What Do Audio Transformers Hear? Probing Their Representations For Language Delivery & Structure.
ICDM (Workshops)(2022)
摘要
Transformer models across multiple domains such as natural language processing and speech form an unavoidable part of the tech stack of practitioners and researchers alike. Audio transformers that exploit representational learning to train on unlabeled speech have recently been used for tasks from speaker verification to discourse-coherence with much success. However, little is known about what these models learn and represent in the high-dimensional latent space. In this paper, we interpret two such recent state-of-the-art models, wav2vec2.0 and Mockingjay, on linguistic and acoustic features. We probe each of their layers to understand what it is learning and at the same time, we draw a distinction between the two models. By comparing their performance across a wide variety of settings including native, non-native, read and spontaneous speeches, we also show how much these models are able to learn transferable features. Our results show that the models are capable of significantly capturing a wide range of characteristics such as audio, fluency, suprasegmental pronunciation, and even syntactic and semantic text-based characteristics. For each category of characteristics, we identify a learning pattern for each framework and conclude which model and which layer of that model is better for a specific category of feature to choose for feature extraction for downstream tasks.
更多查看译文
关键词
audio transformers,language delivery,representations
AI 理解论文
溯源树
样例
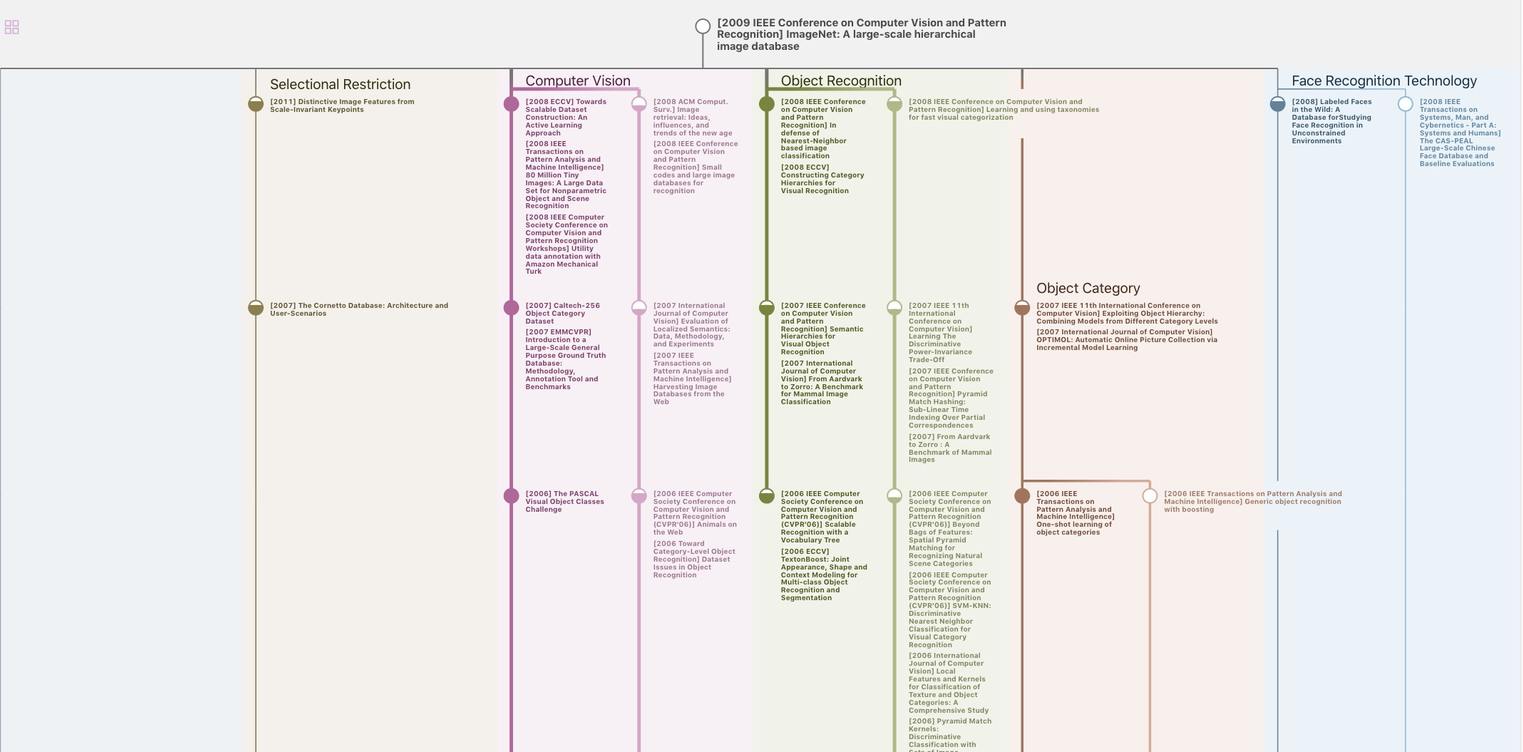
生成溯源树,研究论文发展脉络
Chat Paper
正在生成论文摘要