APHYN-EP: Physics-Based Deep Learning Framework to Learn and Forecast Cardiac Electrophysiology Dynamics.
STACOM@MICCAI(2022)
摘要
Biophysically detailed mathematical modeling of cardiac electrophysiology is often computationally demanding, for example, when solving problems for various patient pathological conditions. Furthermore, it is still difficult to reduce the discrepancy between the output of idealized mathematical models and clinical measurements, which are usually noisy. In this paper, we propose a fast physics-based deep learning framework to learn cardiac electrophysiology dynamics from data. This novel framework has two components, decomposing the dynamics into a physical term and a data-driven term, respectively. This construction allows the framework to learn from data of different complexity. Using 0D in silico data, we demonstrate that this framework can reproduce the complex dynamics of transmembrane potential even in presence of noise in the data. Additionally, using ex vivo 0D optical mapping data of action potential, we show the ability of our framework to identify the relevant physical parameters for different heart regions.
更多查看译文
关键词
deep learning framework,deep learning,cardiac,dynamics,physics-based
AI 理解论文
溯源树
样例
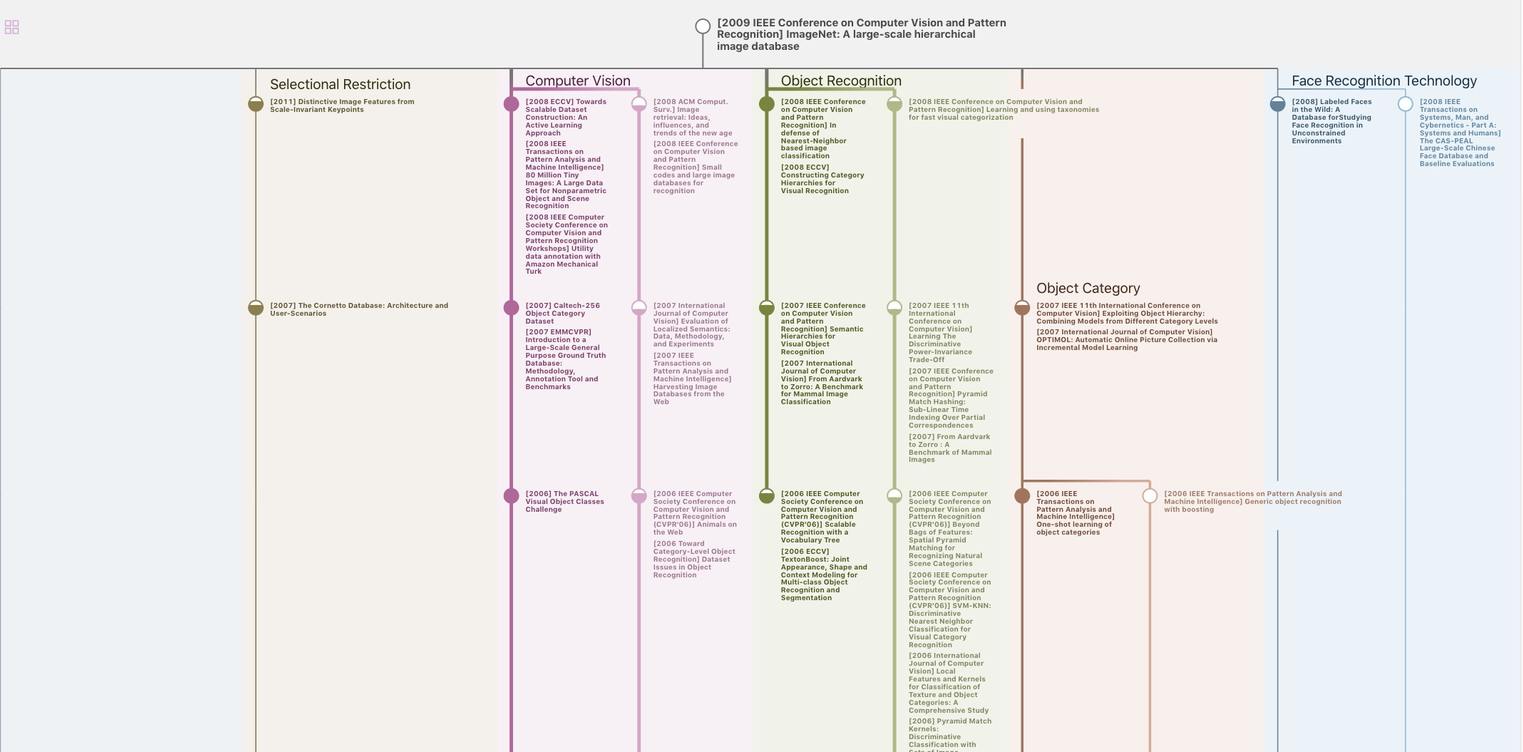
生成溯源树,研究论文发展脉络
Chat Paper
正在生成论文摘要