Marketing Budget Allocation with Offline Constrained Deep Reinforcement Learning.
WSDM(2023)
摘要
We study the budget allocation problem in online marketing campaigns that utilize previously collected offline data. We first discuss the long-term effect of optimizing marketing budget allocation decisions in the offline setting. To overcome the challenge, we propose a novel game-theoretic offline value-based reinforcement learning method using mixed policies. The proposed method reduces the need to store infinitely many policies in previous methods to only constantly many policies, which achieves nearly optimal policy efficiency, making it practical and favorable for industrial usage. We further show that this method is guaranteed to converge to the optimal policy, which cannot be achieved by previous value-based reinforcement learning methods for marketing budget allocation. Our experiments on a large-scale marketing campaign with tens-of-millions users and more than one billion budget verify the theoretical results and show that the proposed method outperforms various baseline methods. The proposed method has been successfully deployed to serve all the traffic of this marketing campaign.
更多查看译文
关键词
offline constrained deep reinforcement
AI 理解论文
溯源树
样例
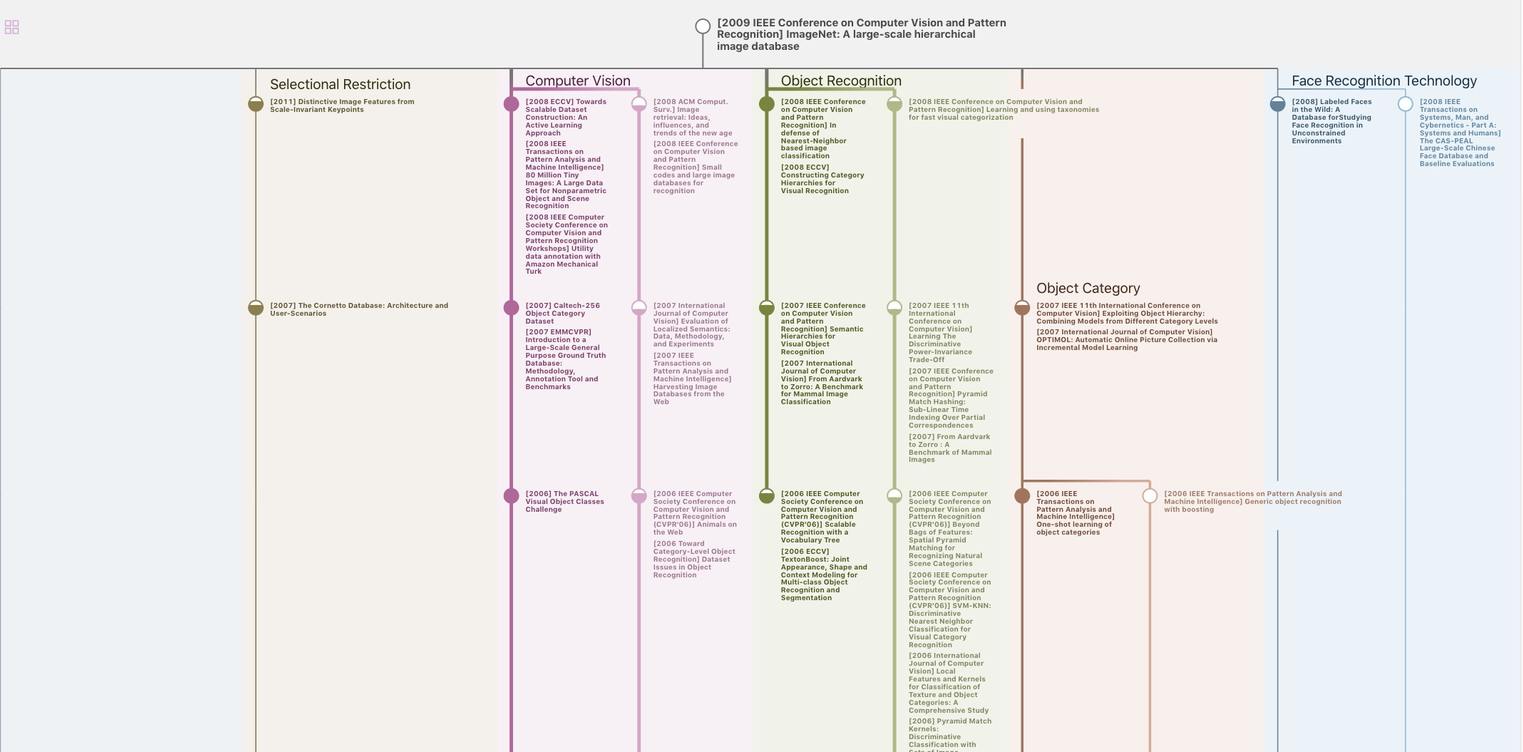
生成溯源树,研究论文发展脉络
Chat Paper
正在生成论文摘要