Application of YOLOv5 and MASK R-CNN for diurnal activity monitoring of freshwater fish.
SCIS/ISIS(2022)
摘要
Real-time monitoring of aquatic environment such as fish is an important task for a deeper understanding of aquatic ecosystems. While underwater monitoring has been studied intensively, it is still challenging to monitor target ecosystems during night-time. In this study, we aim to develop an image processing pipeline to monitor small freshwater fish in day and night. For this purpose, we used instance segmentation and object detection as an image processing technique specifically for detecting fish individuals in an image. We collected images of our target fish, Nipponocypris temminckii in a spring-fed stream in Tokyo. Images were taken using hand-held digital cameras during the daytime for model training and validation. We then applied data augmentation for model training by adjusting brightness and sharpness, reducing image resolution, and copy-paste augmentation, from which six data sets were prepared by additively combining these methods. Also, in the laboratory aquarium experiment, time-lapse images of the target fish were taken from two directions. The images taken from the eastern side were used as test images, while the images taken from the northern side were used as training images. Half of them were taken in the evening using an infrared camera. We first trained a Mask R-CNN on each of the training data sets and applied it to the test images to verify the accuracy of fish detection and to compare the applicability of the data augmentation methods employed. Then, we compared the framework by training YOLOv5 on the dataset that showed the highest accuracy in training the Mask R-CNN and images acquired in the experiment. Results showed that the combination of many data augmentation methods improved the accuracy of fish detection and allowed for fish detection with infrared images. In particular, we found that the copy-paste method improves the detection accuracy on infrared images taken under a dark condition.
更多查看译文
关键词
freshwater fish, monitoring, deep neural network, image analysis, data augmentation
AI 理解论文
溯源树
样例
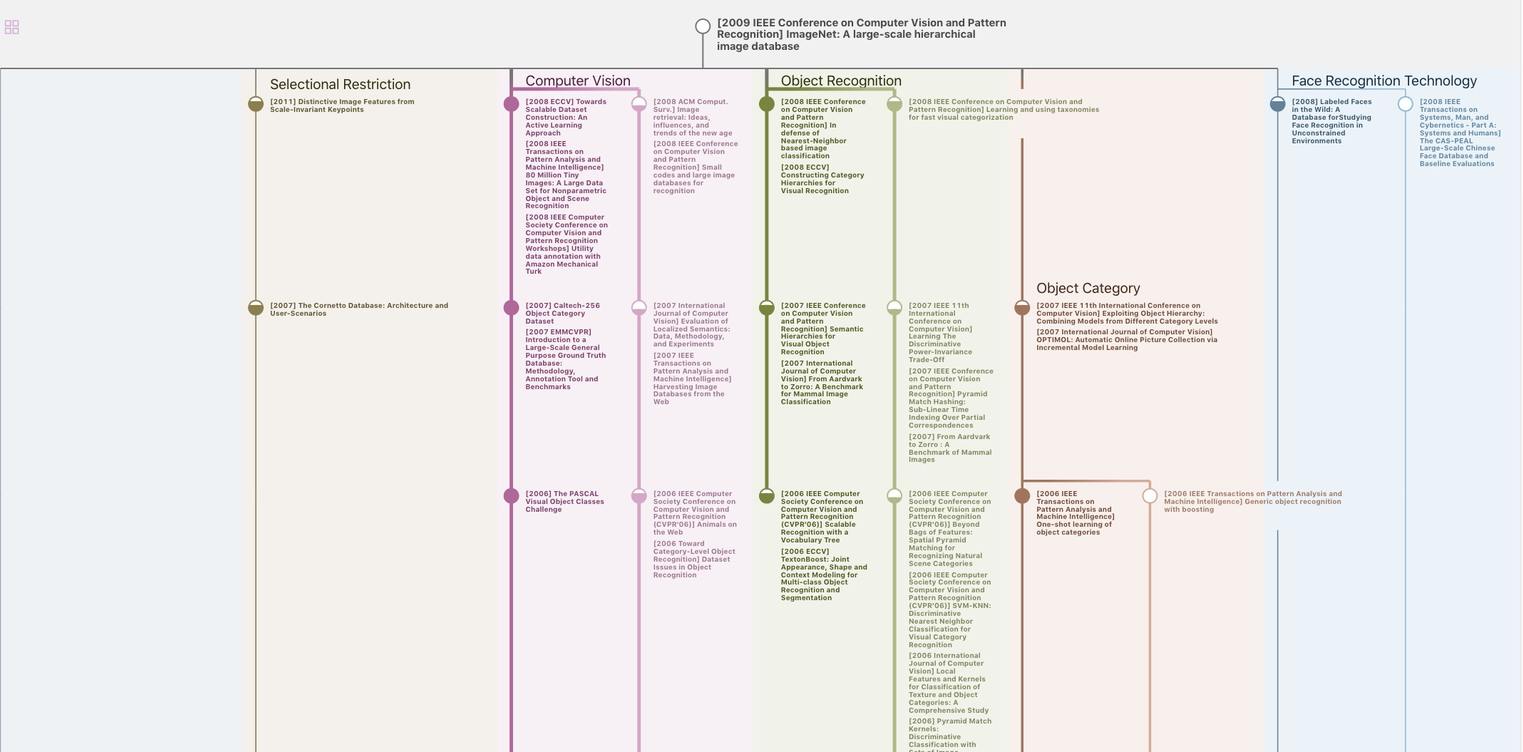
生成溯源树,研究论文发展脉络
Chat Paper
正在生成论文摘要