Uncertain uncertainty in data-driven stochastic optimization: towards structured ambiguity sets.
CDC(2022)
摘要
Ambiguity sets of probability distributions are a prominent tool to hedge against distributional uncertainty in stochastic optimization. The aim of this paper is to build tight Wasserstein ambiguity sets for data-driven optimization problems. The method exploits independence between the distribution components to introduce structure in the ambiguity sets and speed up their shrinkage with the number of collected samples. Tractable reformulations of the stochastic optimization problems are derived for costs that are expressed as sums or products of functions that depend only on the individual distribution components. The statistical benefits of the approach are theoretically analyzed for compactly supported distributions and demonstrated in a numerical example.
更多查看译文
关键词
stochastic optimization,uncertainty,ambiguity,data-driven
AI 理解论文
溯源树
样例
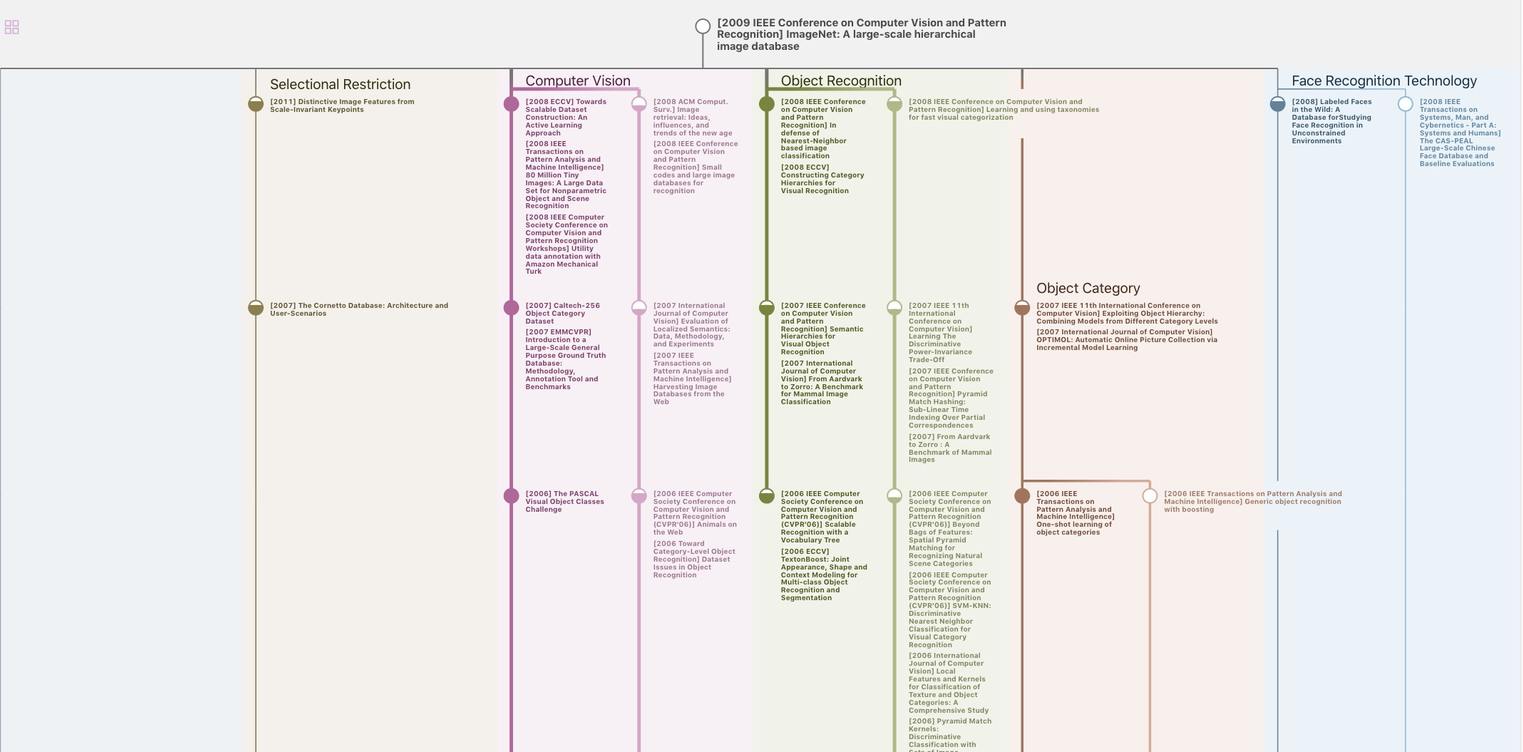
生成溯源树,研究论文发展脉络
Chat Paper
正在生成论文摘要