Online Personalisation of Deep Mobile Activity Recognisers.
iWOAR(2022)
摘要
Activity recognition is an increasingly important feature in mobile consumer devices that enables the design of context-aware applications and the customisation of user experiences. Recent deep learning-based recognisers demonstrate promising performances for hand activity recognition -e.g., washing hands-or human activity recognition -e.g., cycling-when exploiting accelerometer data from smartwatches or Magnetic Angular Rate and Gravity (MARG) data from body-worn sensors. However, the robustness and the generalisation capabilities of deep learning models can be an issue, since the consumers' locomotion data is typically not available at training time. In this paper, we propose for the first time a pipeline of pre-trained deep feature extractors and online learning-capable shallow classifiers-such as passive-aggressive classifiers, random forests, and support vector classifiers-for the personalisation of mobile activity recognition systems. Our suggested solution could enable efficient on-device online learning for personalisation, thereby contributing to users' privacy preservation. We report a series of experiments exploring the potential and the limitations of this approach to increase user-specific performance given only a limited number of training samples. Our results are encouraging and show (i) that a single additional data point per activity class (in a 25-class problem) from a new user can reduce recognition errors relatively by up to 20 %, and (ii) that with an increasing number of training samples our personalised models outperform comparable approaches in the literature, achieving accuracies of up to 91.4 %.
更多查看译文
关键词
Mobile Activity Recognition, Personalisation, Online Learning, Deep Learning, Shallow Classifiers
AI 理解论文
溯源树
样例
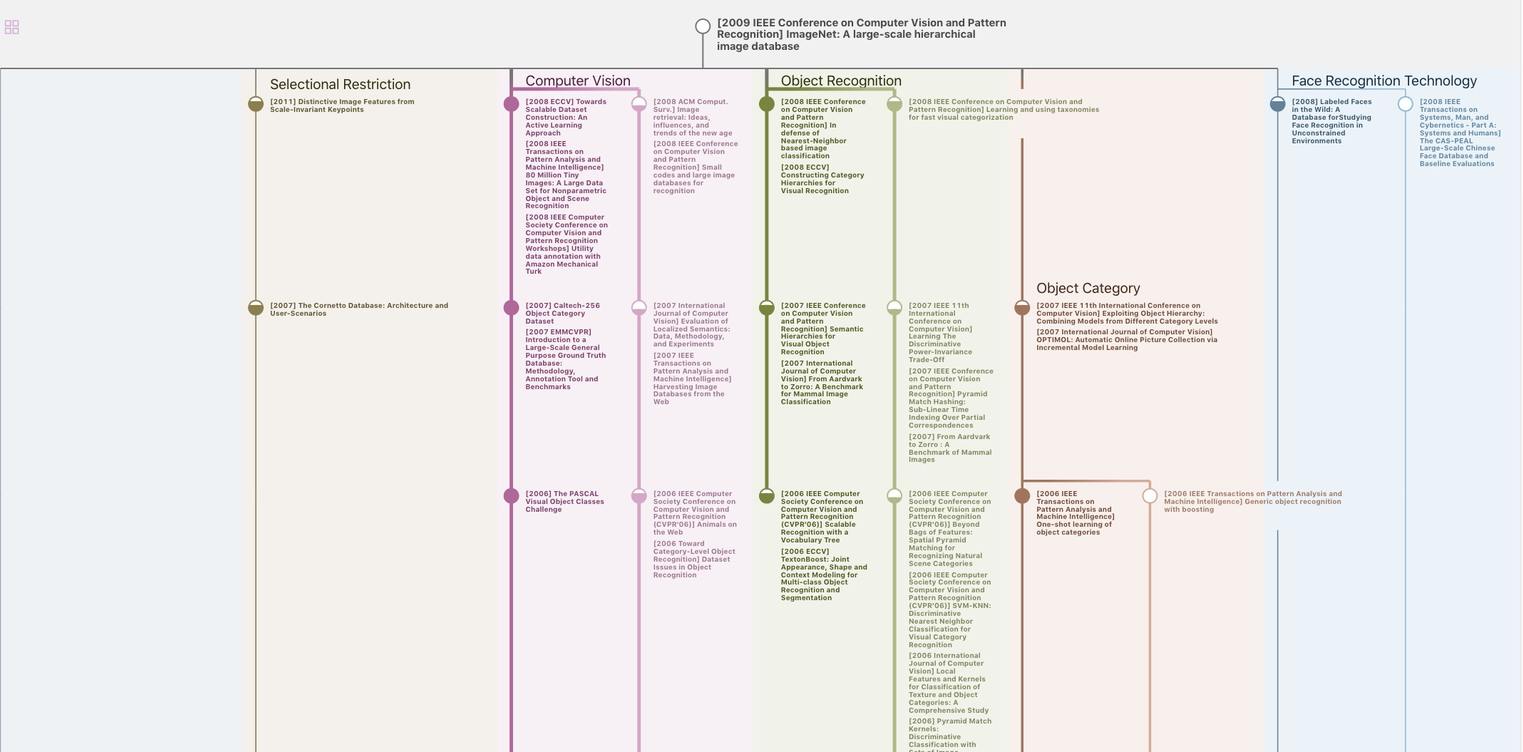
生成溯源树,研究论文发展脉络
Chat Paper
正在生成论文摘要