Learning to Solve a Stochastic Orienteering Problem with Time Windows.
LION(2022)
摘要
Reinforcement learning (RL) has seen increasing success at solving a variety of combinatorial optimization problems. These techniques have generally been applied to deterministic optimization problems with few side constraints, such as the traveling salesperson problem (TSP) or capacitated vehicle routing problem (CVRP). With this in mind, the recent IJCAI AI for TSP competition challenged participants to apply RL to a difficult routing problem involving optimization under uncertainty and time windows. We present the winning submission to the challenge, which uses the policy optimization with multiple optima (POMO) approach combined with efficient active search and Monte Carlo roll-outs. We present experimental results showing that our proposed approach outperforms the second place approach by 1.7%. Furthermore, our computational results suggest that solving more realistic routing problems may not be as difficult as previously thought.
更多查看译文
关键词
stochastic orienteering problem,learning
AI 理解论文
溯源树
样例
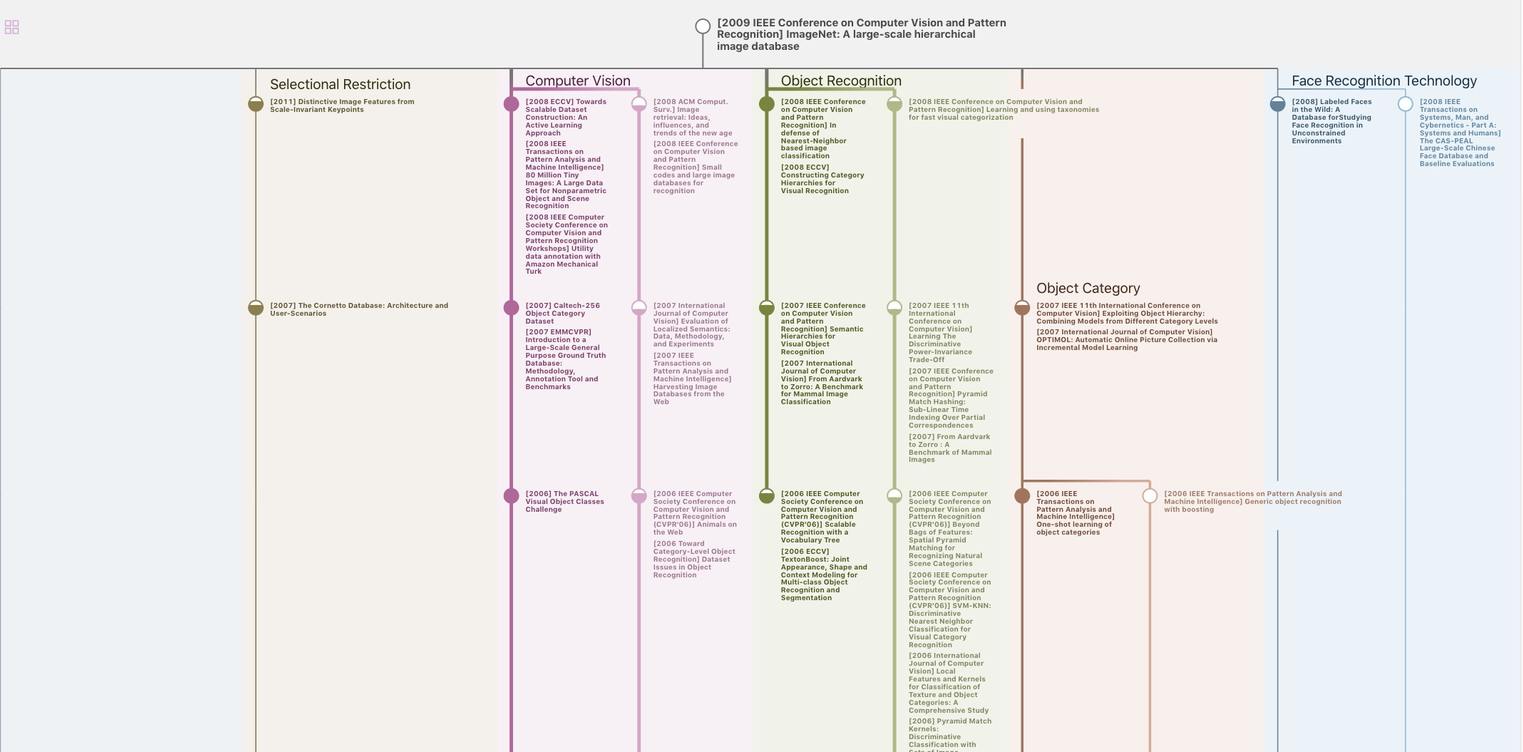
生成溯源树,研究论文发展脉络
Chat Paper
正在生成论文摘要