Selective ensemble learning for cross-muscle ALS disease identification with EMG signal.
BIBM(2022)
摘要
Electromyography (EMG) provides a useful way to identify amyotrophic lateral sclerosis(ALS) disease. EMG signals sampled from different muscles show different sensitivities on ALS disease identification, in which to find out the most sensitive muscles to ALS is meaningful. In this paper, a selective ensemble learning method is proposed for cross-muscle ALS disease identification. First, omics features are extracted from time, frequency and wavelet domains of the original EMG signals and their adaptively decomposed components respectively. Second, the ensemble learning method with selective voting strategy is proposed for ALS identification in cross-individual and cross-muscle scenarios. Finally, the contributions of each sample to the individual identification are comprehensively analyzed using the ridge regression model. Two EMG datasets from different human race and different devices are used to evaluate the performance of the proposed method. Experimental results illustrate the effectiveness of the proposed method on cross-individual and cross-muscle ALS identification, i.e. the classification accuracy and sensitivity improve by 1% ~ 11% and 1%. ~ 18% respectively.
更多查看译文
关键词
Electromyography,Amyotrophic lateral sclerosis,Ensemble learning,Omics feature extractionRidge regression
AI 理解论文
溯源树
样例
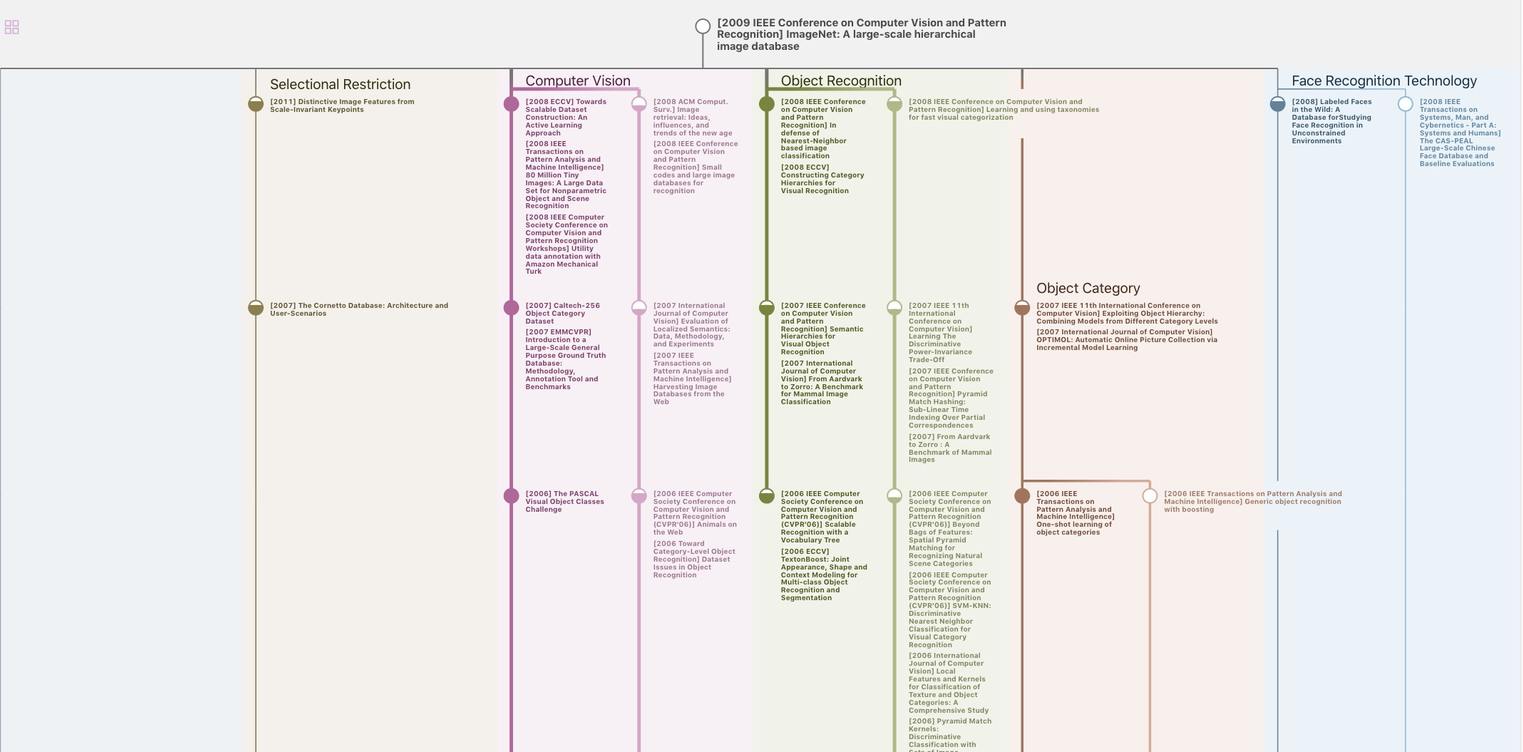
生成溯源树,研究论文发展脉络
Chat Paper
正在生成论文摘要