Deep-Reinforcement-Learning-Based Doppler Compensation for High-Mobility Cell-Free Massive MIMO Systems.
WCSP(2022)
摘要
In this paper, we consider a cell-free (CF) massive multiple-input multiple-output (MIMO) system in high-mobility scenario with multiple access points (APs) serving a high-speed railway (HSR). The signal transmits through multiple paths, where different Doppler frequency offsets (DFOs) are related with distinct angle of departures (AoDs) and hence resulting in fast time-varying of the channel in uplink transmission. To solve this problem, we propose a method for Doppler compensating in the angle domain based on Multi-agent Deep Deterministic Policy Gradient (MADDPG) framework. Specifically, we design a beamforming network using a large-scale uniform linear array (ULA) at both the transmitter and receiver, where different parallel beam branches separate different DFOs, which can be compensated accordingly before transmission starts. The simulation results indicate that our proposed scheme can achieve effective performance in Doppler compensation, and at the same time, it can achieve comparable performance with respect to exhaustive search with lower complexity.
更多查看译文
关键词
Cell-free massive MIMO,high-mobility,doppler compensation,beamforming,multi-agent reinforcement learning
AI 理解论文
溯源树
样例
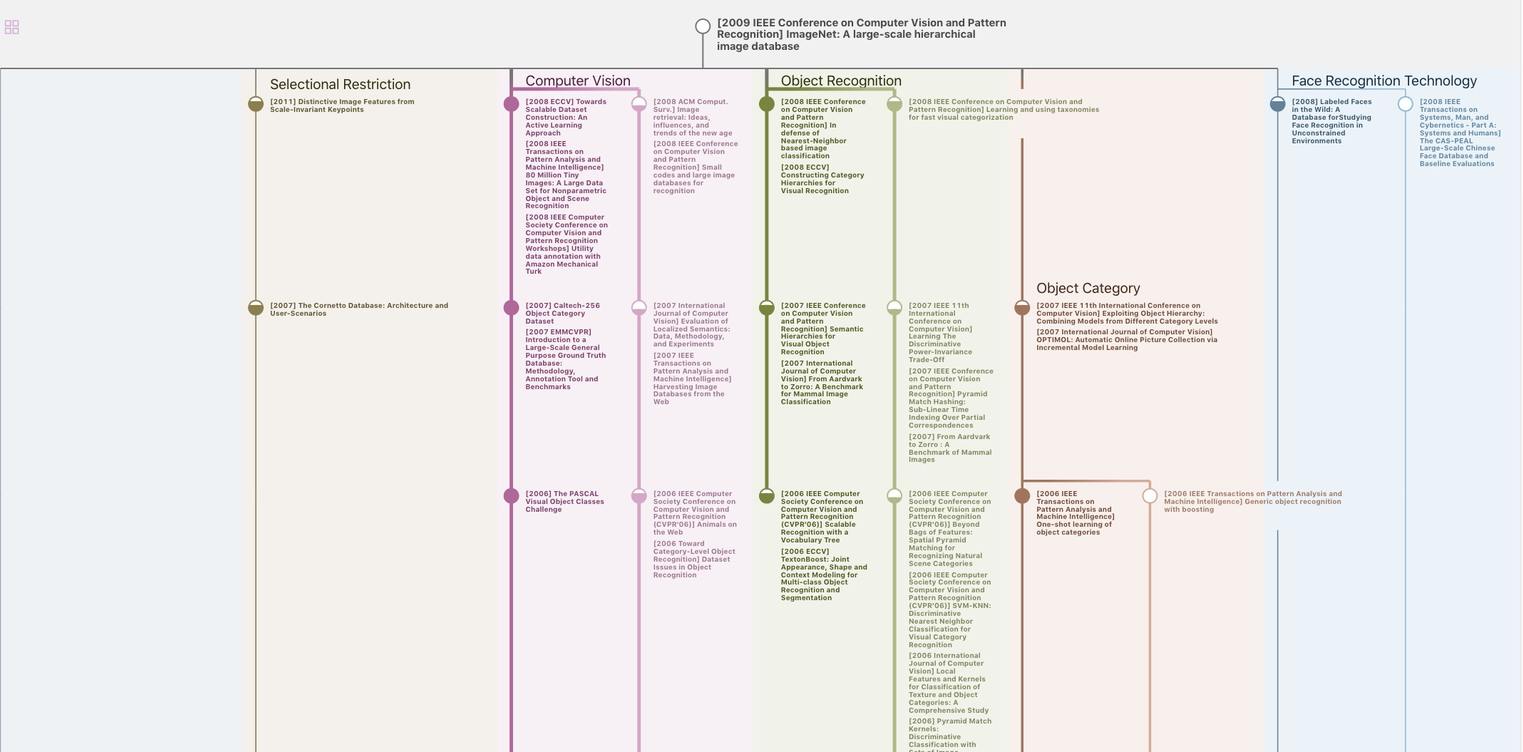
生成溯源树,研究论文发展脉络
Chat Paper
正在生成论文摘要