Explicit or Implicit? On Feature Engineering for ML-based Variability-intensive Systems.
VaMoS(2023)
摘要
Software variability engineering benefits from Machine Learning (ML) to learn e.g., variability-aware performance models, explore variants of interest and minimize their energy impact. As the number of applications of combining variability with ML grows, we would like to reflect on what is the core to the configuration process in software variability and inference in ML: feature engineering. These disciplines previously managed features explicitly, easing graceful combinations. Now, deep learning techniques derive automatically obscure but efficient features from data. Shall we give up explicit feature management in variability-intensive systems to embrace machine learning advances?
更多查看译文
关键词
feature, machine learning, software variability
AI 理解论文
溯源树
样例
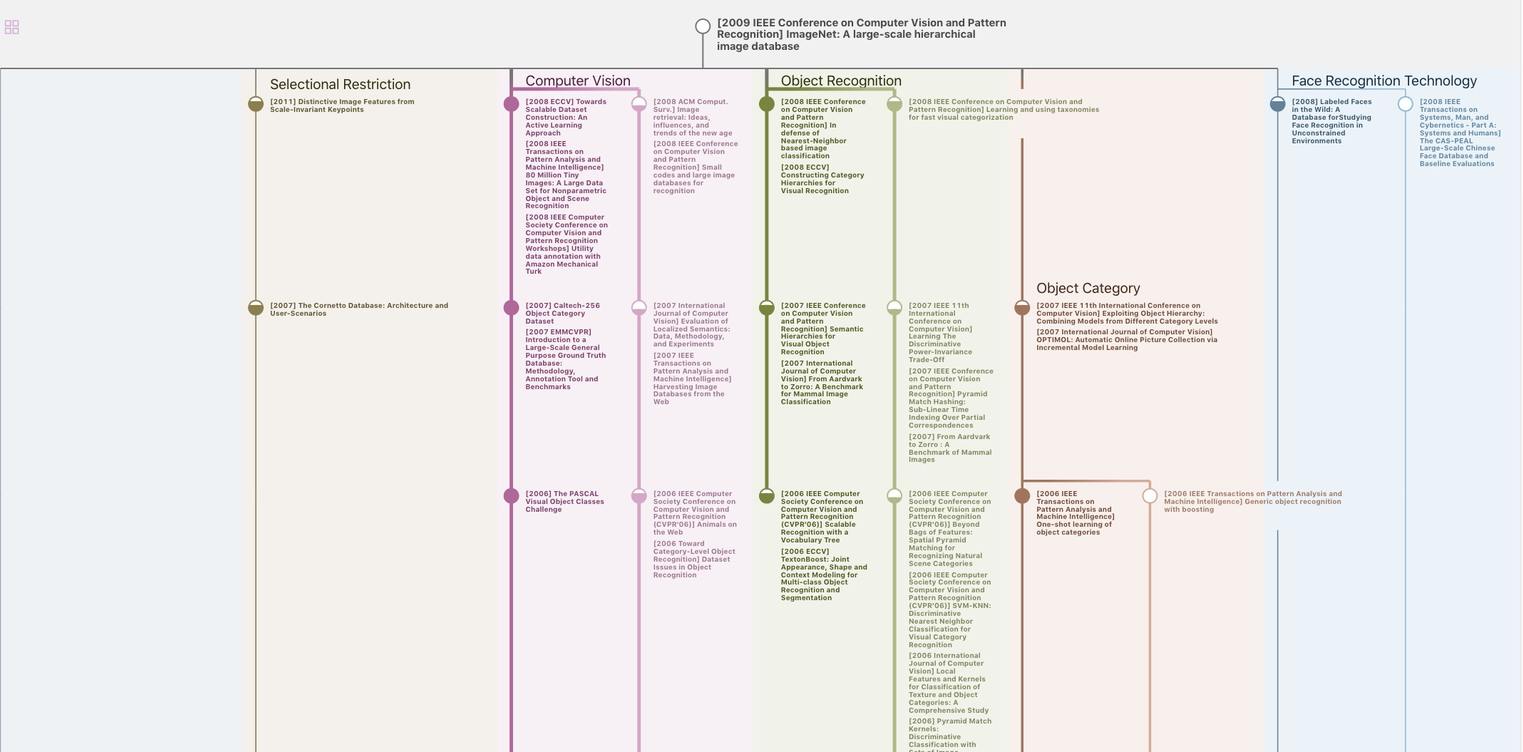
生成溯源树,研究论文发展脉络
Chat Paper
正在生成论文摘要