Invariant Factor Graph Neural Networks.
ICDM(2022)
摘要
Graph neural networks (GNNs) have achieved significant success in numerous fields under settings where training and testing graphs are identically distributed. However, this setting is rarely satisfied in real life. Due to the lack of outof-distribution (OOD) generalization abilities, existing GNNs methods perform disappointingly when there exist distribution shifts between testing and training graphs. Though several attempts have been made to deal with the issue, they mainly focus on structural properties while overlooking rich graph feature information. To this end, we propose an Invariant Factor GNN (IFGNN), which utilizes causal factor graphs to achieve invariant performances across different environments. Specifically, we dissect the graph generalization problem in a causal view, and argue that the key of graph generalization lies in discovering causal factors. Thus we extract the latent factors in the graph through disentanglement, and the causal ones are discovered with the invariant learning mechanism. We conduct extensive experiments on both synthetic and real-world datasets with distribution shifts to validate the OOD generalization abilities. The results demonstrate that our proposed IFGNN significantly outperforms the state-of-the-art baselines.
更多查看译文
关键词
graph neural networks,out-of-distribution generalization,invariant learning,disentanglement
AI 理解论文
溯源树
样例
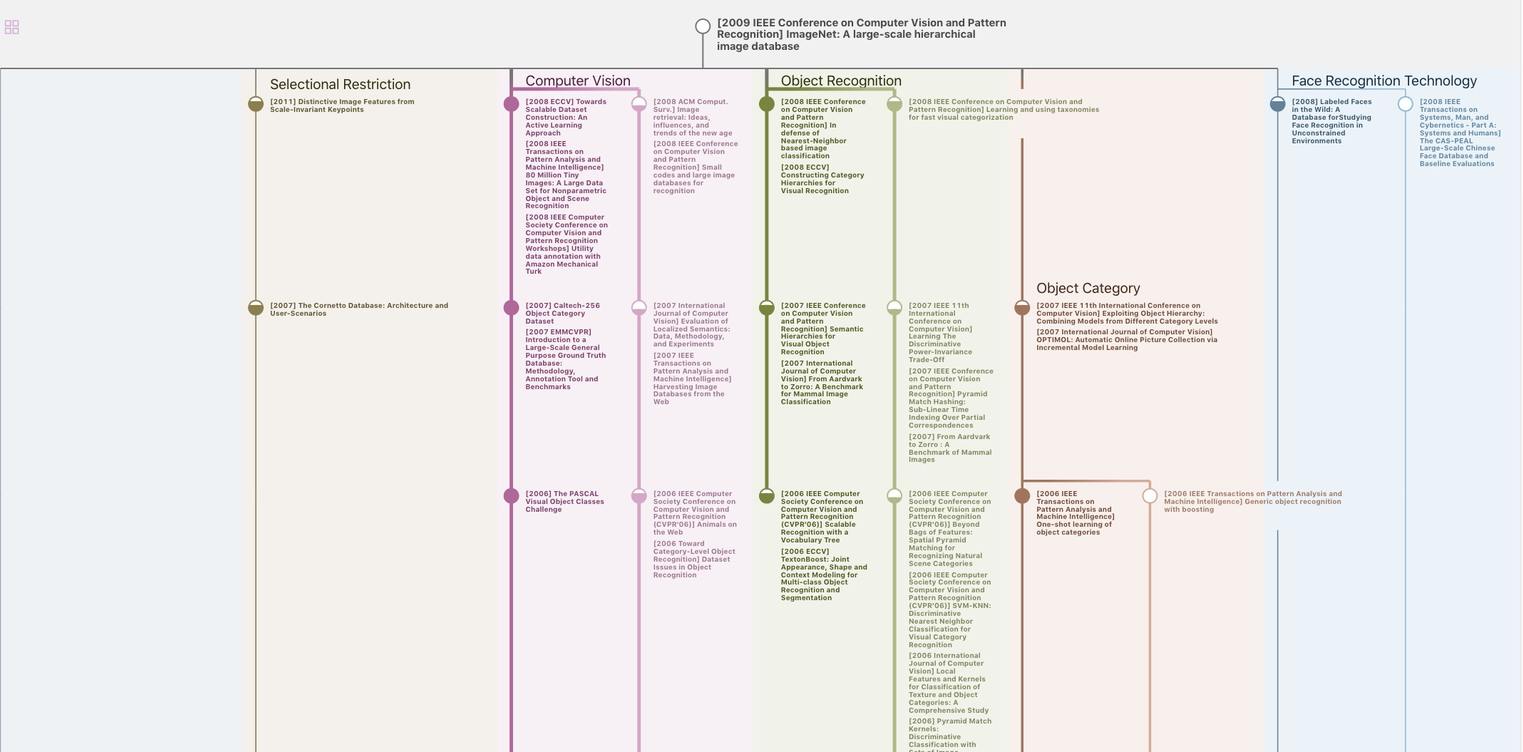
生成溯源树,研究论文发展脉络
Chat Paper
正在生成论文摘要