A Dual Accelerated Method for a Class of Distributed Optimization Problems: From Consensus to Decentralized Policy Evaluation.
CDC(2022)
摘要
Motivated by decentralized sensing and policy evaluation problems, we consider a particular type of distributed stochastic optimization problem over a network, called the online stochastic distributed averaging problem. We design a dual-based method for this distributed consensus problem with Polyak-Ruppert averaging and analyze its behavior. We show that the proposed algorithm attains an accelerated deterministic error depending optimally on the condition number of the network, and also that it has an order-optimal stochastic error. This improves on the guarantees of state-of-the-art distributed stochastic optimization algorithms when specialized to this setting, and yields-among other things-corollaries for decentralized policy evaluation. Our proofs rely on explicitly studying the evolution of several relevant linear systems, and may be of independent interest.
更多查看译文
关键词
distributed optimization problems,dual accelerated method
AI 理解论文
溯源树
样例
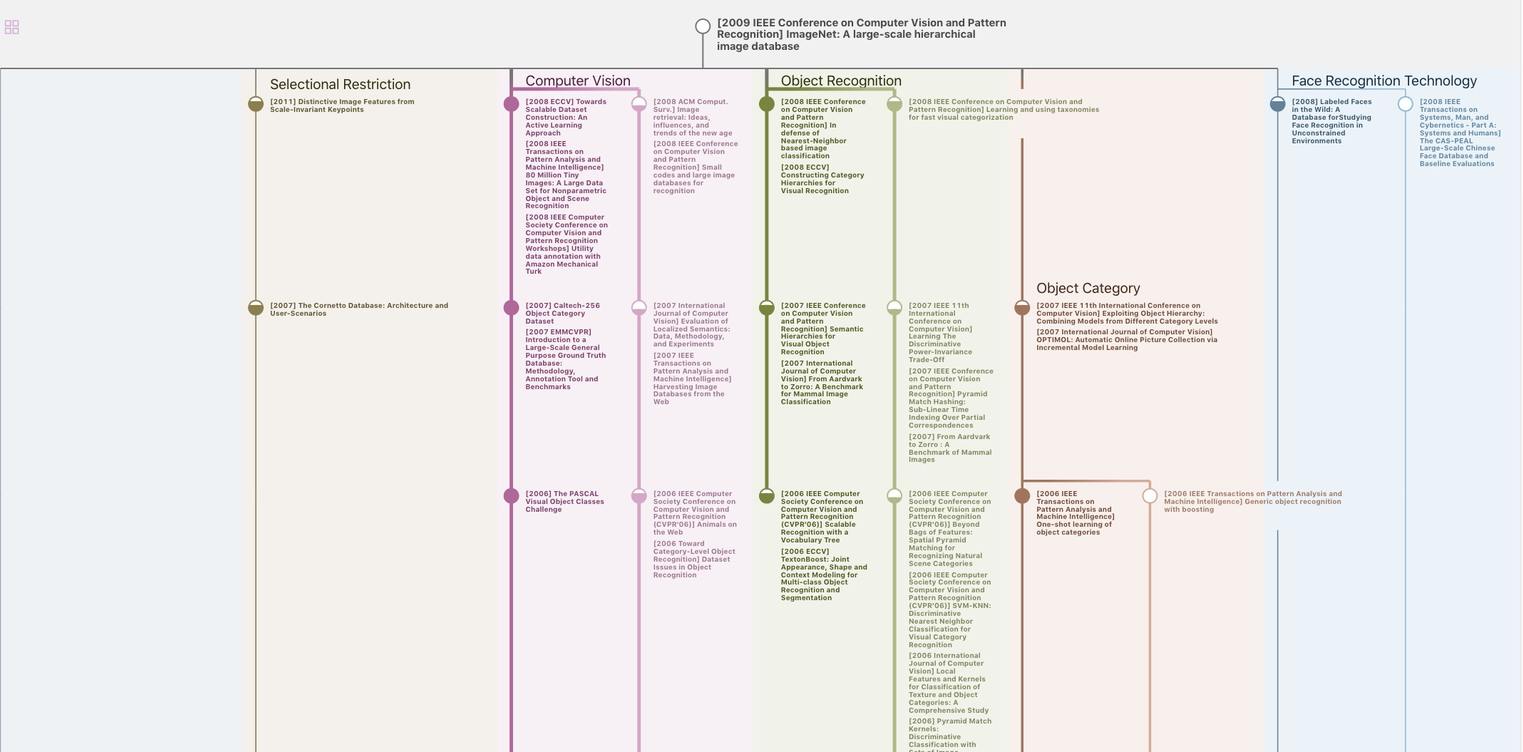
生成溯源树,研究论文发展脉络
Chat Paper
正在生成论文摘要