MVSF: Multi-View Signature Fusion Network for Noninvasively Predicting Ki67 Status.
BIBM(2022)
摘要
Ki67 is a promising molecular biomarker for the diagnosis of lung adenocarcinoma. However, previous methods to determine Ki67 status often require tumor tissue sampling, which is invasive for patients. This study proposes a multi-view signature fusion network (MVSF), combining deep learning encoded (DLE) signatures, handcrafted radiomics (HCR) signatures, and clinical information to noninvasively predict Ki67 status. Multi-view signatures are combined through a tensor fusion network to obtain potentially high-dimensional signatures. Finally, a cooperative game theory-based approach is applied to quantitatively interpret the contribution of signatures to decision-making. The proposed MVSF is evaluated on a retrospectively collected dataset of 661 patients. Experimental results show that the MVSF achieves encouraging performance, with an area under the receiver operating characteristic curve of 0.80 and an accuracy of 0.78, outperforming several state-of-the-art Ki67 status prediction methods, which implies that our proposed method could provide potential support for Ki67 status prediction.
更多查看译文
关键词
Lung adenocarcinoma,Ki67 status prediction,Multi-view signatures,Tensor fusion,Signature interpretability
AI 理解论文
溯源树
样例
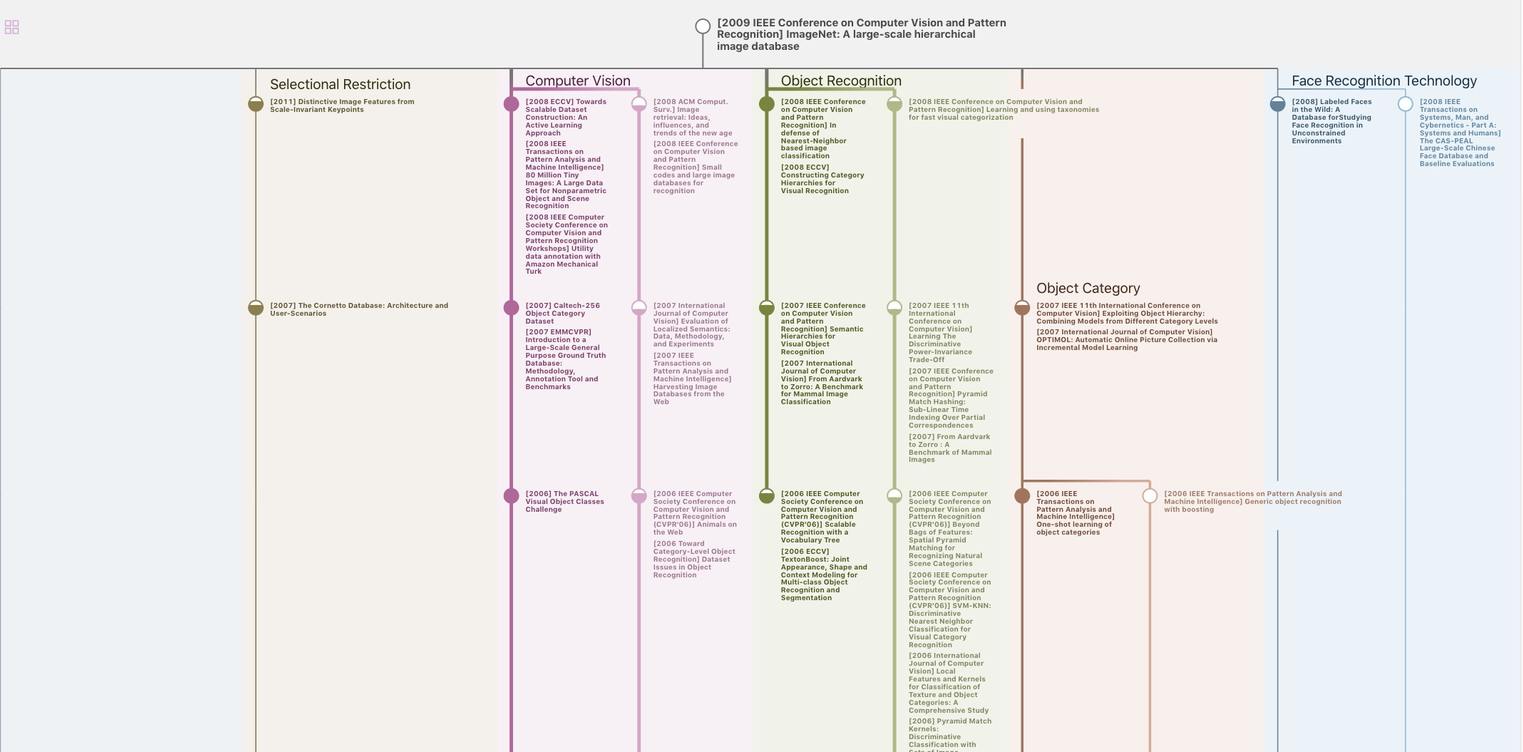
生成溯源树,研究论文发展脉络
Chat Paper
正在生成论文摘要