Safe Finite-Time Reinforcement Learning for Pursuit-Evasion Games.
CDC(2022)
摘要
In this paper, we develop a safe pursuit-evasion game for enabling finite-time capture and optimal performance. The pursuit-evasion game is formulated as a zero-sum differential game wherein the pursuer seeks to minimize its relative distance to the target while the evader attempts to maximize it. A critic-only reinforcement learning-based algorithm is then proposed for learning online and in finite time the pursuit-evasion policies, thus enabling finite-time capture of the evader. Safety is ensured by means of barrier functions associated with the obstacles, which are integrated into the running cost. Simulation results illustrate the efficacy of the proposed approach.
更多查看译文
关键词
Safety, online adaptive learning, finite-time stability, differential games
AI 理解论文
溯源树
样例
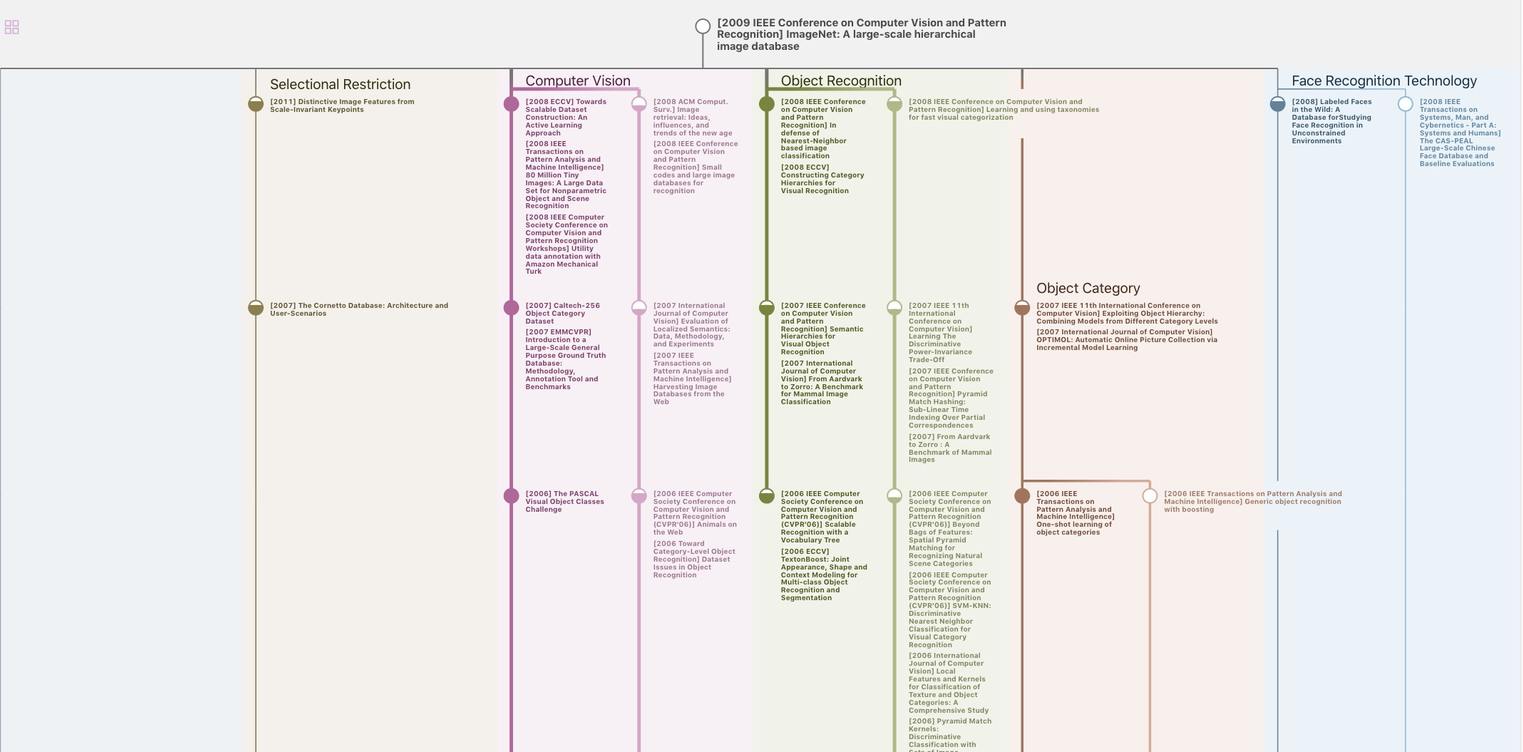
生成溯源树,研究论文发展脉络
Chat Paper
正在生成论文摘要