An Empirical Study on Characterizing Natural Disasters in Class Imbalanced Social Media Data using Weak Supervision.
Big Data(2022)
摘要
Supervised learning has proven to be successful in classifying both class balanced and imbalanced data when a strong supervision signal is available. However, generating the supervision signal (eg: ground truth labels) is expensive and a major bottleneck of supervised learning. To curtail this, we rely on the theory of noisy learning and weak supervision to generate supervision signals. In this work, we utilize a noisy labeled dataset to train several class balanced and imbalanced machine learning models and compare the results to observe how efficient the models trained on silver standard dataset are in identifying ground truth labels. We demonstrate the approach on a natural disasters application which contains data from three different natural disasters. Our results demonstrate that theory of noisy learning can be utilized to build models via weak supervision for both class balanced and imbalanced data from social media sources for natural disasters application.
更多查看译文
关键词
Weak supervision,Large scale data analysis,Machine learning,Social media data
AI 理解论文
溯源树
样例
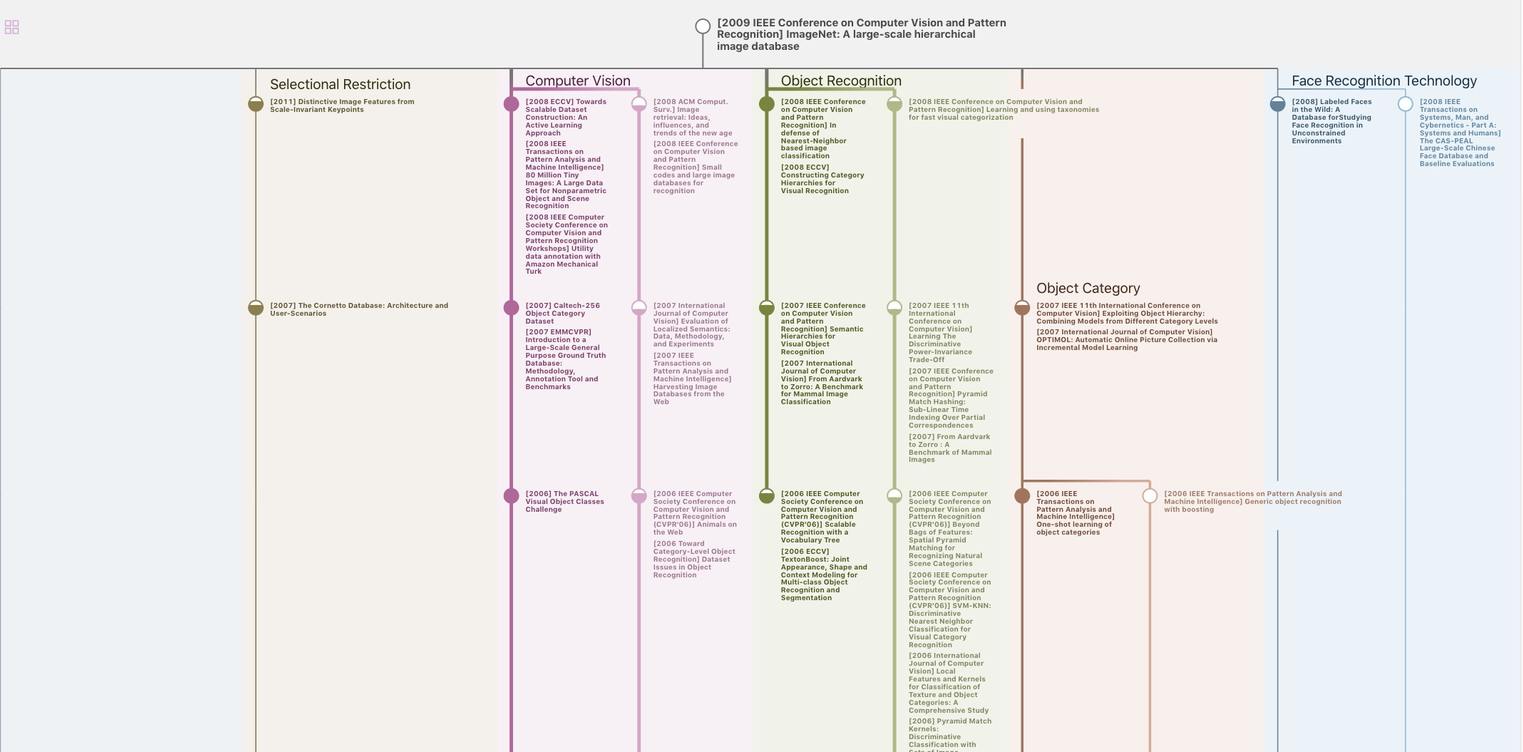
生成溯源树,研究论文发展脉络
Chat Paper
正在生成论文摘要