Identifying Variability in U.S. COVID-19 Response Through Temporal Partial Ordering Detection.
BIBM(2022)
摘要
We gain insight to the COVID-19 pandemic response by the various U.S. states through analysis of open source emergency declaration, mitigation, and response policy data. We propose ASNM + POD, a Partial Ordering Detection extension to the Adaptive Sorted Neighborhood Method to identify redundancies and implied temporal ordering requirements to understand how various U.S. states respond to COVID-19. We further strengthen the well-established ASNM entity matching method and address key limitations of its Longest Common Subsequence extension (ASNM + LCS) through detection of all temporal order requirements. Partial order requirements are determined probabilistically through empirical review of all records’ time-ordered event sequences. We demonstrate effectiveness against a COVID-19 U.S. state policy dataset comprised of daily time-series data pulled from February and October 2022, where attributes are partially and variably populated. ASNM + POD yielded an F1 of 0.995 and an MCC of 0.985, significantly outperforming both ASNM and ASNM + LCS with F1/MCC improvements of 22%/50% and 15%/37%, respectively. Finally, we highlight the limited consensus on policies enacted, the variability in timelines of policy activations/deactivations, and activity at and after the two-year mark.
更多查看译文
关键词
COVID-19,temporal data,record deduplication,record linkage,entity matching
AI 理解论文
溯源树
样例
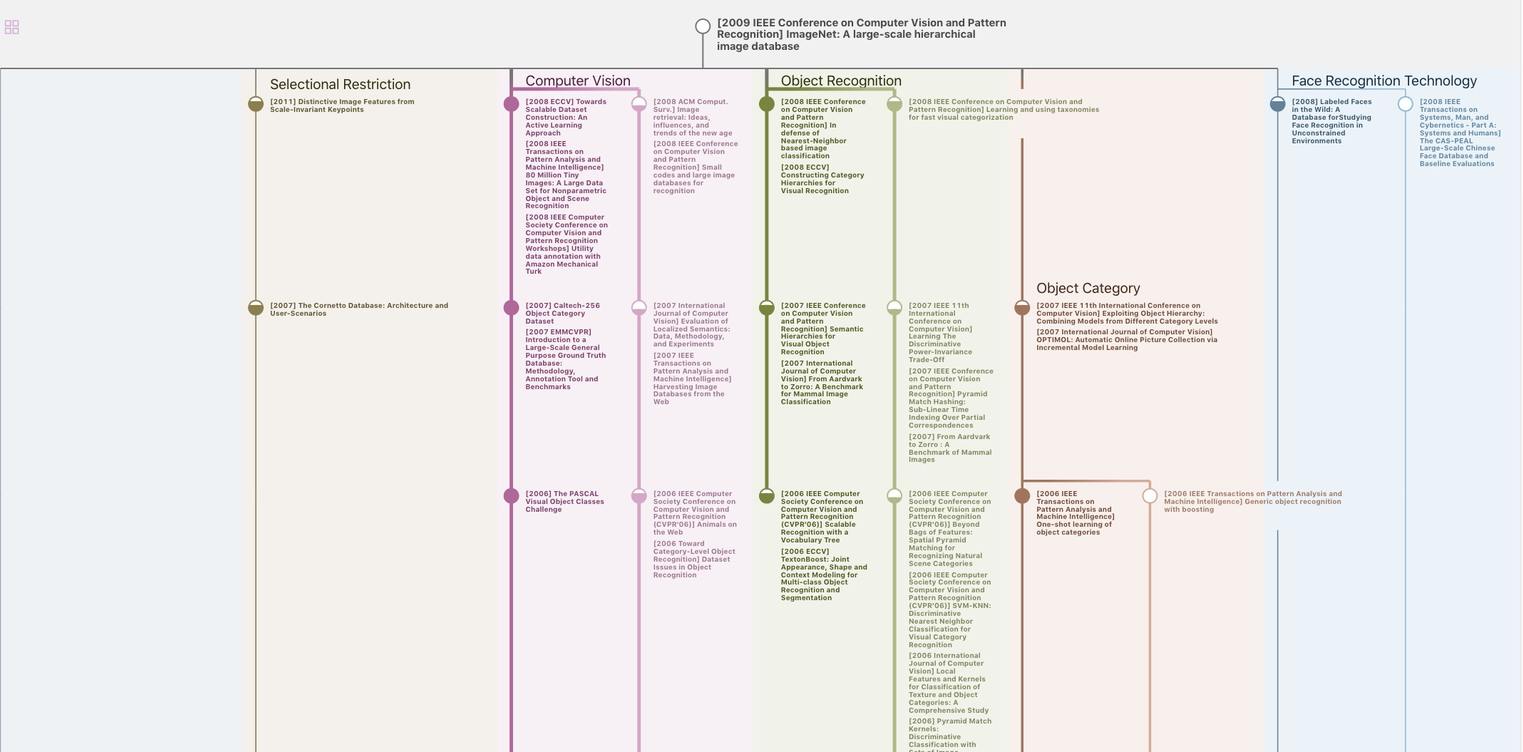
生成溯源树,研究论文发展脉络
Chat Paper
正在生成论文摘要