Debiased Learning of Self-Labeled Twitter Data for User Demographic Prediction.
Big Data(2022)
摘要
Labeling sufficient data for supervised learning remains an open challenge in social network analysis. An alternative is to collect self-labeled data, i.e. the data labeled by their owners. Emmery et al show that standard models can be trained and perform well on self-labeled data, suggesting the effectiveness of this approach. In this paper, we argue self-labeled data may not be representative of the population. Taking Twitter demographic prediction as an example, we show the popular FastText model standardly trained on self-labeled data does not generalize well on random testing samples. We then present a new learner DeFastText that aims to correct data bias using the kernel means matching technique. In experiment, we show it achieves lower generalization errors than FastText. This research raises an attention of the data bias problem when learning from self-labeled data in social network analysis.
更多查看译文
关键词
self-labeled data,demographic prediction,fast-text,kernel means matching,concept drift
AI 理解论文
溯源树
样例
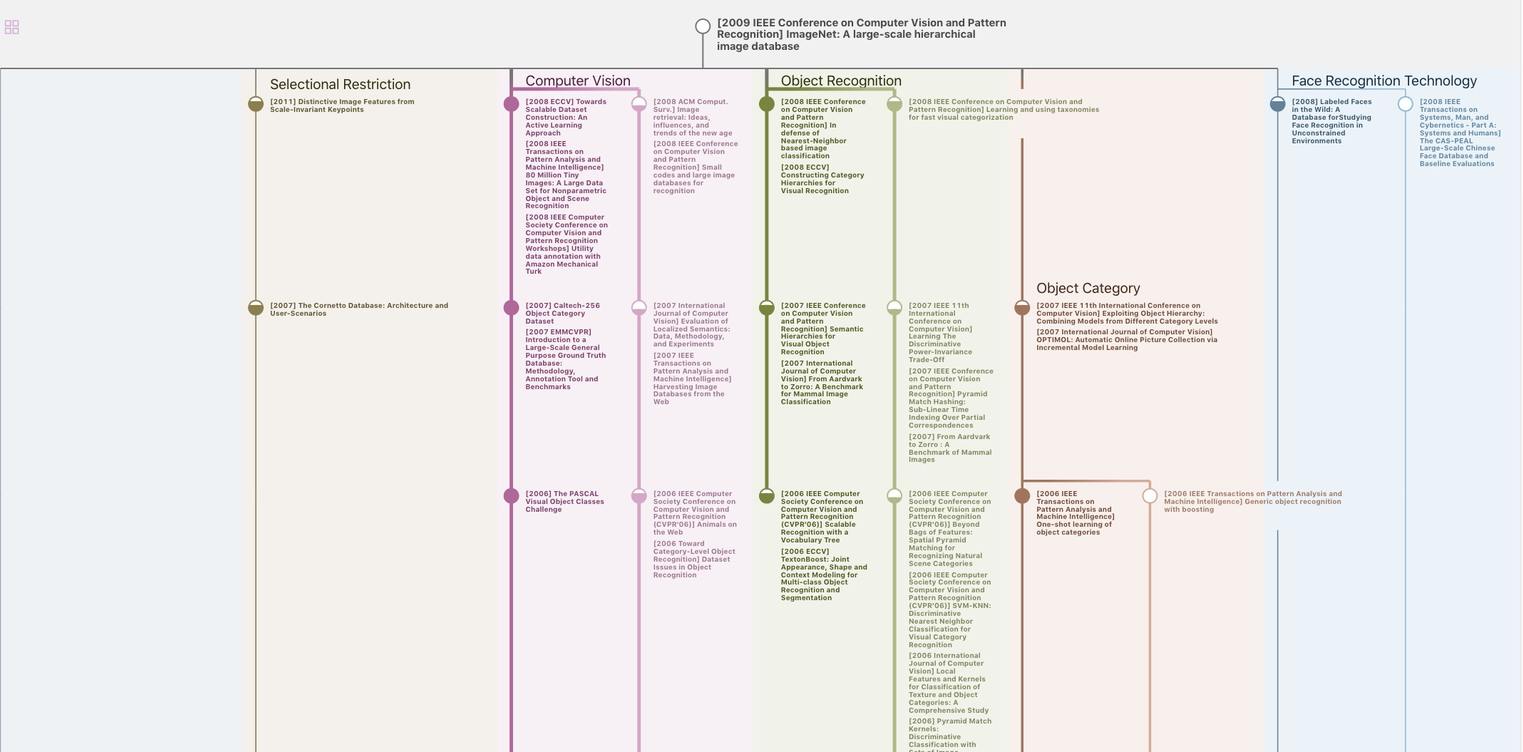
生成溯源树,研究论文发展脉络
Chat Paper
正在生成论文摘要