An encrypted traffic classification method based on contrastive learning.
ICCIP(2022)
摘要
Network traffic classification has become an important part of network management, which is conducive to realizing intelligent network operation and maintenance, improving network quality of service (QoS), and ensuring network security. With the rapid development of various applications and protocols, more and more encrypted traffic appears in the network. Due to the loss of semantic information after traffic encryption, poor content intelligibility, and difficulty in feature extraction, traditional detection methods are no longer applicable. Existing solutions mainly rely on the powerful feature self-learning ability of end-to-end deep neural networks to identify encrypted traffic. However, such methods are overly dependent on data size, and it has been experimentally proven that it is often difficult to achieve satisfactory results when validating across datasets. In order to solve this problem, this paper proposes an encrypted traffic identification method based on contrastive learning. First, the clustering method is used to expand the labeled data set. When the encrypted traffic features are difficult to extract, it is only necessary to learn the feature space to achieve discrimination.more suitable for encrypted traffic identification. When validating across datasets, only fine-tuning is required on a small amount of labeled data to achieve good recognition results. Compared with the end-to-end learning method, there is an improvement of about 5%. CCS CONCEPTS • Security and privacy • Network security • Security protocols
更多查看译文
AI 理解论文
溯源树
样例
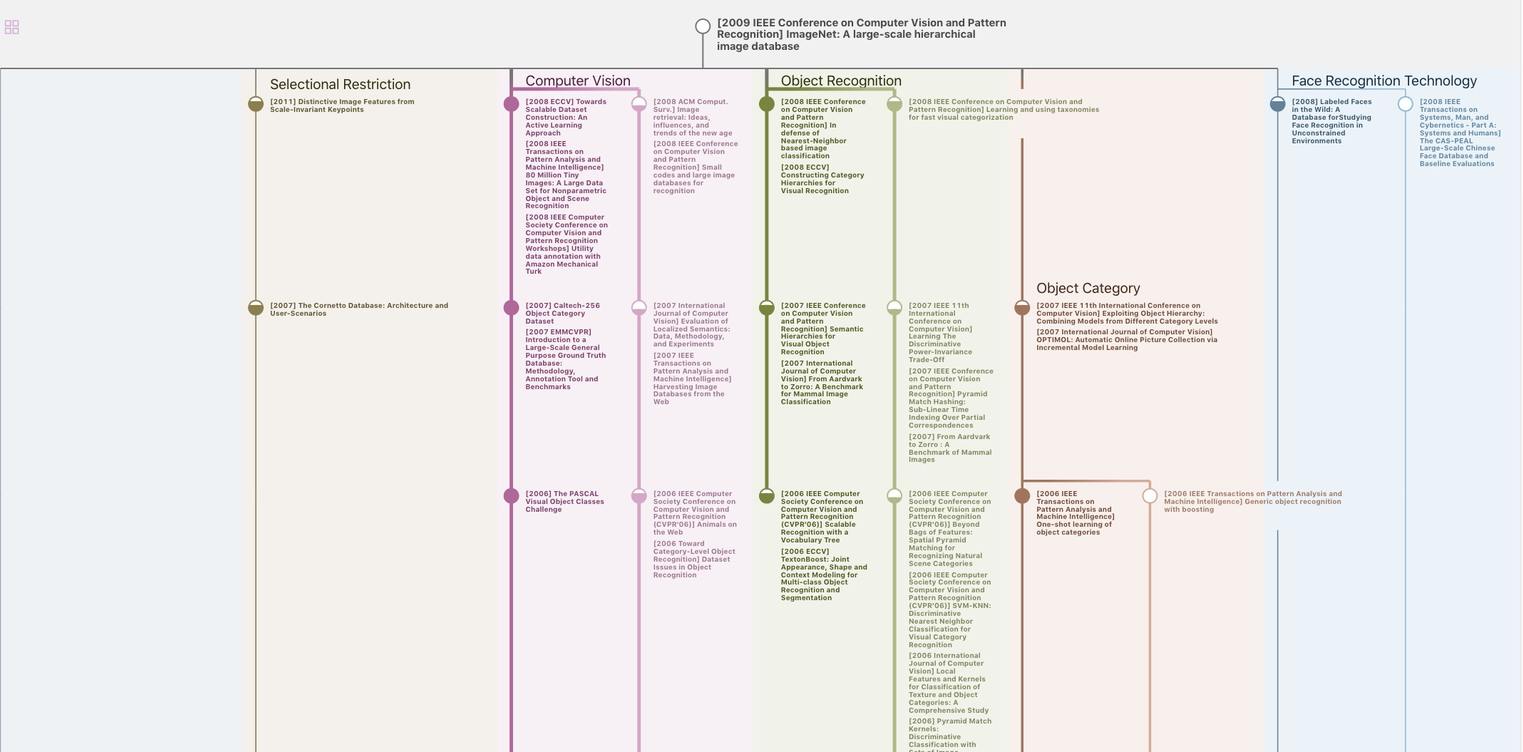
生成溯源树,研究论文发展脉络
Chat Paper
正在生成论文摘要