Power-Optimized Processor Slowdown Control.
CDC(2022)
摘要
For user-serving computational workloads in data centers (like ML/AI inference tasks with e-commerce applications), managing both the latency experienced by the user and the energy consumption of such workloads is essential. The slowdown quality-of-service metric is increasingly used in real-world systems, as it captures the perceived user delay proportional to the expected job service time. In this work, we introduce a framework to control job slowdown in a data center with multi-class workloads, by adjusting the processor rate. Increasing the processor rate decreases slowdown at the cost of additional power; the goal is to select the processor rate to optimize this tradeoff. We present optimal rate control characterizations for both 1) when the sizes of all jobs in the queue are observed and 2) when only the head-of-line job size and the total queue length are observed. We compare the controls analytically and numerically, and demonstrate how this framework can be employed to achieve desired slowdown targets with minimal power.
更多查看译文
关键词
data center,energy consumption,head-of-line job size,job service time,job slowdown,optimal rate control,power-optimized processor slowdown control,processor rate,quality of service metric,real-world systems,total queue length
AI 理解论文
溯源树
样例
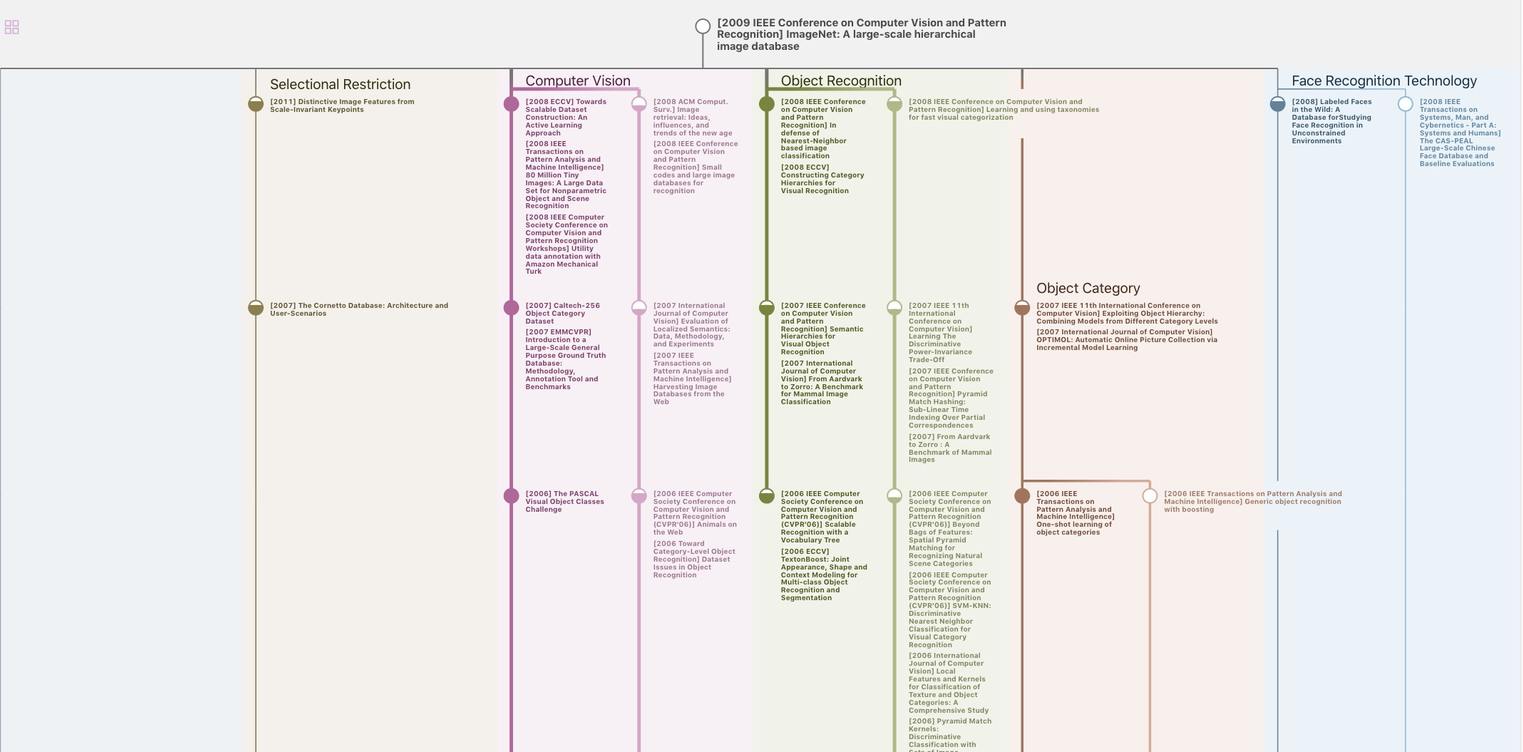
生成溯源树,研究论文发展脉络
Chat Paper
正在生成论文摘要