Detection of Advertising Users Based on K-SMOTE and Ensemble Learning.
HCC(2021)
摘要
Aiming at the problem of the unbalanced advertising user data of social networks leading to unsatisfactory prediction results, we propose a prediction model for advertising users based on the combination among K-Means, synthetic minority oversampling Technique (SMOTE), and Ensemble Learning. On the basis of the real user data provided by Scholat, we analyzed the data and extracted many key features from it to draw a portrait of advertising users. Our algorithm first clusters the minority class, and then processes the continuous and discrete features of each sample separately through the improved SMOTE to synthesize new minority samples, and finally constructs an integrated classifier using the ensemble learning. This method effectively avoids the problems of blurred positive and negative class boundaries caused by SMOTE and the inability of SMOTE to process discrete features. Meanwhile, ensemble learning enables the classifier to get more reasonable results and reduce overall errors. The experimental results showthat our method improves the quality of the generated minority class samples and significantly improves the prediction performance of advertising users.
更多查看译文
关键词
advertising users,detection,k-smote
AI 理解论文
溯源树
样例
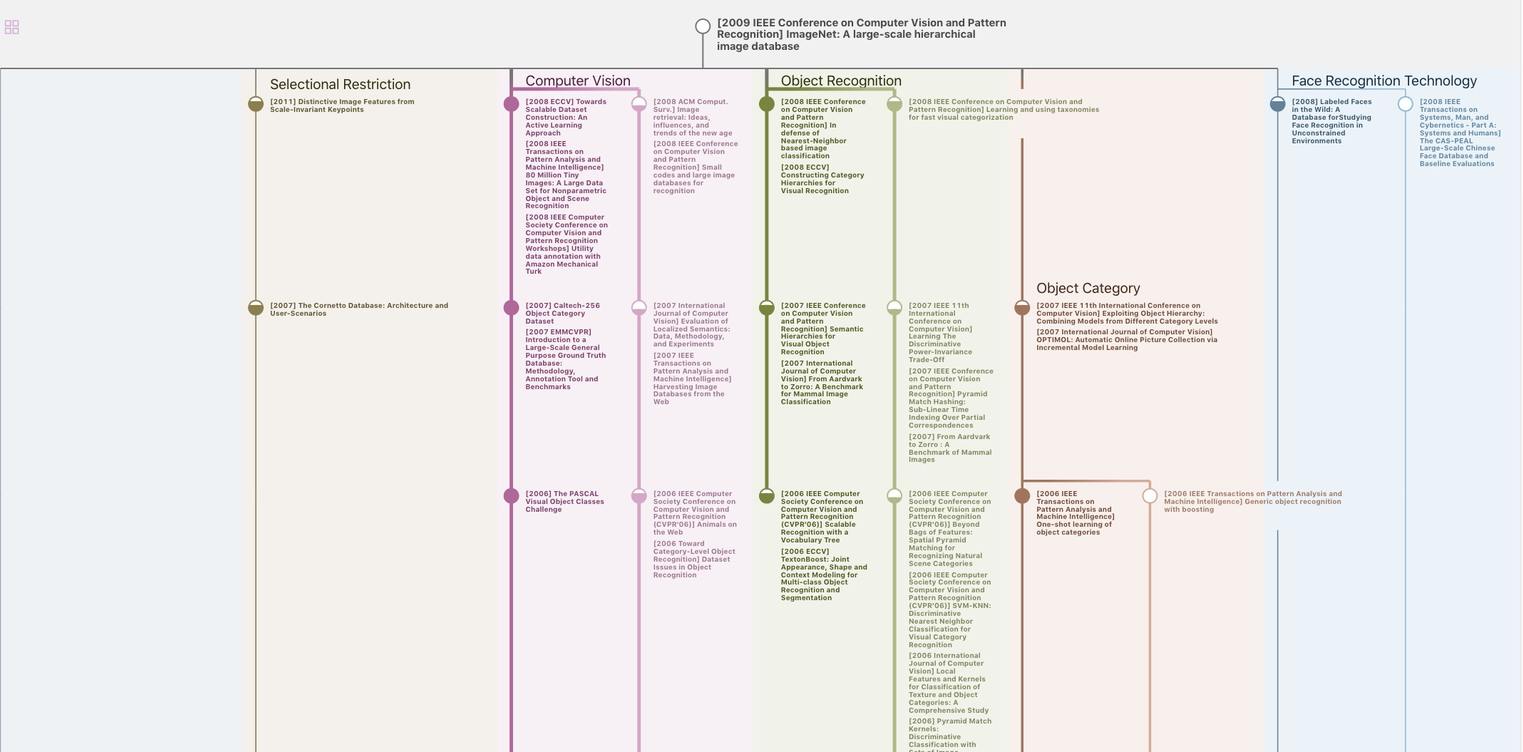
生成溯源树,研究论文发展脉络
Chat Paper
正在生成论文摘要