Phenotyping of Cervical Cancer Risk Groups via Generalized Low-Rank Models Using Medical Questionnaires.
NAIS(2022)
摘要
AbstractThe purpose of this study is to uncover cervical cancer (CC) risk phenotypes from self-reported lifestyle questionnaires and screening data. In general, computational phenotype discovery aims to find subgroups among individuals that share distinctive characteristics by analyzing electronic health records (EHR). This can benefit the understanding of a disease as well as uncover risk factors and provide possibilities for preventive action. The features in the women ($$n = 6359$$ n = 6359 ) by questionnaire features ($$p=29$$ p = 29 ) matrix with missing data are of different statistical data types (e.g., binary or ordinal data). We use so-called generalized low-rank models (GLRM) that can address this challenge via different statistical-data-type-dependent loss functions. We show that these models can uncover phenotypes related to cervical cancer risk factors from large-scale questionnaire data.
更多查看译文
关键词
cervical cancer risk groups,medical questionnaires,low-rank
AI 理解论文
溯源树
样例
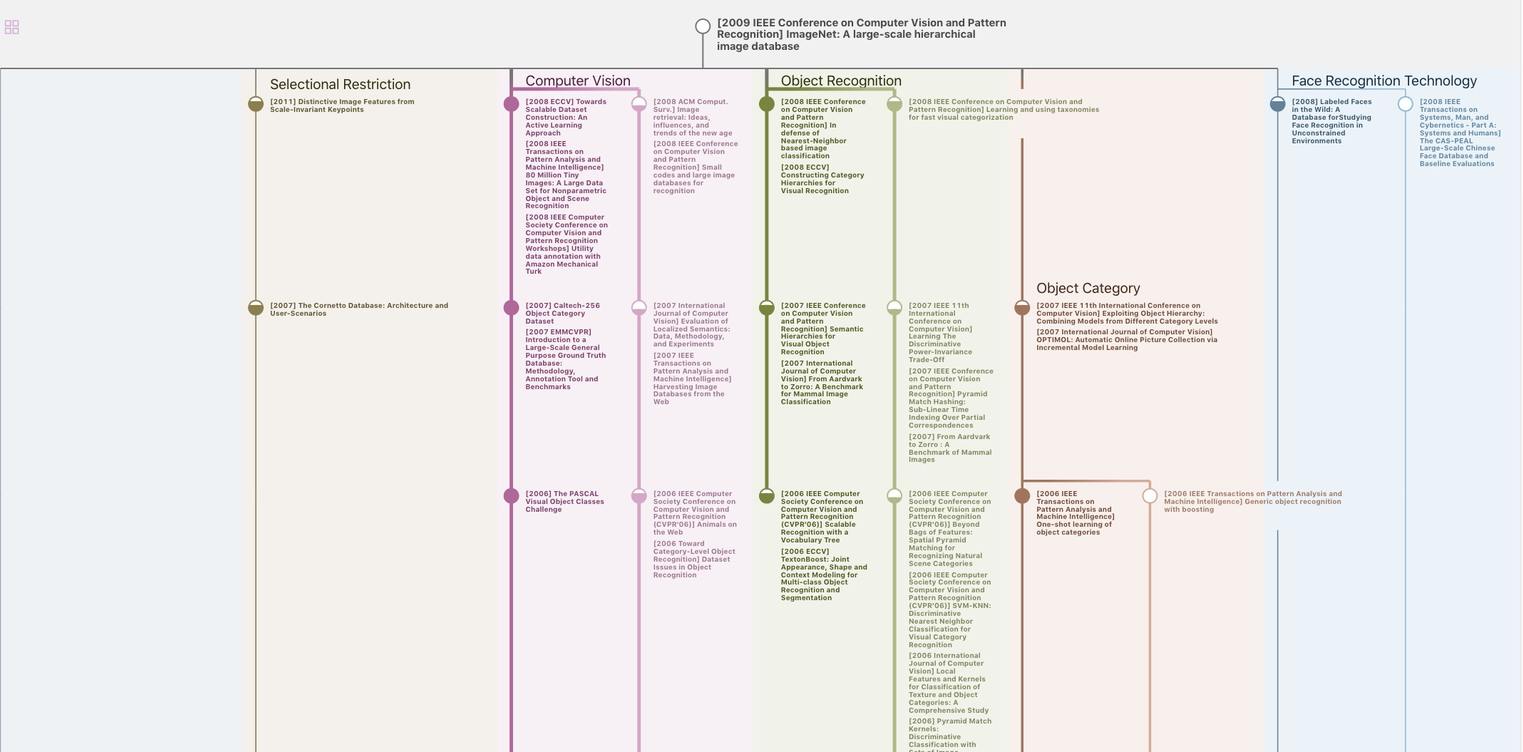
生成溯源树,研究论文发展脉络
Chat Paper
正在生成论文摘要