Poster: Towards NLOS Ranging Error Detection and Mitigation using Machine Learning on Embedded Ultra-Wideband Devices.
EWSN(2022)
摘要
We study the ranging error classification and mitigation capabilities of machine learning models used in ultrawideband systems. This is relevant, as distance estimates in non-line-of-sight (NLOS) conditions can be off by several meters, which may severely compromise the performance of applications that require location awareness. Our ultimate goal is to optimize the size of a convolutional neural network (CNN) used for classifying and mitigating ranging errors such that it can run on constrained embedded devices without affecting its performance. To this end, we present an optimized CNN implementation that, in contrast to resourcehungry machine learning models requiring hundreds of kB of memory, can classify and mitigate NLOS conditions with 12 kB of RAM and 75 kB of ROM.
更多查看译文
AI 理解论文
溯源树
样例
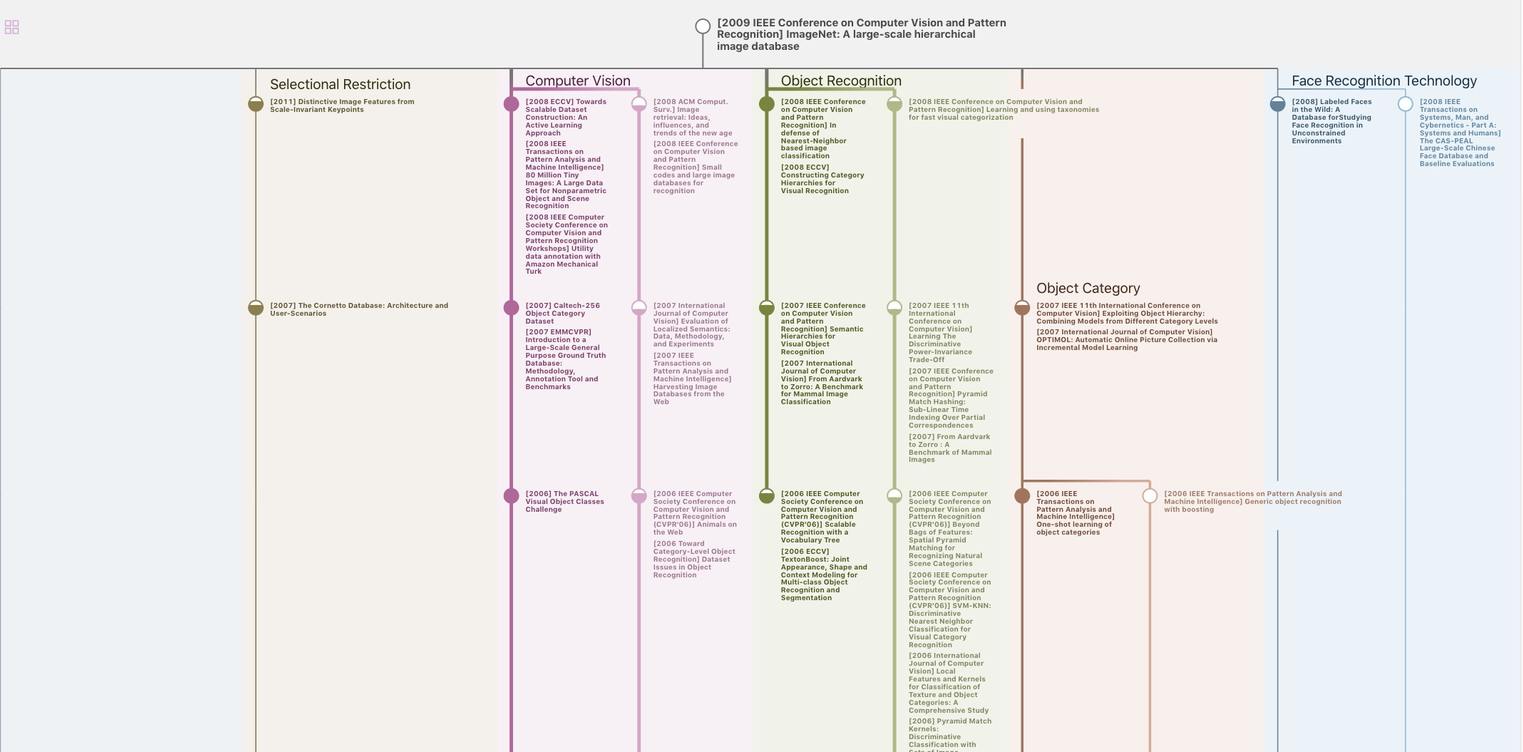
生成溯源树,研究论文发展脉络
Chat Paper
正在生成论文摘要