Deep Time Series Sketching and Its Application on Industrial Time Series Clustering.
Big Data(2022)
摘要
Today, voluminous multivariate time series data collected from sensors provides tremendous benefit for understanding of modern industrial systems such as power plants, wind turbines and aircrafts. However, the dynamic and complex nature of these systems, as well as the lack of prior knowledge impose challenges in perceiving different system behaviors from the time series data. To handle these issues, time series clustering has become one of the key analysis techniques. Nevertheless, the data nonlinearity, varying lengths and high dimensions of industrial time series could hinder the quality of clustering. To deal with these challenges, we propose Deep Time Series Sketching (DTSS) model. This model is a representation learning model based on temporal convolutional networks that perform on a sliding window basis along time series to learn the windows’ embeddings. The sequence of embeddings is then fed into embedding sketching to obtain its sketch. Such sketch is a descriptor of the whole time series and will be fed into K-means for clustering. Our model is a novel end-to-end hybrid model that incorporates both local and global contextual features. It is able to project multivariate time series with varying lengths into the same latent space. Moreover, we show that our model is able to perform early clustering as it can assign real-time label without seeing the whole time series. We test our model on both benchmark and real world industrial datasets, and experiments show that our proposed method outperforms popular time series clustering baselines.
更多查看译文
关键词
deep time series sketching,industrial
AI 理解论文
溯源树
样例
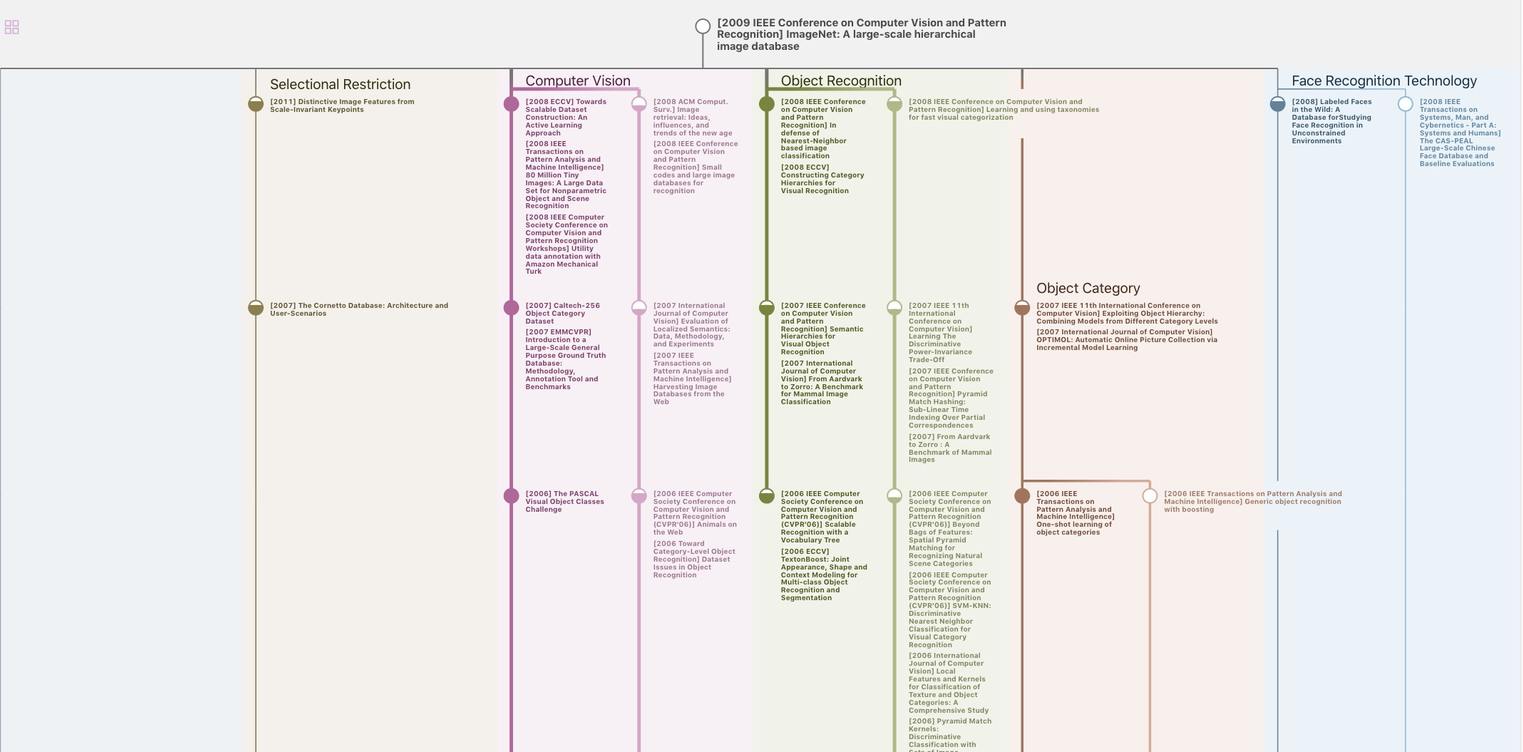
生成溯源树,研究论文发展脉络
Chat Paper
正在生成论文摘要