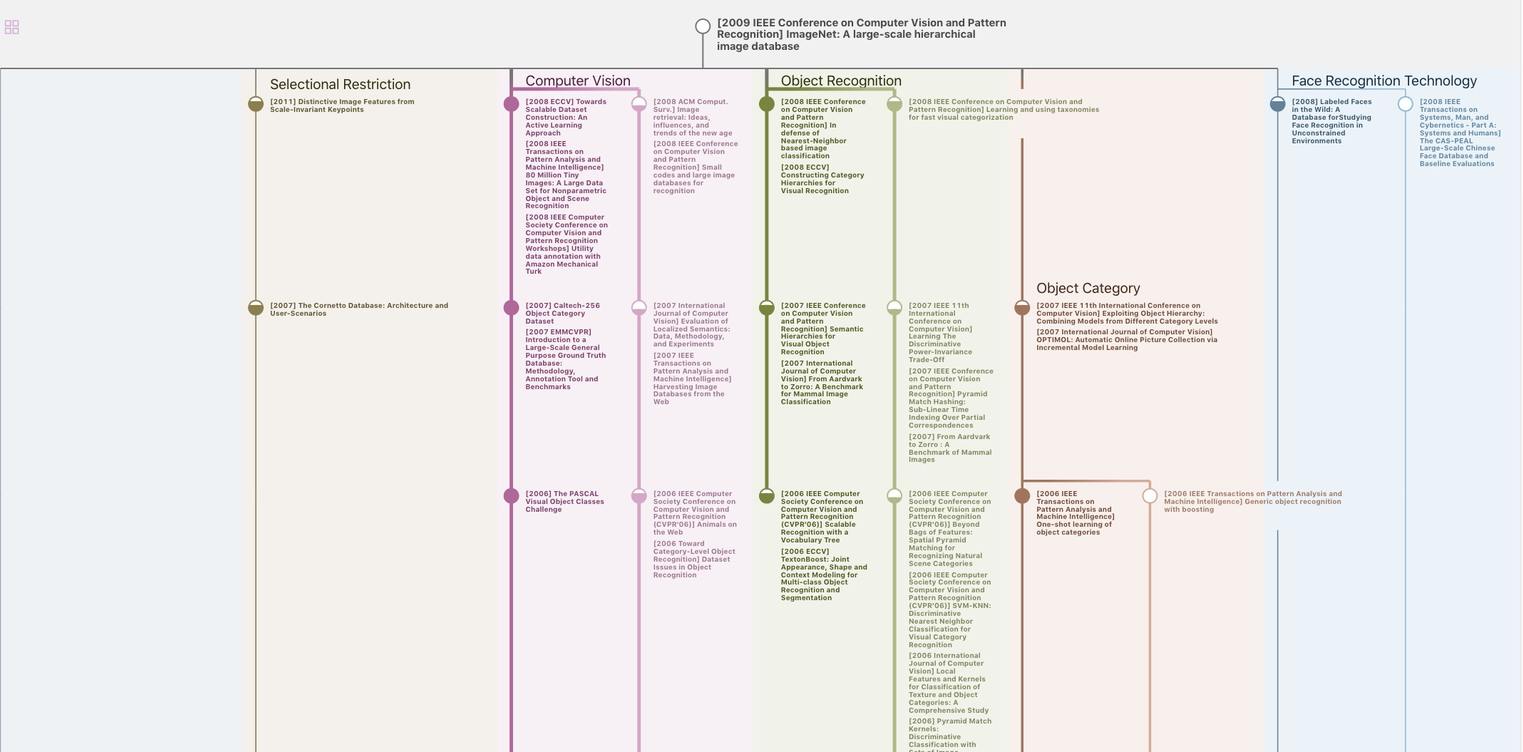
生成溯源树,研究论文发展脉络
Chat Paper
正在生成论文摘要