Collaborative Filtering Guided Deep Reinforcement Learning for Sequential Recommendations
Big Data(2022)
摘要
Earlier recommendation techniques, such as Collaborative Filtering (CF), assume the users’ preferences do not change over time and strive to maximize the immediate reward. In recent studies, Reinforcement Learning (RL) has been used to make interactive recommendation systems that capture users’ preferences over time and maximize the long-term reward. However, these methods have two limitations. First, they assume that items are independently distributed and do not consider the relations between items. This assumption ignores the power of the relations between items for recommendation systems, as demonstrated by CF. RL-based methods rely primarily on users’ positive feedback to understand their preferences, and sampling is used to incorporate their negative feedback. In a practical setting, users’ negative feedback is just as crucial as their positive feedback for understanding their preferences. We present a novel Deep Reinforcement Learning (DRL) recommendation framework to address the limitations above. We specifically utilize the actor-critic paradigm, which considers the recommendation problem a sequential decision-making process to adapt to users’ behaviors and maximize the long-term reward. Motivated by the intuition that similar users like similar items, we extract the relations between items using CF and integrate it into our framework to boost overall performance. Instead of negative sampling, our proposed framework relies on all users’ positive and negative feedback to understand users’ preferences more accurately. Extensive experiments with our dataset and two public datasets demonstrate the effectiveness of our proposed framework.
更多查看译文
关键词
Deep Reinforcement Learning,Actor-Critic Framework,Collaborative Filtering,Negative Feedback,Interactive Recommendation Systems
AI 理解论文
溯源树
样例
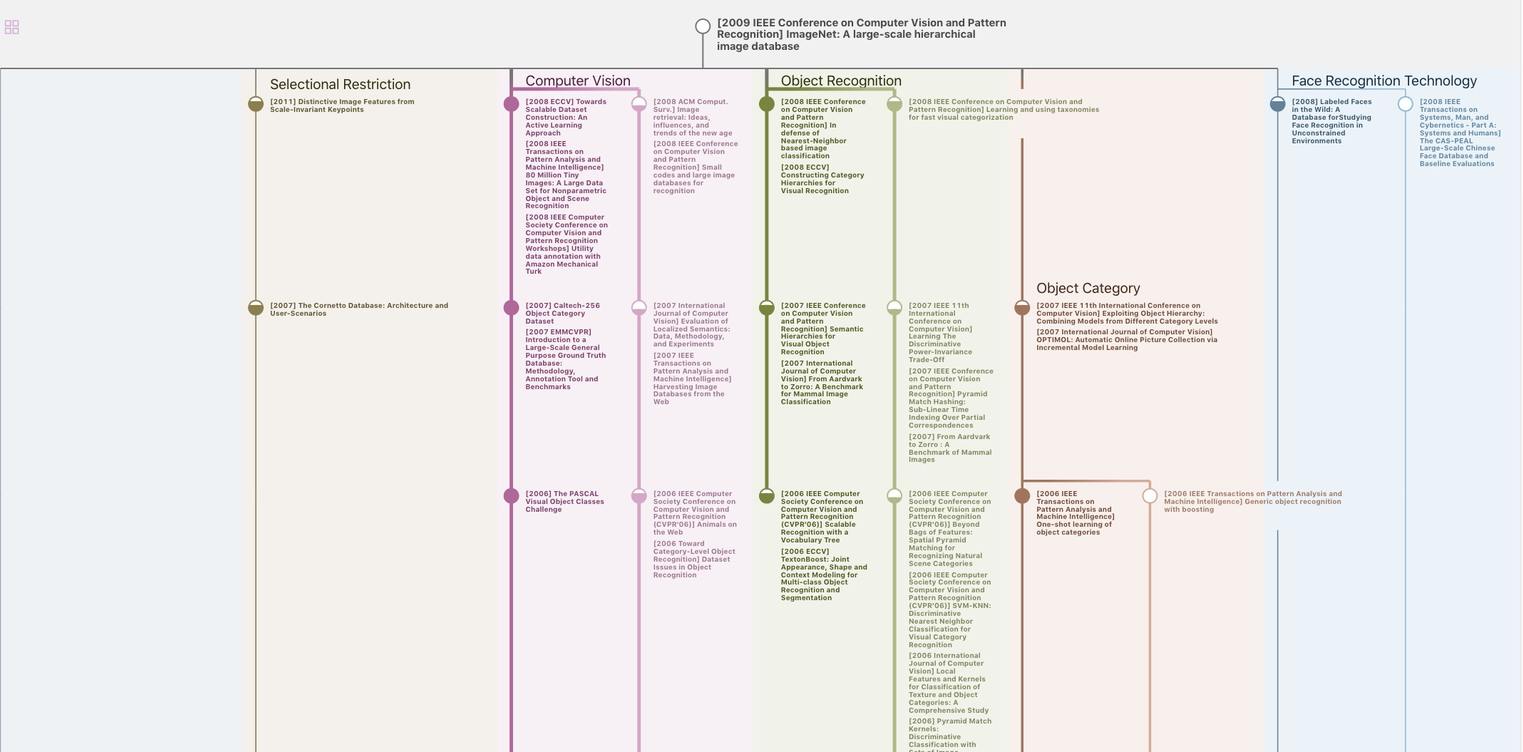
生成溯源树,研究论文发展脉络
Chat Paper
正在生成论文摘要