A Hybrid CNN-LSTM for Battery Remaining Useful Life Prediction with Charging Profiles Data.
IC3INA(2022)
摘要
The capacity degradation of battery can occur due to continuously used as primary energy source equipment. An accurate prediction of battery remaining useful life (RUL) is necessary to avoid system functionality failure. This study proposes battery RUL prediction using data-driven method based on a hybrid deep model of Convolutional Neural Networks (CNN) and Long-Short Term Memory (LSTM). CNN and LSTM are used to extract features from multiple measurable data in parallel. CNN extracts features of multi-channel charging profiles, whereas LSTM extracts features of historical capacity data of discharging profiles which related to time dependency. An error index is compared between single model LSTM and hybrid model CNN-LSTM. The result indicates that the proposed hybrid model outperforms the single model by up to 37%-61% in case of mean absolute percentage error.
更多查看译文
AI 理解论文
溯源树
样例
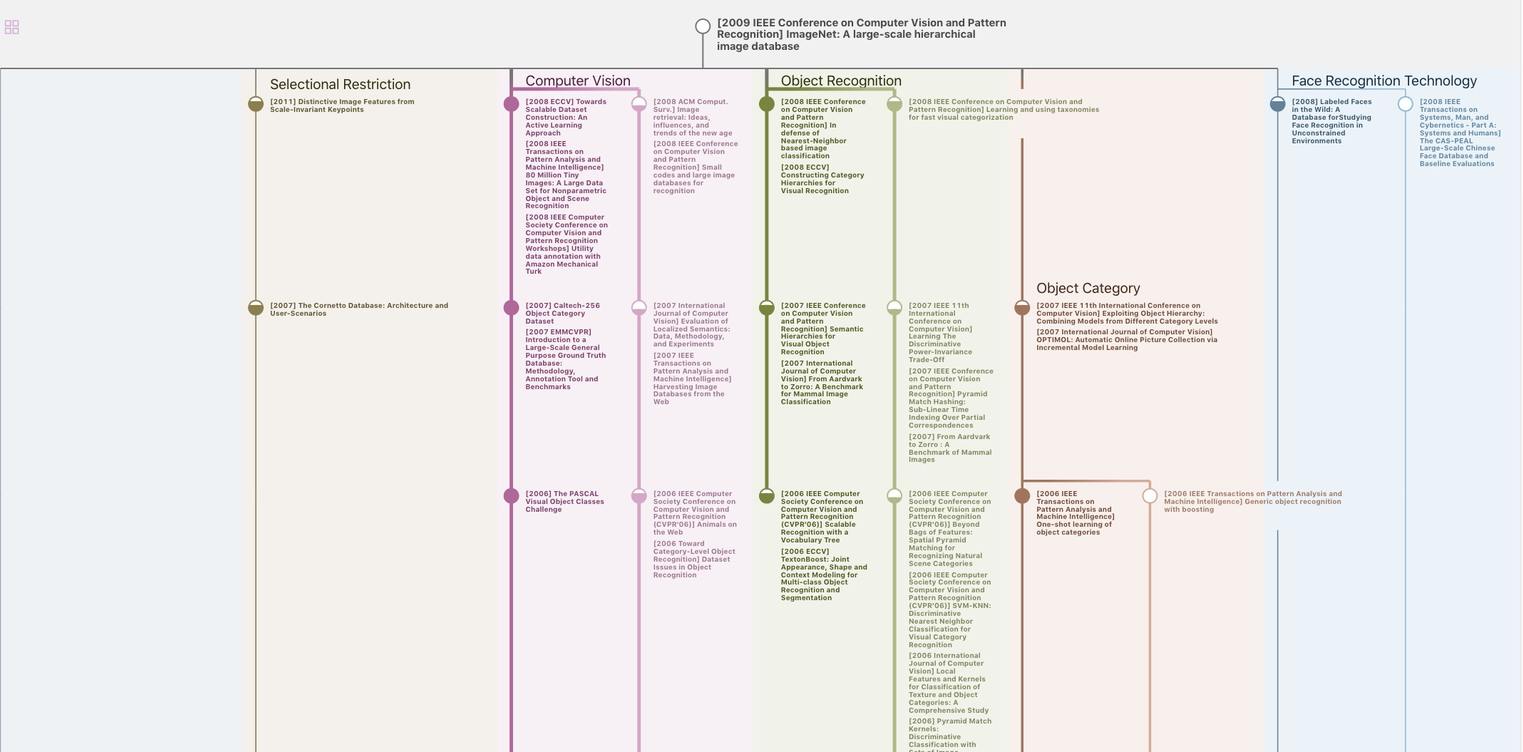
生成溯源树,研究论文发展脉络
Chat Paper
正在生成论文摘要