A Global-Local Cascade Network for Multi-bone Segmentation in Chest CT.
BIBM(2022)
摘要
Chest multi-bone segmentation plays an important role in clinical applications such as metastasis analysis and surgical planning. The global context between bones is critical for accurately identifying bone sub-classes; meanwhile, the influence of local details on bone segmentation accuracy cannot be ignored. However, the global context and local details are challenging to trade off under hardware and computation cost limitations. In this study, we propose Bone-Net that simultaneously leverages global context and local details by employing a two-stage cascaded framework for multi-bone segmentation in chest CT. In the first stage, we feed low-resolution large-scale images to bidirectional feature pyramid network to extract global context and perform multi-bone category prediction. In the second stage, we propose a global-guided gating module to link global context and local details to refine bone segmentation. During inference, we obtained the multi-bone segmentation results by rendering the category information into the segmentation results. Furthermore, we design a novel loss function to alleviate the category confusion problem for adjacent bones. The proposed method is trained and evaluated on a challenging dataset of 250 chest CT scans from various centers and scanners. Experimental results show that the proposed Bone-Net achieves high performance (84.1% of dice similarity coefficient, 87.7% of recall, and 80.7% of precision) and outperforms five benchmarks for multi-bone segmentation.
更多查看译文
关键词
chest,ct,global-local,multi-bone
AI 理解论文
溯源树
样例
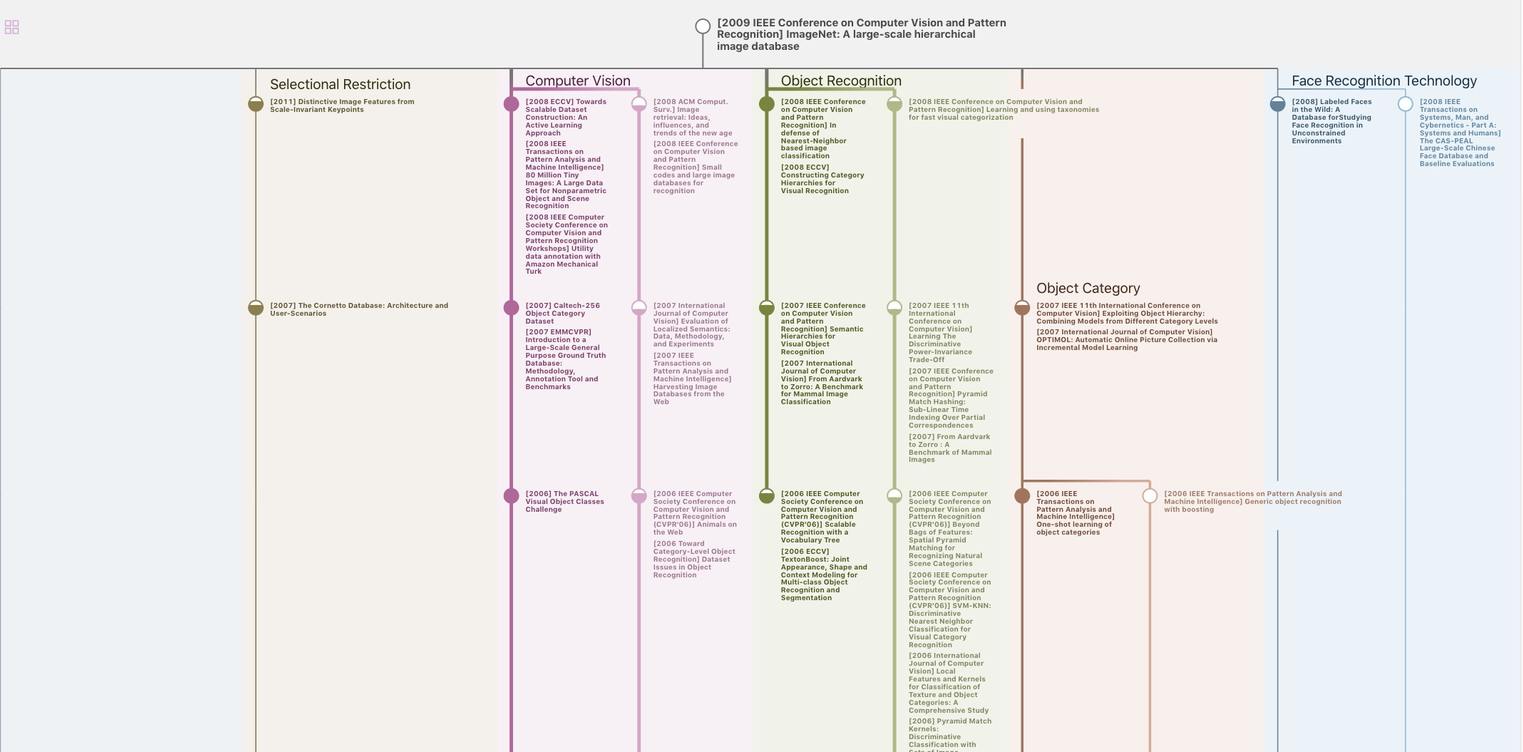
生成溯源树,研究论文发展脉络
Chat Paper
正在生成论文摘要