Distributionally Robust Optimization via Haar Wavelet Ambiguity Sets.
CDC(2022)
摘要
This paper introduces a spectral parameterization of ambiguity sets to hedge against distributional uncertainty in stochastic optimization problems. We build an ambiguity set of probability densities around a histogram estimator, which is constructed by independent samples from the unknown distribution. The densities in the ambiguity set are determined by bounding the distance between the coefficients of their Haar wavelet expansion and the expansion of the histogram estimator. This representation facilitates the computation of expectations, leading to tractable minimax problems that are linear in the parameters of the ambiguity set, and enables the inclusion of additional constraints that can capture valuable prior information about the unknown distribution.
更多查看译文
关键词
distributional uncertainty,distributionally robust optimization,Haar wavelet ambiguity sets,Haar wavelet expansion,histogram estimator,minimax problems,probability densities,spectral parameterization,stochastic optimization,unknown distribution
AI 理解论文
溯源树
样例
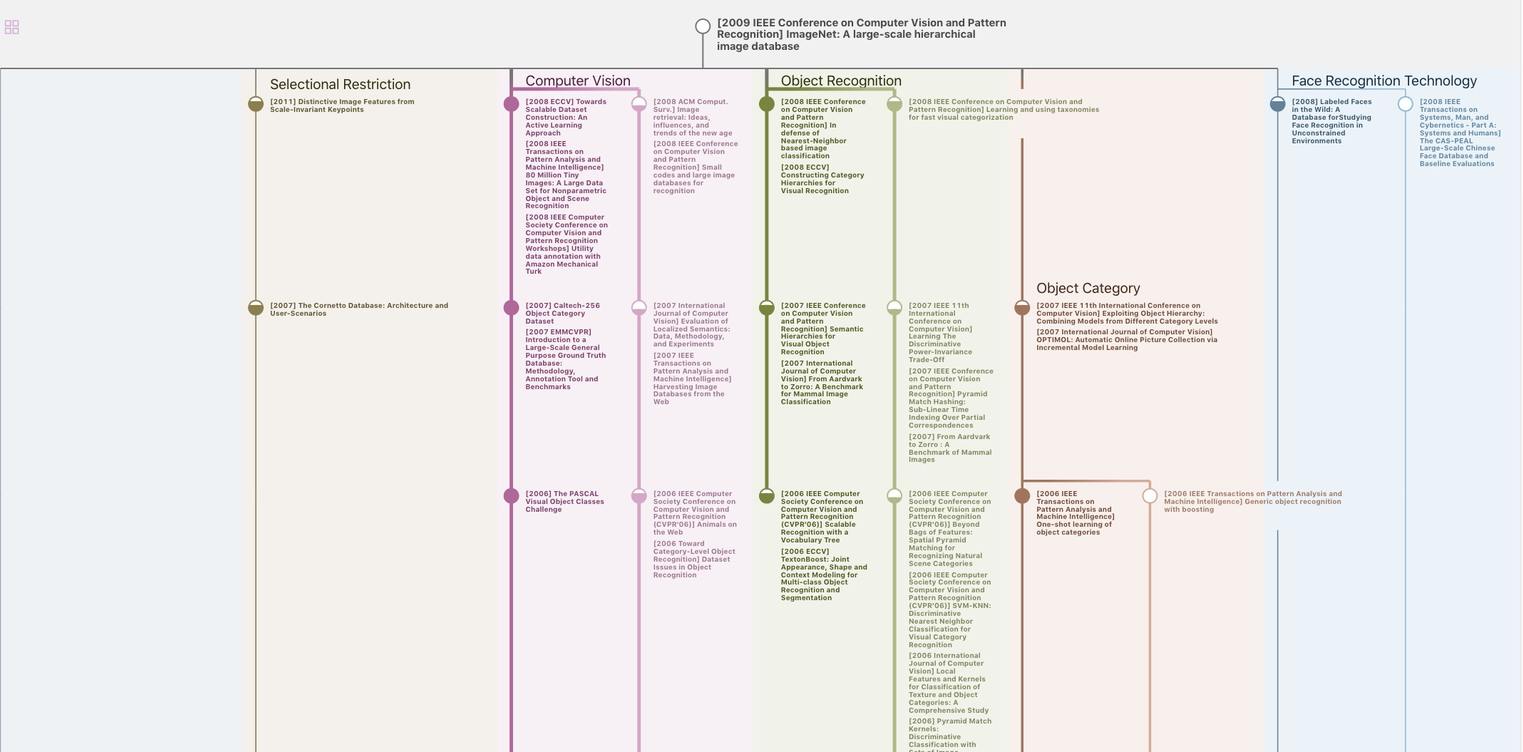
生成溯源树,研究论文发展脉络
Chat Paper
正在生成论文摘要