Unfair AI: It Isn't Just Biased Data.
ICDM(2022)
摘要
Conventional wisdom holds that discrimination in machine learning is a result of historical discrimination: biased training data leads to biased models. We show that the reality is more nuanced; machine learning can be expected to induce types of bias not found in the training data. In particular, if different groups have different optimal models, and the optimal model for one group has higher accuracy, the optimal accuracy joint model will induce disparate impact even when the training data does not display disparate impact. We argue that due to systemic bias, this is a likely situation, and simply ensuring training data appears unbiased is insufficient to ensure fair machine learning.
更多查看译文
关键词
Machine Learning, Fairness, Systemic Bias
AI 理解论文
溯源树
样例
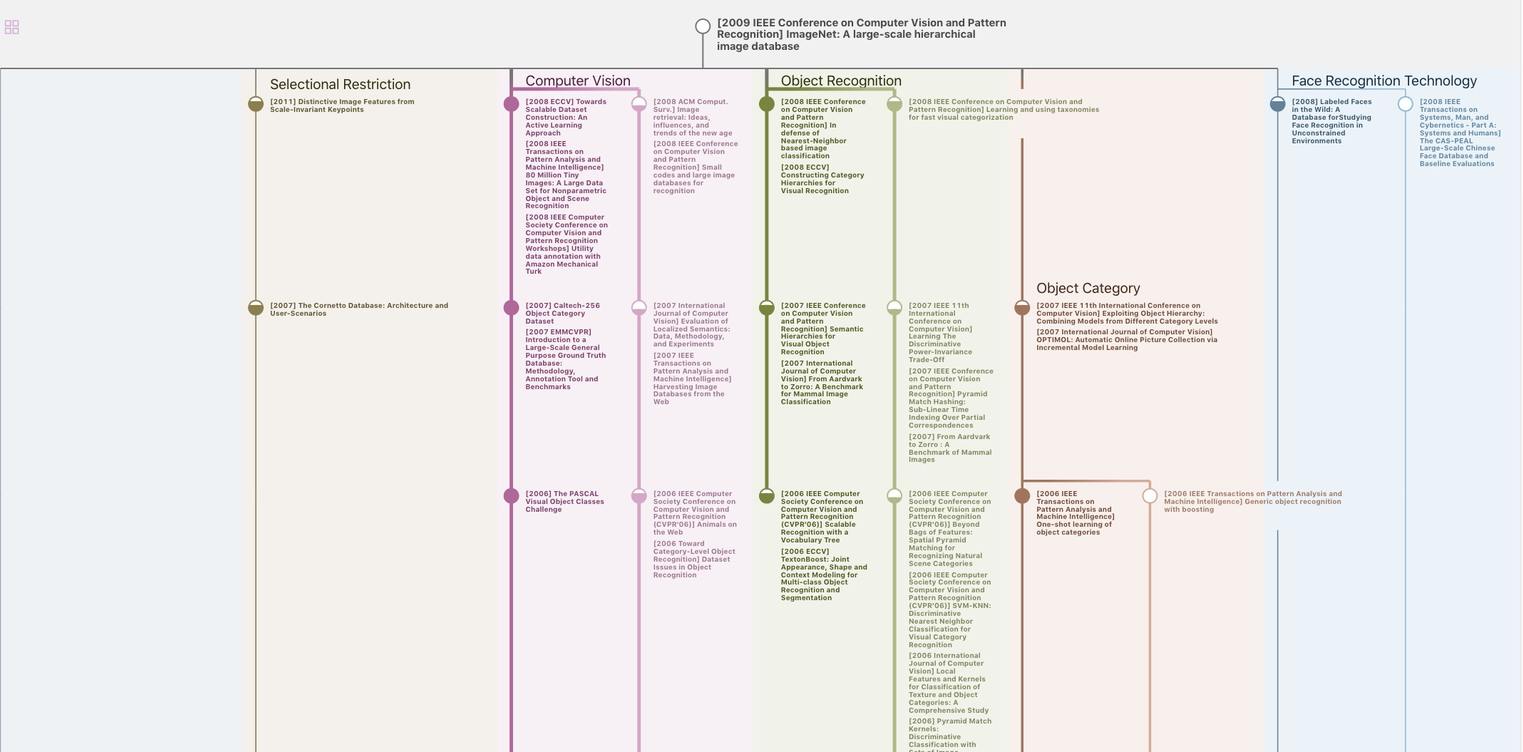
生成溯源树,研究论文发展脉络
Chat Paper
正在生成论文摘要